A 95% accurate EEG-connectome processor for a mental health monitoring system
JOURNAL OF SEMICONDUCTOR TECHNOLOGY AND SCIENCE(2016)
摘要
An electroencephalogram (EEG)-connectome processor to monitor and diagnose mental health is proposed. From 19-channel EEG signals, the proposed processor determines whether the mental state is healthy or unhealthy by extracting significant features from EEG signals and classifying them. Connectome approach is adopted for the best diagnosis accuracy, and synchronization likelihood (SL) is chosen as the connectome feature. Before computing SL, reconstruction optimizer (ReOpt) block compensates some parameters, resulting in improved accuracy. During SL calculation, a sparse matrix inscription (SMI) scheme is proposed to reduce the memory size to 1/24. From the calculated SL information, a small world feature extractor (SWFE) reduces the memory size to 1/29. Finally, using SLs or small word features, radial basis function (RBF) kernel-based support vector machine (SVM) diagnoses user's mental health condition. For RBF kernels, look-up-tables (LUTs) are used to replace the floating-point operations, decreasing the required operation by 54%. Consequently, The EEG-connectome processor improves the diagnosis accuracy from 89% to 95% in Alzheimer's disease case. The proposed processor occupies 3.8 mm2 and consumes 1.71 mW with 0.18 mu m CMOS technology.
更多查看译文
关键词
Connectome,EEG processor,brain disease,synchronization likelihood,wearable
AI 理解论文
溯源树
样例
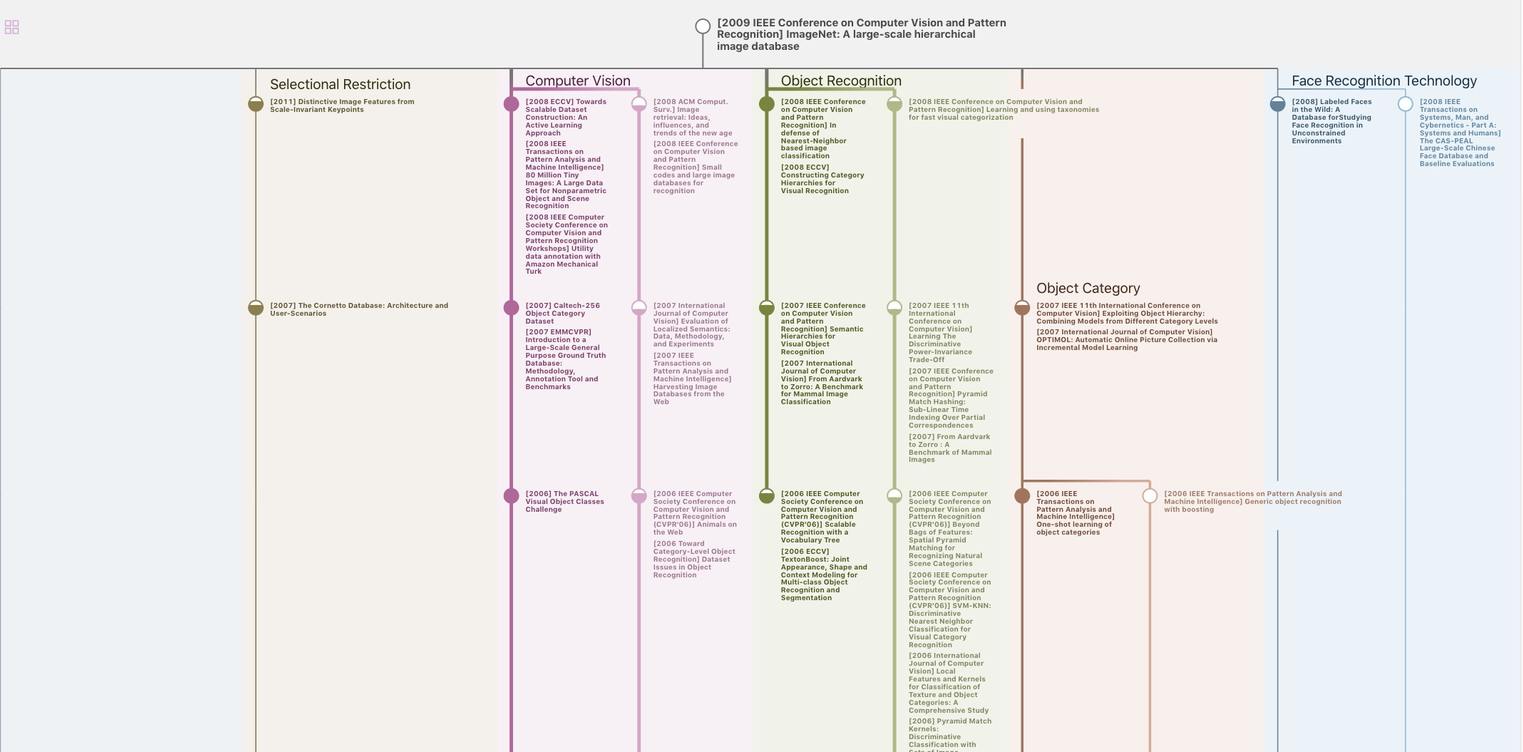
生成溯源树,研究论文发展脉络
Chat Paper
正在生成论文摘要