Nonparametric identification and maximum likelihood estimation for hidden Markov models
BIOMETRIKA(2016)
摘要
Nonparametric identification and maximum likelihood estimation for finite-state hidden Markov models are investigated. We obtain identification of the parameters as well as the order of the Markov chain if the transition probability matrices have full-rank and are ergodic, and if the state-dependent distributions are all distinct, but not necessarily linearly independent. Based on this identification result, we develop a nonparametric maximum likelihood estimation theory. First, we show that the asymptotic contrast, the Kullback-Leibler divergence of the hidden Markov model, also identifies the true parameter vector nonparametrically. Second, for classes of state-dependent densities which are arbitrary mixtures of a parametric family, we establish the consistency of the nonparametric maximum likelihood estimator. Here, identification of the mixing distributions need not be assumed. Numerical properties of the estimates and of nonparametric goodness of fit tests are investigated in a simulation study.
更多查看译文
关键词
Hidden Markov model,Latent state model,Nonparametric identification,Nonparametric maximum likelihood estimation
AI 理解论文
溯源树
样例
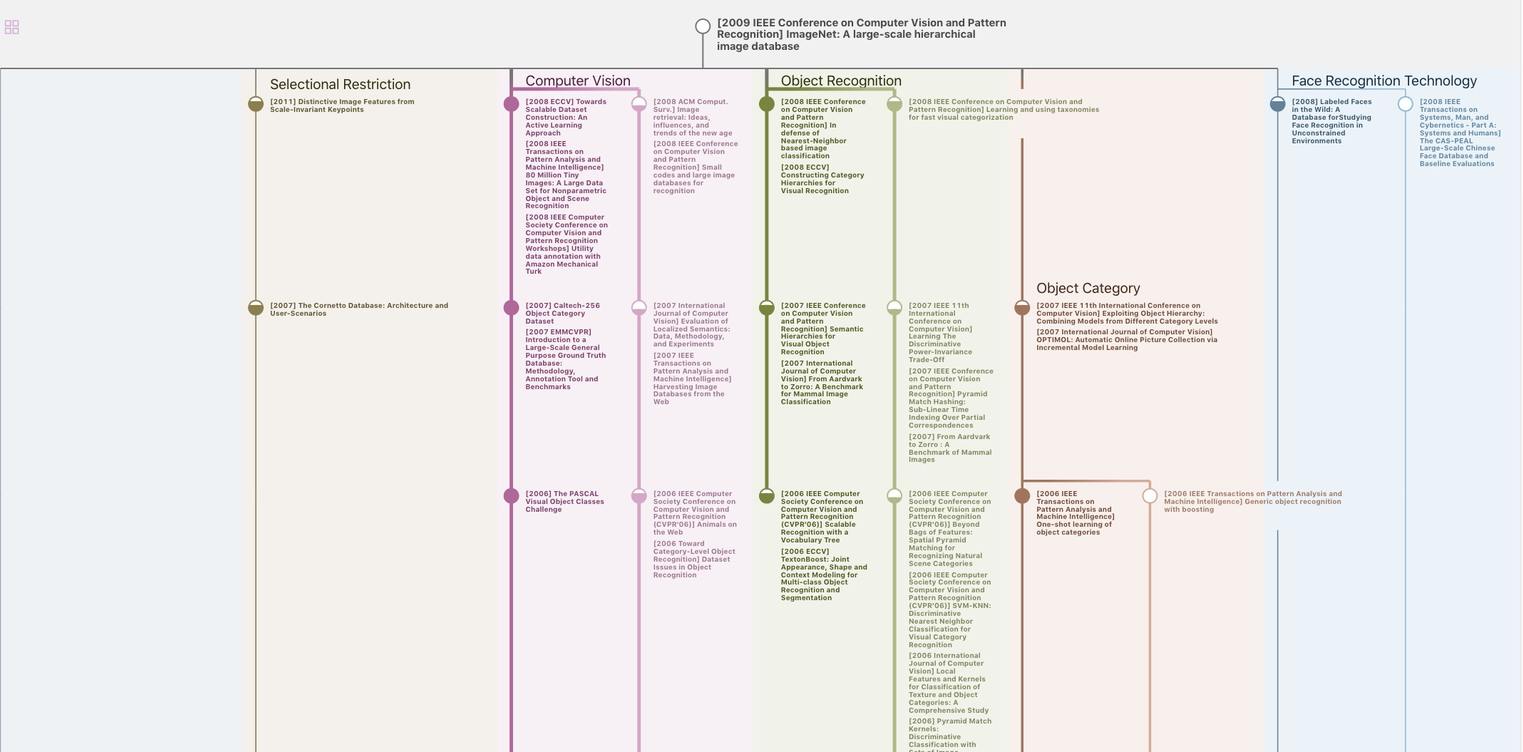
生成溯源树,研究论文发展脉络
Chat Paper
正在生成论文摘要