Scaling Semantic Frame Annotation
LAW@NAACL-HLT(2015)
摘要
Large-scale data resources needed for progress toward natural language understanding are not yet widely available and typically require considerable expense and expertise to create. This paper addresses the problem of developing scalable approaches to annotating semantic frames and explores the viability of crowdsourcing for the task of frame disambiguation. We present a novel supervised crowdsourcing paradigm that incorporates insights from human computation research designed to accommodate the relative complexity of the task, such as exemplars and real-time feedback. We show that non-experts can be trained to perform accurate frame disambiguation, and can even identify errors in gold data used as the training exemplars. Results demonstrate the efficacy of this paradigm for semantic annotation requiring an intermediate level of expertise. 1 The semantic bottleneck Behind every great success in speech and language lies a great corpus—or at least a very large one. Advances in speech recognition, machine translation and syntactic parsing can be traced to the availability of large-scale annotated resources (Wall Street Journal, Europarl and Penn Treebank, respectively) providing crucial supervised input to statistically learned models. Semantically annotated resources have been comparatively harder to come by: representing meaning poses myriad philosophical, theoretical and practical challenges, particularly for general purpose resources that can be applied to diverse domains. If these challenges can be addressed, however, semantic resources hold significant potential for fueling progress beyond shallow syntax and toward deeper language understanding. This paper explores the feasibility of developing scalable methodologies for semantic annotation, inspired by three strands of work. First, frame semantics, and its instantiation in the Berkeley FrameNet project (Fillmore and Baker, 2010), offers a principled approach to representing meaning. FrameNet is a lexicographic resource that captures syntactic and semantic generalizations that go beyond surface form and part of speech, famously including the relationships among words like buy, sell, purchase and price. These rich structural relations provide an attractive foundation for work in deeper natural language understanding and inference, as attested by the breadth of applications at the Workshop in Honor of Chuck Fillmore at ACL 2014 (Petruck and de Melo, 2014). But FrameNet was not designed to support scalable language technologies; indeed, it is perhaps a paradigm example of a hand-curated knowledge resource, one that has required significant expertise, training, time and expense to create and that remains under development. Second, the task of automatic semantic role labeling (ASRL) (Gildea and Jurafsky, 2002) serves as an applied counterpart to the ideas of frame semantics. Recent progress has demonstrated the viability of training automated models using frameannotated data (Das et al., 2013; Das et al., 2010; Johansson and Nugues, 2006). Results based on FrameNet data have been limited by its incomplete
更多查看译文
关键词
semantic frame annotation
AI 理解论文
溯源树
样例
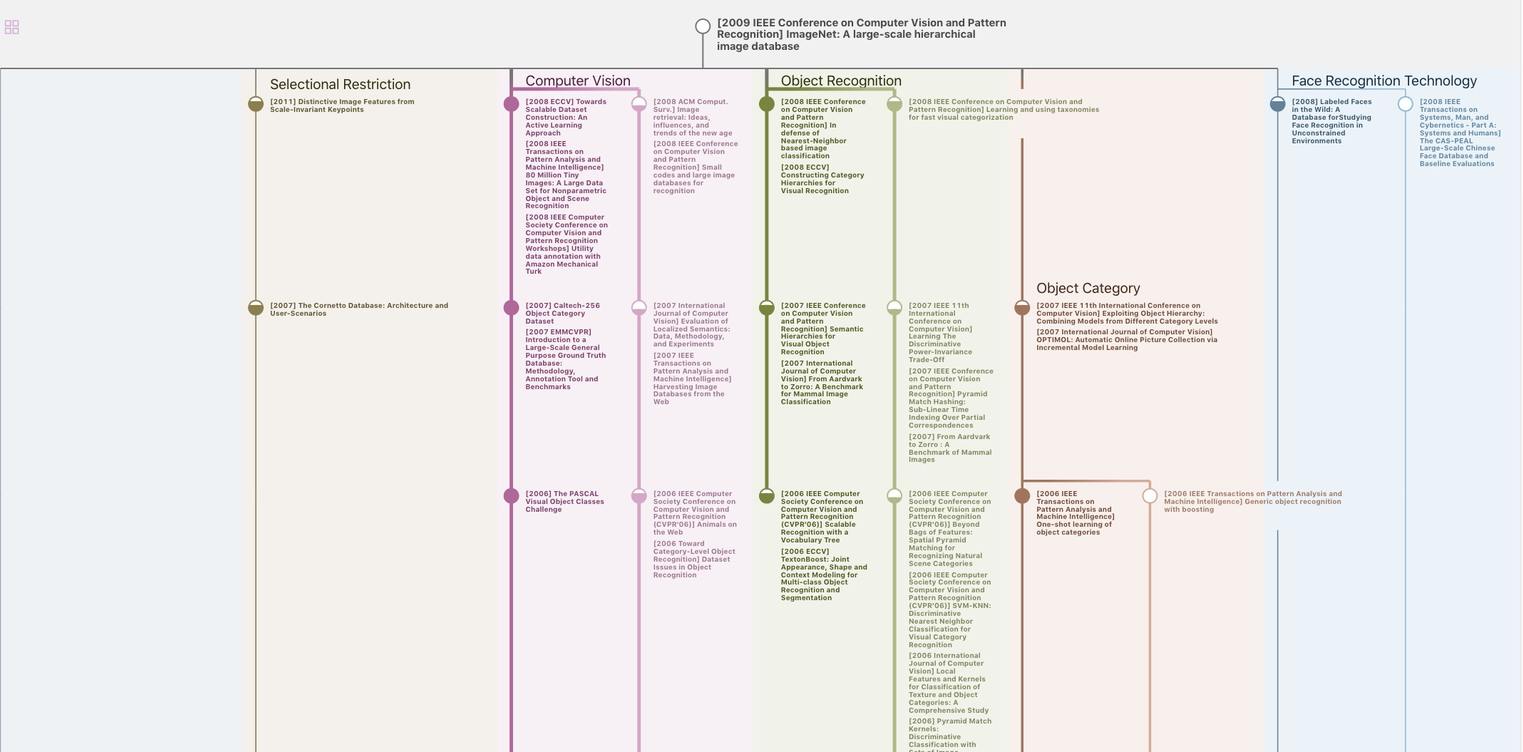
生成溯源树,研究论文发展脉络
Chat Paper
正在生成论文摘要