Optimal Shrinkage of Singular Values
IEEE Trans. Information Theory(2017)
摘要
We consider the recovery of low-rank matrices from noisy data by shrinkage of singular values, in which a single, univariate nonlinearity is applied to each of the empirical singular values. We adopt an asymptotic framework, in which the matrix size is much larger than the rank of the signal matrix to be recovered, and the signal-to-noise ratio of the low-rank piece stays constant. For a variety of loss functions, including Mean Square Error (MSE) - (square Frobenius norm), the nuclear norm loss and the operator norm loss, we show that in this framework there is a well-defined asymptotic loss that we evaluate precisely in each case. In fact, each of the loss functions we study admits a unique admissible shrinkage nonlinearity dominating all other nonlinearities. We provide a general method for evaluating these optimal nonlinearities, and demonstrate our framework by working out simple, explicit formulas for the optimal nonlinearities in the Frobenius, nuclear and operator norm cases. For example, for a square low-rank $n$ -by- $n$ matrix observed in white noise with level $\\sigma $ , the optimal nonlinearity for MSE loss simply shrinks each data singular value $y$ to $\\sqrt {y^{2}-4n\\sigma ^{2}}$ (or to 0 if $y<2\\sqrt {n}\\sigma $ ). This optimal nonlinearity guarantees an asymptotic MSE of $2nr\\sigma ^{2}$ , which compares favorably with optimally tuned hard thresholding and optimally tuned soft thresholding, providing guarantees of $3nr\\sigma ^{2}$ and $6nr\\sigma ^{2}$ , respectively. Our general method also allows one to evaluate optimal shrinkers numerically to arbitrary precision. As an example, we compute optimal shrinkers for the Schatten- $p$ norm loss, for any $p>0$ .
更多查看译文
关键词
Information theory,Signal to noise ratio,Noise reduction,Estimation,Covariance matrices,Noise level,Noise measurement
AI 理解论文
溯源树
样例
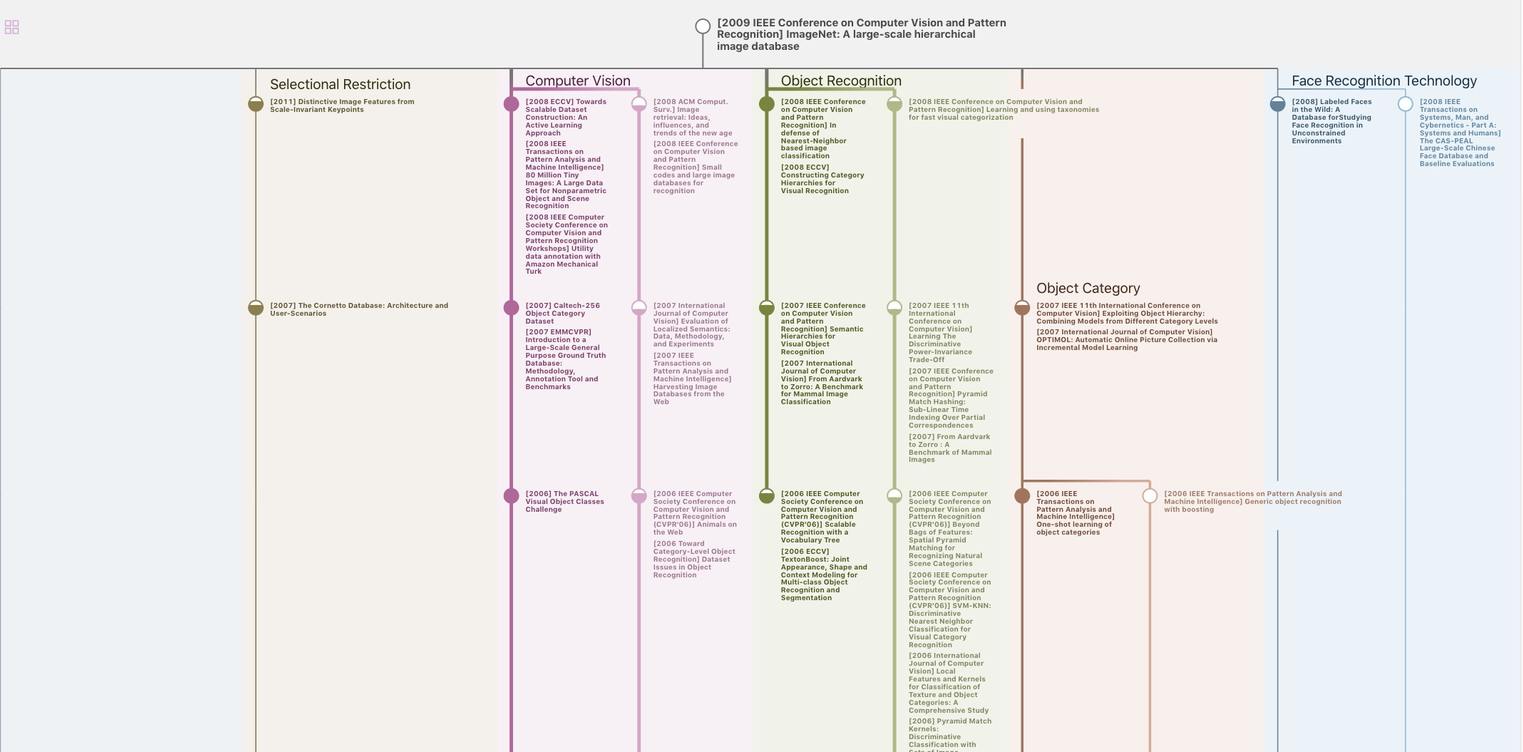
生成溯源树,研究论文发展脉络
Chat Paper
正在生成论文摘要