Spatio-temporal graphical modeling with innovations based on multi-scale diffusion kernel
Spatial Statistics(2014)
摘要
A random field of interest is observed on an undirected spatial graph over time, thereby providing a time series of dependent random fields. We propose a general modeling procedure which has the potential to explicitly quantify intrinsic and extrinsic fluctuations of such dynamical system. We adopt a paradigm in which the intrinsic fluctuations correspond to a process of latent diffusion on the graph arising from stochastic interactions within the system, whereas the extrinsic fluctuations correspond to a temporal drift reflecting the effects of the environment on the system. We start with a spatio-temporal diffusion process which gives rise to the latent spatial process. This makes a bridge with the conventional Wold representation, for which the latent process represents the innovation process, and beyond that with the stochastic differential equation associated to the Fokker–Planck dynamic. The innovation process is modeled by a Gaussian distribution whose covariance matrix is defined by a multi-scale diffusion kernel. This model leads to a multi-scale representation of the spatio-temporal process. We propose a statistical procedure to estimate the multi-scale structure and the model parameters in the case of the vector autoregressive model with drift. Modeling and estimation tasks are illustrated on simulated and real biological data.
更多查看译文
关键词
Spatio-temporal graphical model,Spatial statistic modeling,Multi-scale heat diffusion kernel,Graph Laplacian,Intrinsic and extrinsic stochastic fluctuations,Multi-scale decomposition
AI 理解论文
溯源树
样例
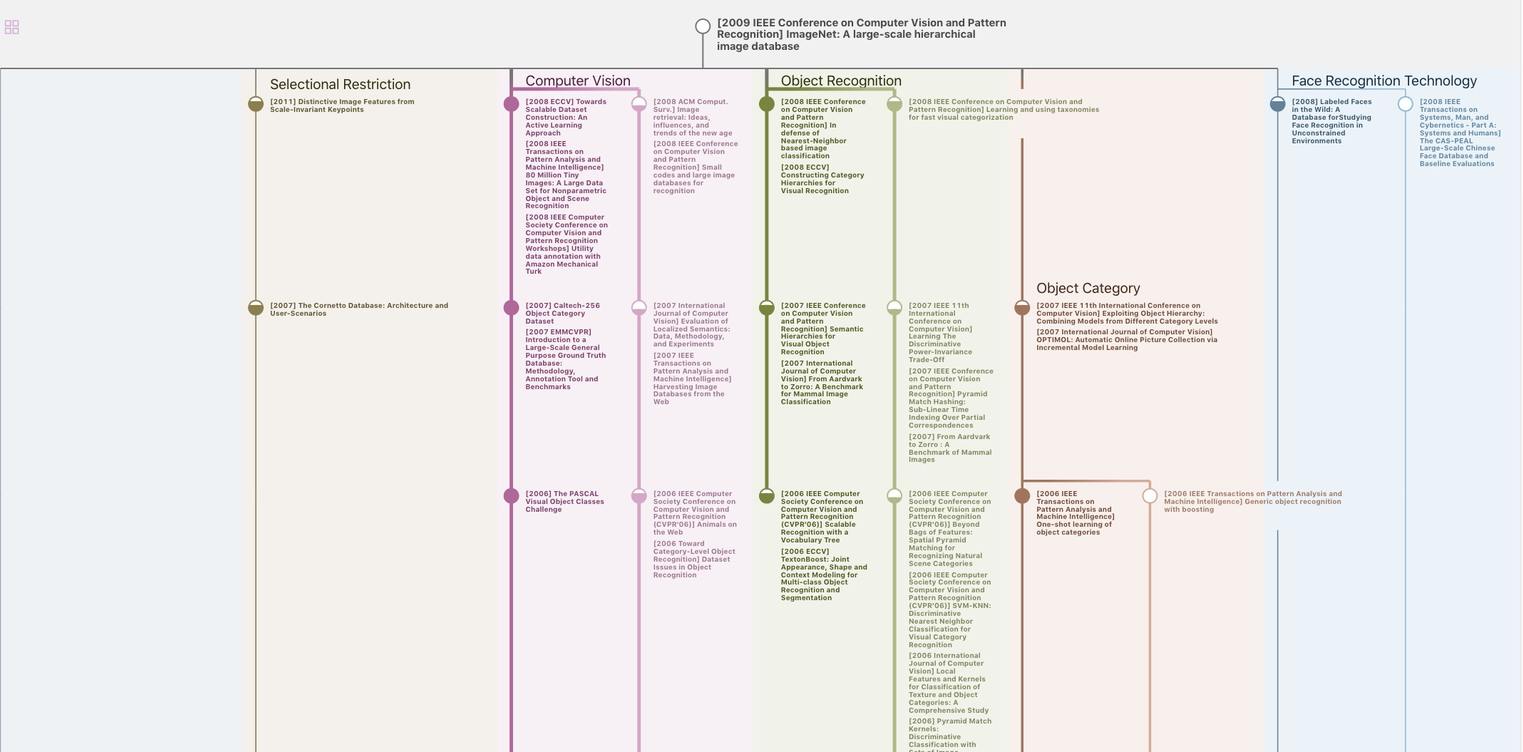
生成溯源树,研究论文发展脉络
Chat Paper
正在生成论文摘要