Active Learning with an ERM Oracle
mag(2013)
摘要
One technique that has proved theoretically profitable is to maintain a candidate set of hypotheses (sometimes called a version space), and to query the label of a point if and only if there is disagreement within this set about how to label the point. The criteria for membership in this candidate set need to be carefully defined so that the optimal hypothesis h∗ is always included, but otherwise this set can be quickly whittled down as more labels are queried. This technique is rather straightforward in the realizable setting [3], and it can be extended to the agnostic setting with the use of confidence bounds and hard example constraints [1, 4]. One of the algorithms in [2] uses large importance weights to essentially achieve the same effect.
更多查看译文
AI 理解论文
溯源树
样例
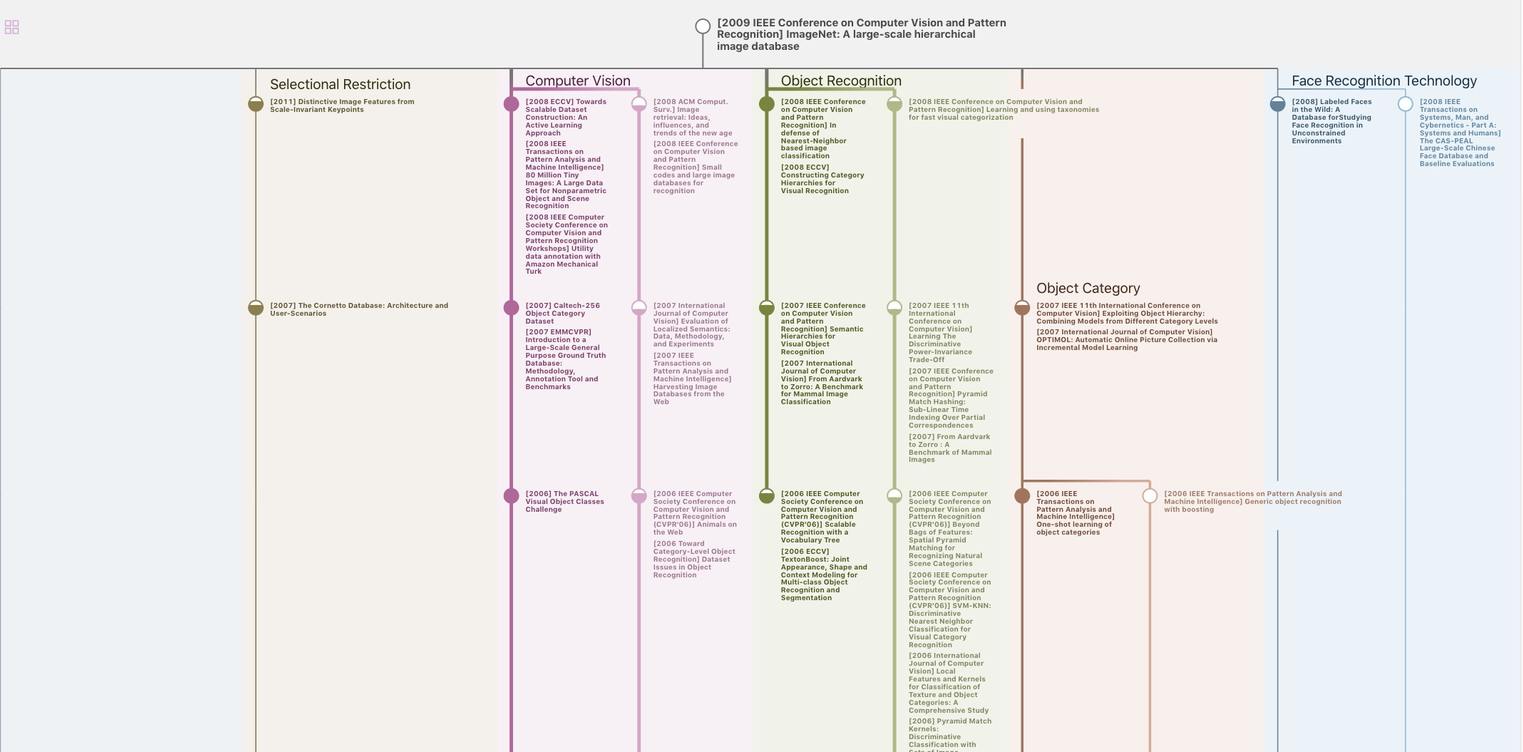
生成溯源树,研究论文发展脉络
Chat Paper
正在生成论文摘要