Ensemble Learning Soft Sensor Method of Endpoint Carbon Content and Temperature of BOF Based on GCN Embedding Supervised Ensemble Clustering
Measurement science & technology(2024)
摘要
The endpoint control of Basic Oxygen Furnace (BOF) steelmaking depends on the prediction of the endpoint carbon content and temperature. However, predicting these variables is challenging because of the numerous working conditions in the industrial field and the volatility of the sensor data collected during BOF steelmaking. The accuracy of prediction models in ensemble learning depends significantly on the initial distribution of data. However, the complex nature of BOF steelmaking data makes it challenging to generate diverse subsets, which ultimately affects the accuracy of predictions. This paper presents a new approach called Graph Convolutional Network Node Embedding Supervised Ensemble Clustering (GESupEC) for soft sensor modelling in ensemble learning to tackle these issues. GESupEC utilizes a similarity graph derived from a co-association matrix and employs graph convolutional networks to extract structural information among nodes. By optimising the clustering loss within the network, GESupEC learns compact node representations that are useful for the clustering task. Furthermore, it generates a reconstruction matrix based on the similarity of node embeddings. This matrix helps with the extraction of a suitable subset of data for BOF steelmaking through matrix decomposition. After that, the GBDT regression sub-model is established based on the data subset. An ensemble strategy called GRAWA is proposed, which assigns weights based on the grey relation similarity between test samples and different data subsets. This weighted average strategy aims to enhance the accuracy of carbon content and temperature predictions. When tested with actual BOF steelmaking generation process data, the prediction accuracy of carbon content reached 88.6% within the error range of ±0.02%, and the prediction accuracy of temperature reached 92.6% within the error range of ±10℃.
更多查看译文
关键词
soft sensor,BOF steelmaking endpoint,ensemble learning,ensemble clustering,graph convolutional networks
AI 理解论文
溯源树
样例
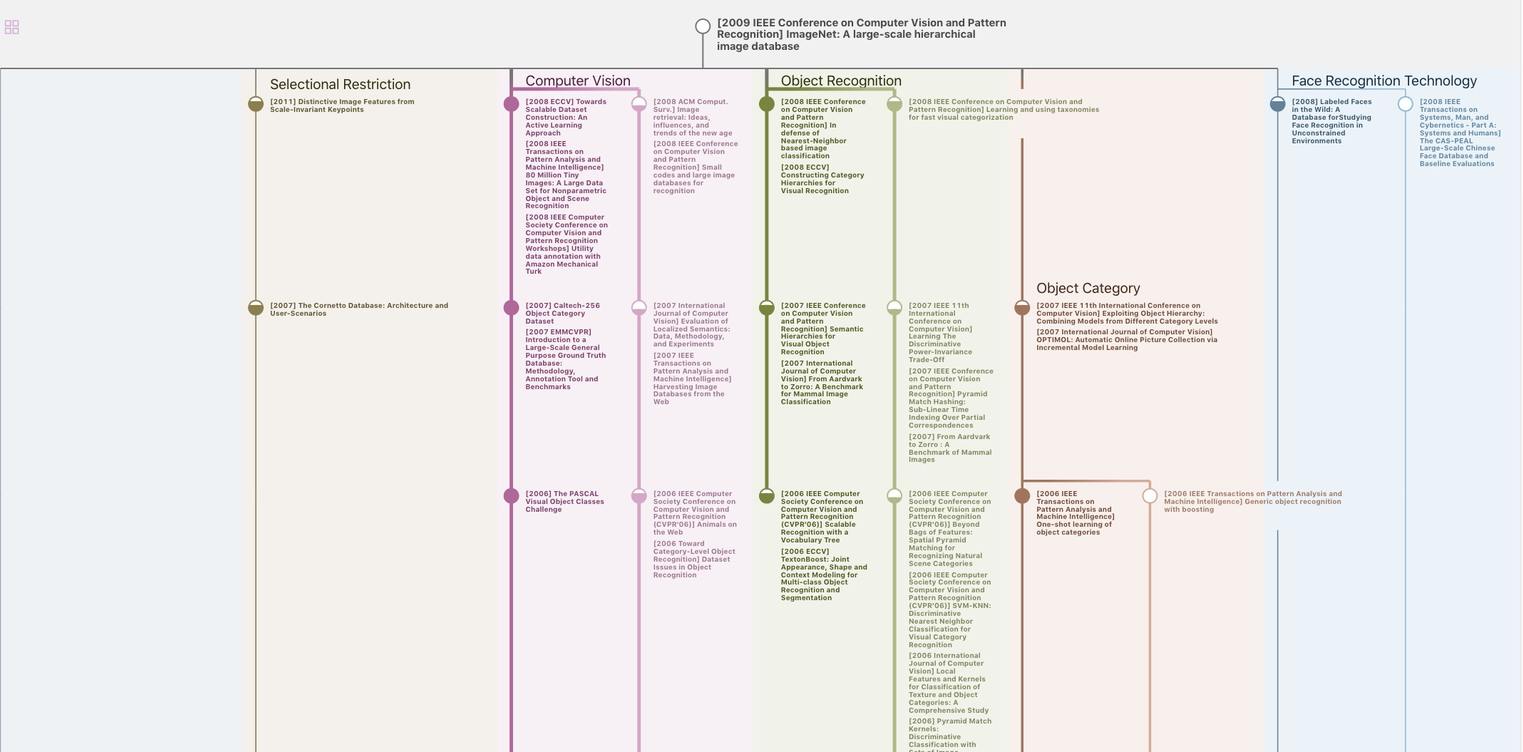
生成溯源树,研究论文发展脉络
Chat Paper
正在生成论文摘要