Kernel-Based Electric Vehicle Charging Load Modeling With Improved Latin Hypercube Sampling
2015 IEEE POWER & ENERGY SOCIETY GENERAL MEETING(2015)
摘要
Daily trip distance and end time of the last trip are two essential variables for home based electric vehicle (HBEV) charging load model. A non-parametric Gaussian kernel density estimation method is proposed to build the probability density distributions of these two variables. This method can improve the precision and adaptability of the distributions compared with parametric estimation. A Latin hypercube sampling technique with cubic spline interpolation is presented to generate random samples of the two variables. This technique is much more efficient in computation than Monte Carlo simulation. Simulation results using three different charging modes demonstrate that a time-of-use electricity price policy can guide consumers to charge at valley load time, and thus can change the shape of HBEV load charging curves.
更多查看译文
关键词
Electric vehicle,charging load,Gaussian kernel density,Latin Hypercube sampling,cubic spline interpolation
AI 理解论文
溯源树
样例
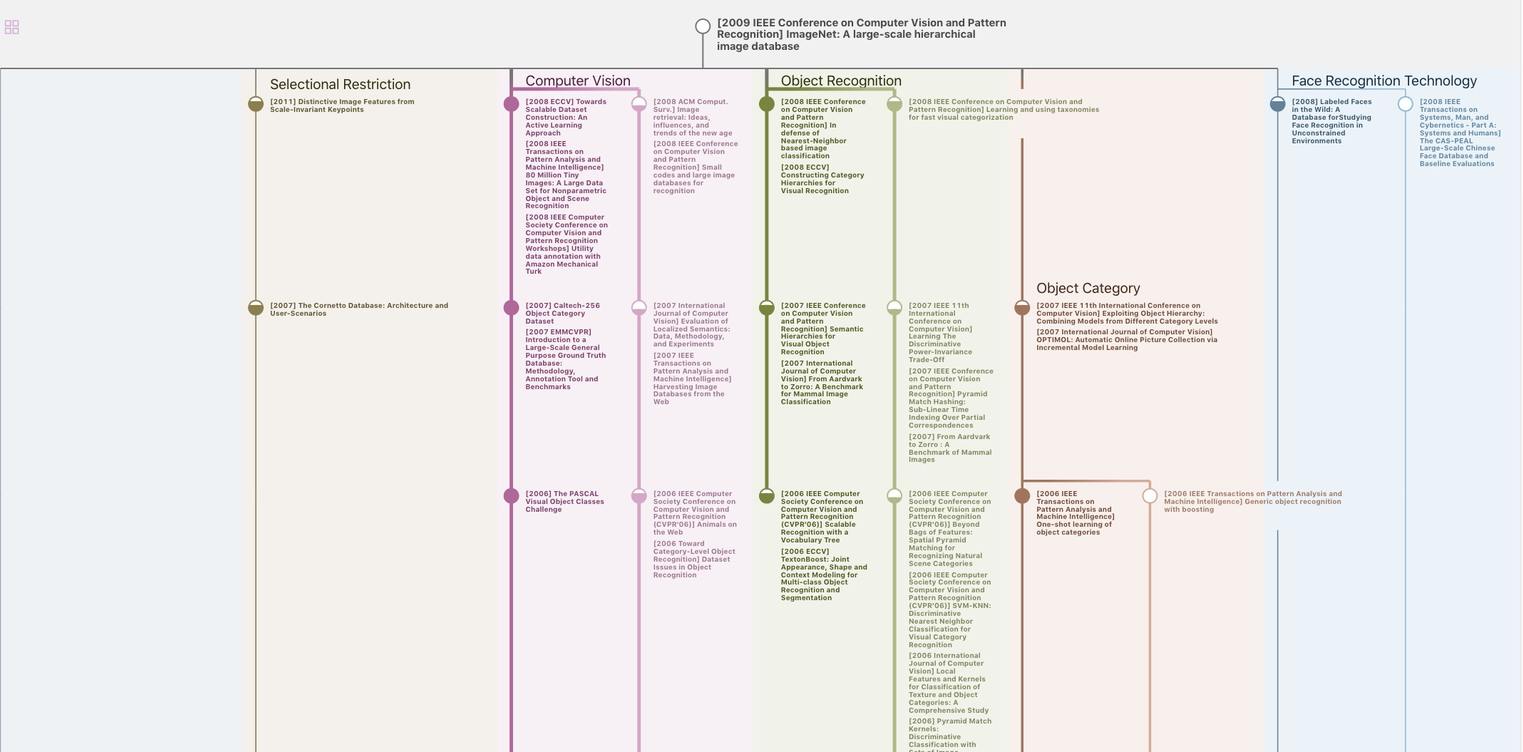
生成溯源树,研究论文发展脉络
Chat Paper
正在生成论文摘要