Traffic Flow Decomposition And Prediction Based On Robust Principal Component Analysis
2015 IEEE 18TH INTERNATIONAL CONFERENCE ON INTELLIGENT TRANSPORTATION SYSTEMS(2015)
摘要
Research on traffic data analysis is becoming more available and important. One of the key challenges is how to accurately decompose the high-dimensional, noisy observation traffic flow matrix into sub-matrices that correspond to different classes of traffic flow which builds a foundation for traffic flow prediction, abnormal data detection and missing data imputation. While in traditional research, Principal Component Analysis (PCA) is usually used for traffic matrix analysis. However, the traffic matrix is usually corrupted by large volume anomalies, the resulting principal components will be significantly skewed from those in the anomaly-free case. In this paper, we introduce the Robust Principal Component Analysis (robust PCA) for decomposition. It can mine more accurate and robust underlining temporal and spatial characteristics of traffic flow with all kinds of fluctuations. We performed a comparative experimental analysis based on robust PCA with PCA-based method on a real-life dataset and got better decomposition performance. In the real-life dataset, results show that through robust PCA most of the large volume anomalies are short-lived and well isolated in the residual traffic matrix while PCA failed. In traffic flow prediction experiments based on decomposition, it shows that the result based on robust PCA outperforms the PCA and simple average. It provide adequate evidence that robust PCA is more appropriate for traffic flow matrix analysis. Robust PCA shows promising abilities in improving the accuracy and reliability of traffic flow analysis.
更多查看译文
关键词
traffic flow decomposition,traffic flow prediction,robust principal component analysis,traffic data analysis,noisy observation traffic flow matrix,submatrices,abnormal data detection,missing data imputation,traffic matrix analysis,volume anomaly,anomaly-free case,robust PCA,residual traffic matrix,traffic flow matrix analysis,traffic flow analysis reliability
AI 理解论文
溯源树
样例
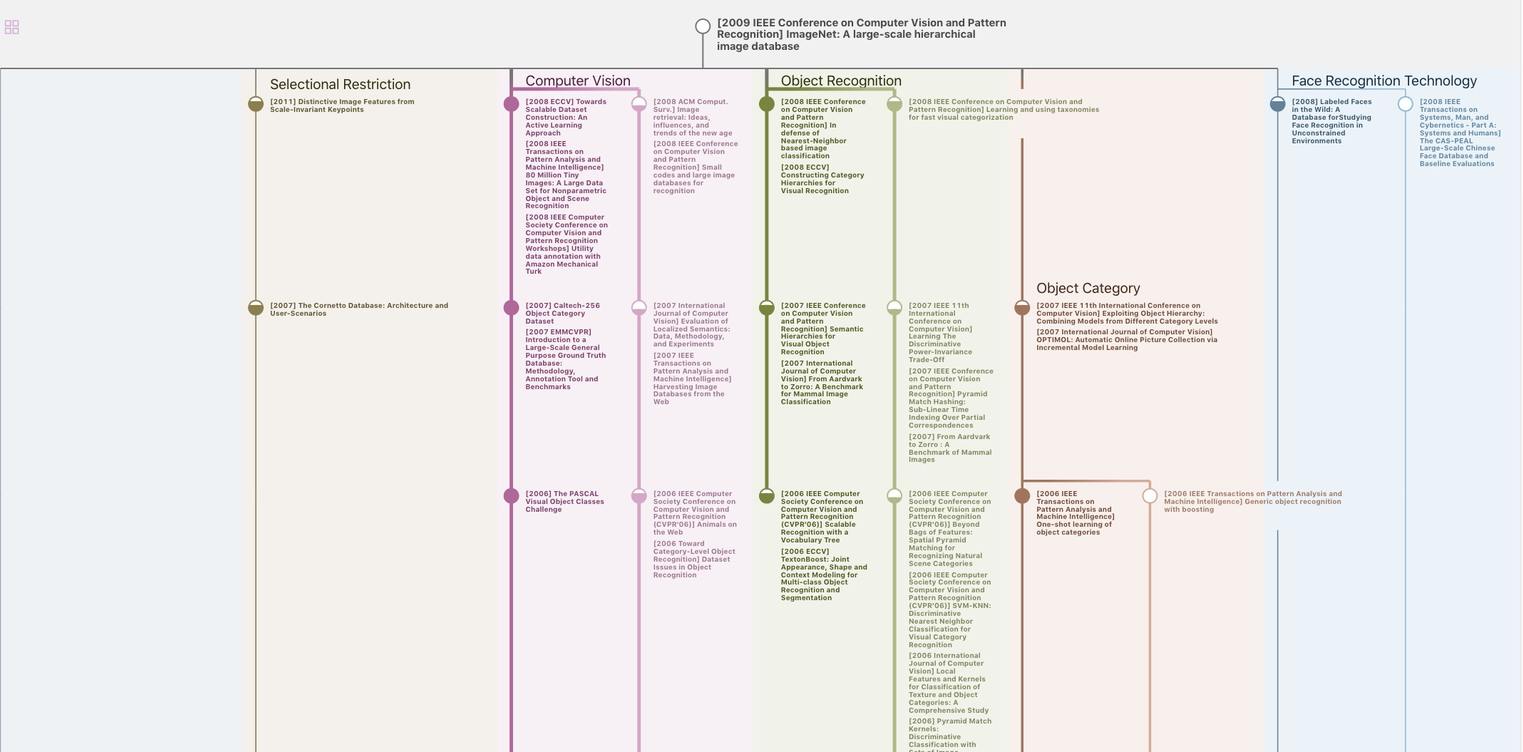
生成溯源树,研究论文发展脉络
Chat Paper
正在生成论文摘要