Multi-Task Learning for Improved Discriminative Training in SMT
WMT@ACL(2013)
摘要
Multi-task learning has been shown to be effective in various applications, including discriminative SMT. We present an experimental evaluation of the question whether multi-task learning depends on a “natural” division of data into tasks that balance shared and individual knowledge, or whether its inherent regularization makes multi-task learning a broadly applicable remedy against overfitting. To investigate this question, we compare “natural” tasks defined as sections of the International Patent Classification versus “random” tasks defined as random shards in the context of patent SMT. We find that both versions of multi-task learning improve equally well over independent and pooled baselines, and gain nearly 2 BLEU points over standard MERT tuning.
更多查看译文
关键词
improved discriminative training,smt,learning,multi-task
AI 理解论文
溯源树
样例
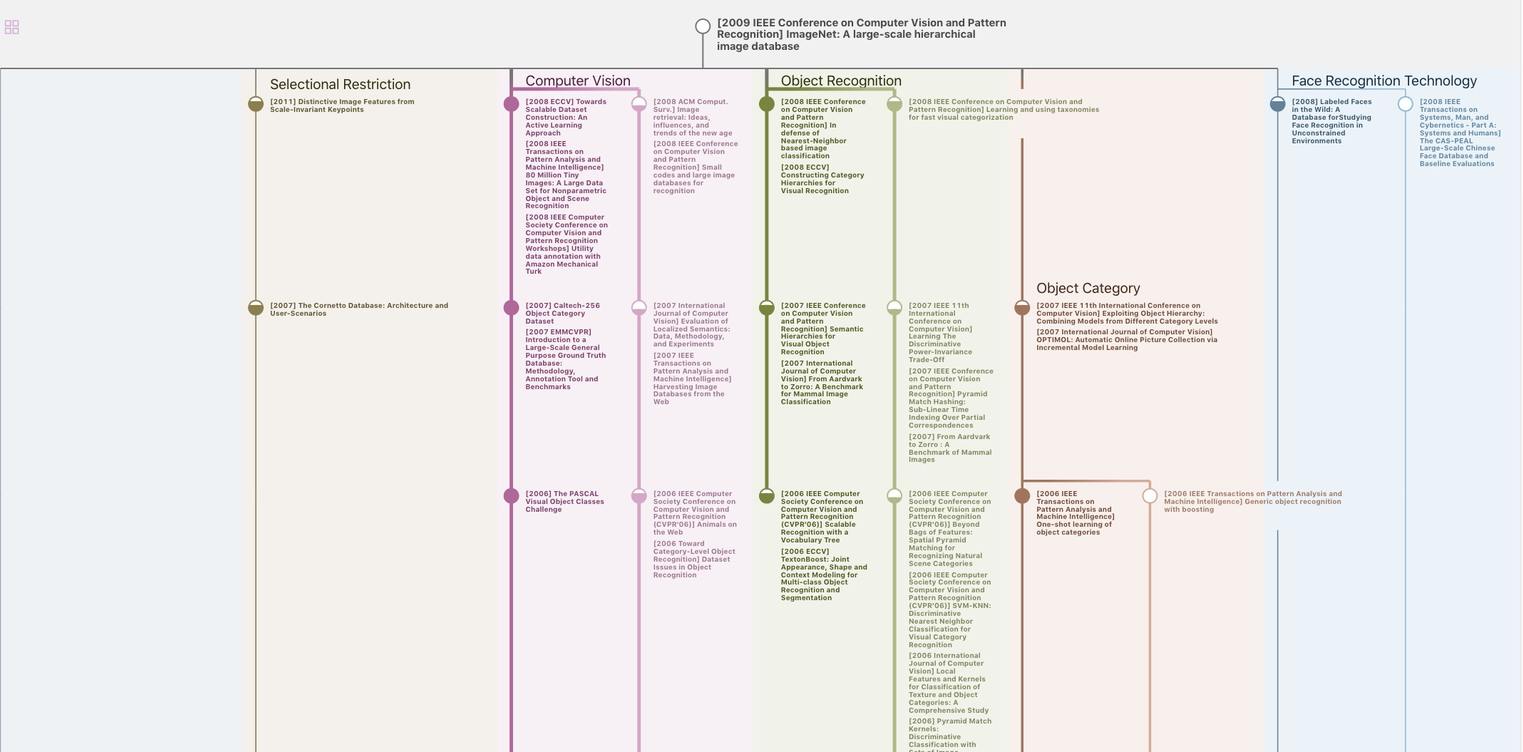
生成溯源树,研究论文发展脉络
Chat Paper
正在生成论文摘要