Stochastic feature selection with distributed feature spacing for hyperspectral data
WHISPERS(2010)
摘要
Feature subset selection is a well studied problem in machine learning. One short-coming of many methods is the selection of highly correlated features; a characteristic of hyperspec-tral data. A novel stochastic feature selection method with three major components is presented. First, we present an optimized feature selection method that maximizes a heuristic using a simulated annealing search which increases the chance of avoiding locally optimum solutions. Second, we exploit local cross correlation pair-wise amongst classes of interest to select suitable features for class discrimination. Third, we adopt the concept of distributed spacing from the multi-objective optimization community to distribute features across the spectrum in order to select less correlated features. The classification performance of our semi-embedded feature selection and classification method is demonstrated on a 12-class textile hyperspectral classification problem under several noise realizations. These results are compared with a variety of feature selection methods that cover a broad range of approaches.
更多查看译文
关键词
cross correlation,data analysis,simulated annealing,hyperspectral imaging,correlation,multi objective optimization,dimensionality reduction,noise,accuracy,hyperspectral,spectrum,machine learning,feature space,learning artificial intelligence,feature selection,multiobjective optimization,feature extraction,imaging
AI 理解论文
溯源树
样例
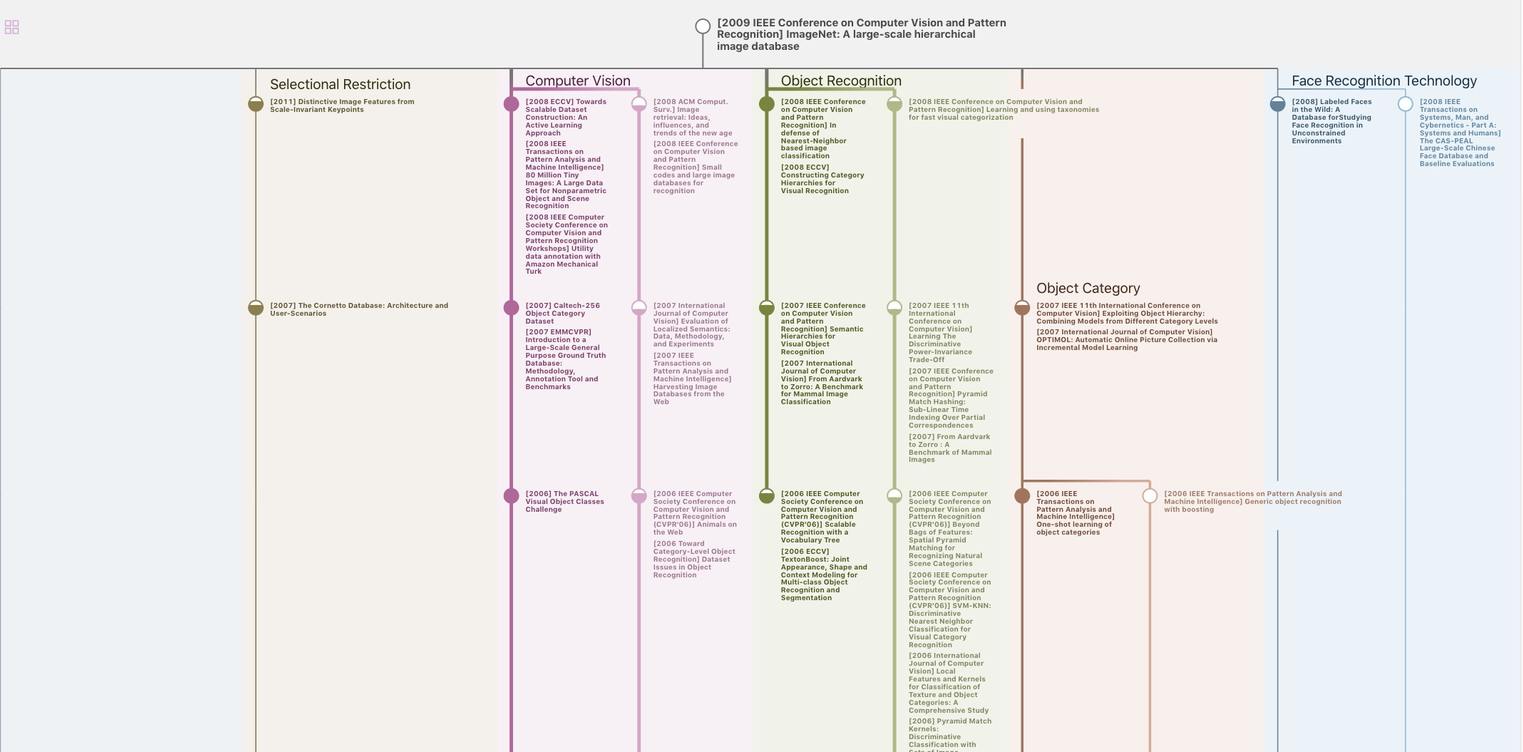
生成溯源树,研究论文发展脉络
Chat Paper
正在生成论文摘要