Using kernel correlation filters and class redundant feature analysis (CFA) with support vector machines on face recognition grand challenge (FRGC) phase-2 data for enhanced holistic face recognition
BIOMETRIC TECHNOLOGY FOR HUMAN IDENTIFICATION III(2006)
摘要
The Face Recognition Grand Challenge (FRGC) dataset is one of the most challenging datasets in the face recognition community, in this dataset we focus on the hardest experiment under the harsh un-controlled conditions. In this paper we compare how other popular face recognition algorithms like Direct Linear Discriminant Analysis (D-LDA) and Gram-Schmidt LDA methods compare to traditional eigenfaces, and fisherfaces. However, we also show that all these linear subspace methods can not discriminate faces well due to large nonlinear distortions in the face images. Thus we present our proposed Class dependence Feature Analysis (CFA) method which we demonstrate to produce superior performance compared to other methods by representing nonlinear features well. We perform this by extending the traditional CFA framework to use Kernel Methods and propose a proper choice of kernel parameters which improves the overall face recognition performance is significantly over the competing face recognition algorithms. We present results of this proposed approach on a large scale database from the Face Recognition Grand Challenge (FRGC)v2 which contains over 36,000 images focusing on Experiment 4 which poses the harshest scenario containing images captured under un-controlled indoor and outdoor conditions yielding significant illumination variations.
更多查看译文
关键词
databases,algorithms
AI 理解论文
溯源树
样例
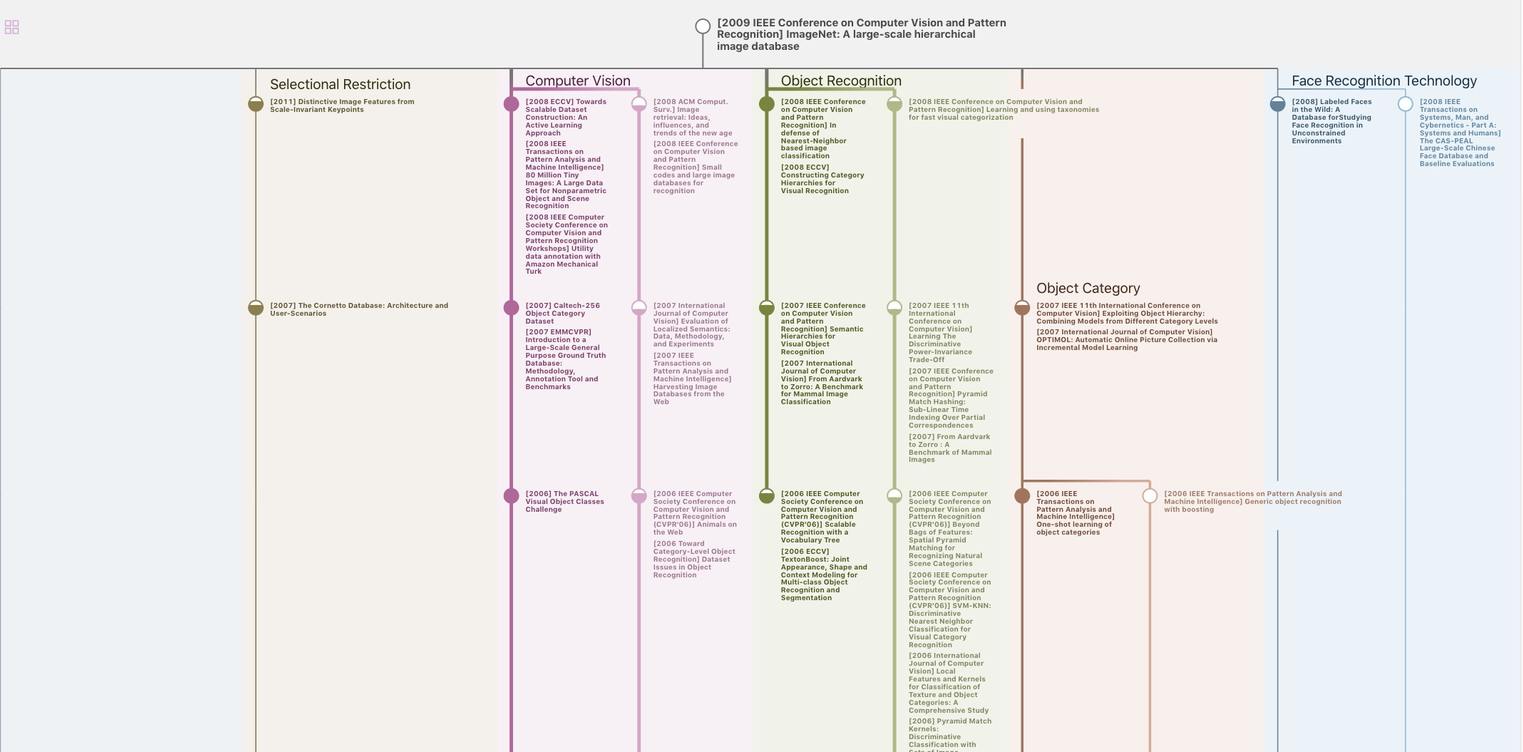
生成溯源树,研究论文发展脉络
Chat Paper
正在生成论文摘要