Physical activity recognition using inertial wearable sensors — A review of supervised classification algorithms
2015 International Conference on Advances in Biomedical Engineering (ICABME)(2015)
摘要
The goal of this paper is to compare the performances of various supervised algorithms in classifying physical daily living activities. Six healthy subjects were asked to perform twelve static and dynamic activities such as walking, running, sitting down, standing-up, lying, climbing stairs, etc. Three triaxial accelerometers are used to measure the human movements resulting from each activity. Seven supervised classification techniques are used: K-Nearest Neighbors (K-NN), Support Vector Machine (SVM), Random Forest (RF), Multi-Layer Perceptron (MLP), Classification And Regression Tree (CART), Naive Bayes (NB), and Gaussian Mixture Model (GMM). These methods are compared in terms of correct classification rate (Accuracy), Recall, Precision, F-measure and execution time. The 10-fold cross validation is used as a validation procedure. The obtained results show that K-NN gives the best results with 96.26 % of correct classification rate.
更多查看译文
关键词
Human activity recognition,Supervised classifiers,Inertial sensors,Acceleration data
AI 理解论文
溯源树
样例
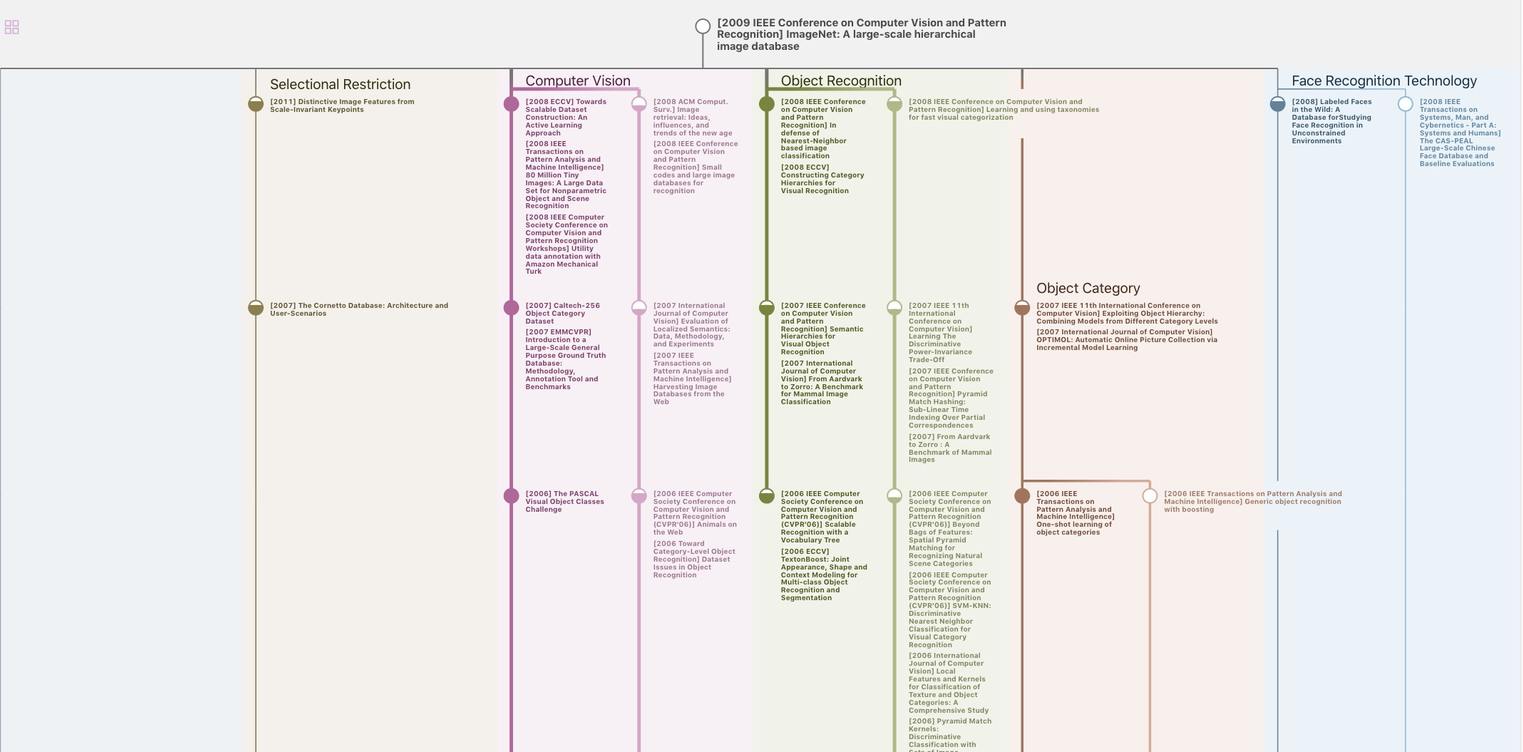
生成溯源树,研究论文发展脉络
Chat Paper
正在生成论文摘要