Motion Planning Of Uncertain Fully-Actuated Dynamical Systems-A Forward Dynamics Formulation
PROCEEDINGS OF THE ASME INTERNATIONAL DESIGN ENGINEERING TECHNICAL CONFERENCES AND COMPUTERS AND INFORMATION IN ENGINEERING CONFERENCE, 2011, VOL 8(2012)
摘要
This work presents a novel nonlinear programming based motion planning framework that treats uncertain fully-actuated dynamical systems described by ordinary differential equations. Uncertainty in multibody dynamical systems comes from various sources, such as: system parameters, initial conditions, sensor and actuator noise, and external forcing. Treatment of uncertainty in design is of paramount practical importance because all real-life systems are affected by it; ignoring uncertainty during design may lead to poor robustness and suboptimal performance. System uncertainties are modeled using Generalized Polynomial Chaos and are solved quantitatively using a least-square collocation method. The computational efficiency of this approach enables the inclusion of uncertainty statistics in the nonlinear programming optimization process. As such, new design questions related to uncertain dynamical systems can now be answered through the new framework.Specifically, this work presents the new framework through a forward dynamics formulation where deterministic actuator inputs are prescribed and uncertain state trajectories are quantified. The benefits of the ability to quantify the resulting state uncertainty are illustrated in an effort optimal motion planning case-study of a serial manipulator pick-and-place application. The resulting design determines a feasible effort optimal motion plan subject to actuator and obstacle avoidance constraints for all possible systems within the probability space. Variance of the system's terminal conditions are also minimized in a Pareto optimal sense. The inverse dynamics formulation (using deterministic state trajectories and uncertain actuator inputs) is presented in a companion paper.
更多查看译文
关键词
Motion Planning,Trajectory Planning,Optimization,Nonlinear Programming,Multibody Dynamics,Uncertainty Quantification
AI 理解论文
溯源树
样例
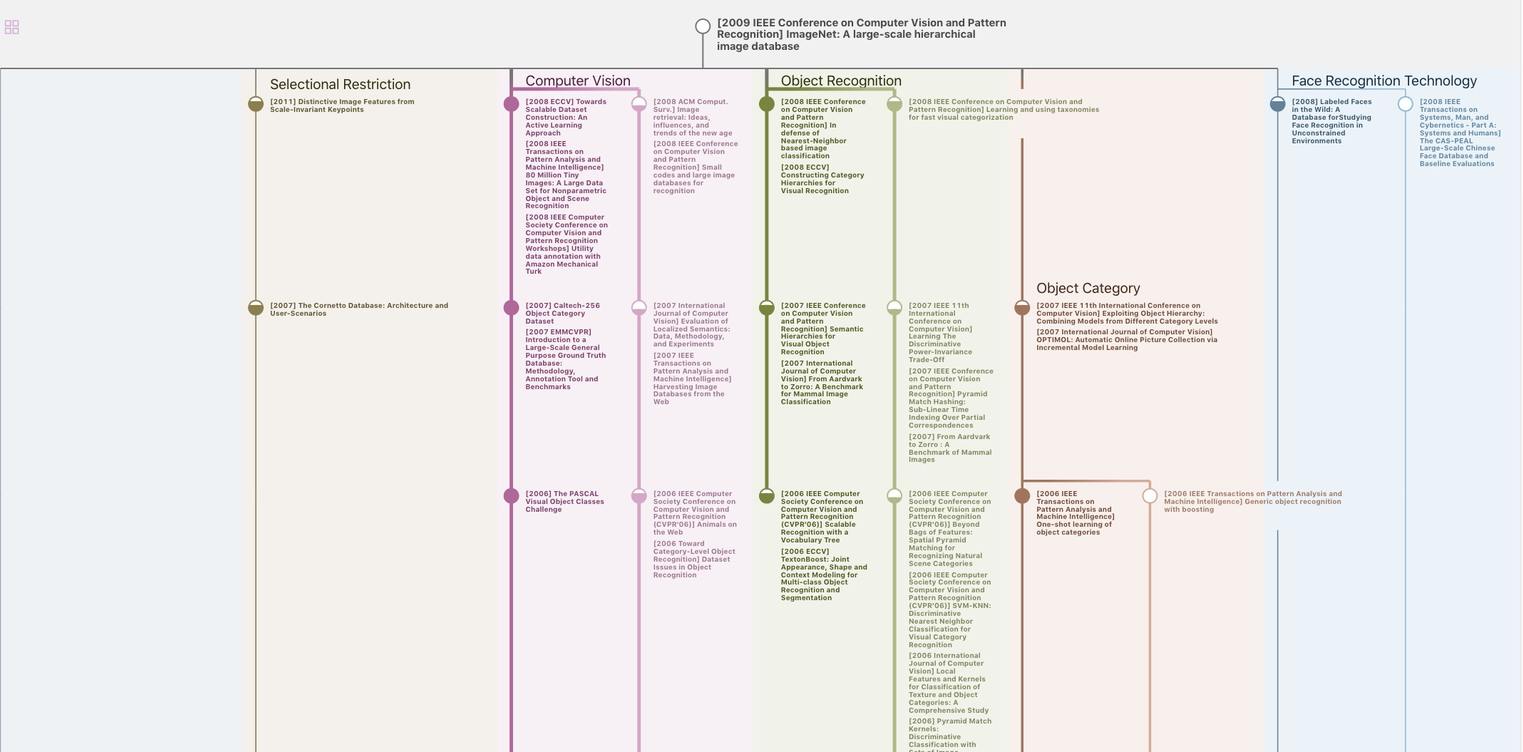
生成溯源树,研究论文发展脉络
Chat Paper
正在生成论文摘要