Using Machine Learning For Behavior-Based Access Control: Scalable Anomaly Detection On Tcp Connections And Http Requests
2013 IEEE MILITARY COMMUNICATIONS CONFERENCE (MILCOM 2013)(2013)
摘要
Today's business processes are more connected than ever before, driven by the ability to share the right information with the right partners at the right time. While this interconnectedness and situational awareness is crucial to success, it also opens the possibility for misuse of the same capabilities by sophisticated adversaries to spread attacks and exfiltrate or corrupt critical sensitive information. We have been investigating means to analyze behaviors of actors and assess trustworthiness of information to support real-time cyber security decision making through a concept called Behavior-Based Access Control (BBAC). The work described in this paper focuses on the statistical machine learning techniques used in BBAC to make predictions about the intent of actors establishing TCP connections and issuing HTTP requests. We discuss pragmatic challenges and solutions we encountered in implementing and evaluating BBAC, discussing (a) the general concepts underlying BBAC, (b) challenges we have encountered in identifying suitable datasets, (c) mitigation strategies to cope with shortcomings in available data, (d) the combination of clustering and support vector machines for performing classification at scale, and (e) results from a number of scientific experiments. We also include expert commentary from Air Force stakeholders and describe current plans for transitioning BBAC capabilities into the Department of Defense together with lessons learned for the machine learning community.
更多查看译文
关键词
transport protocols,authorisation,classification,scaling factor,statistical analysis,learning artificial intelligence,parallel processing
AI 理解论文
溯源树
样例
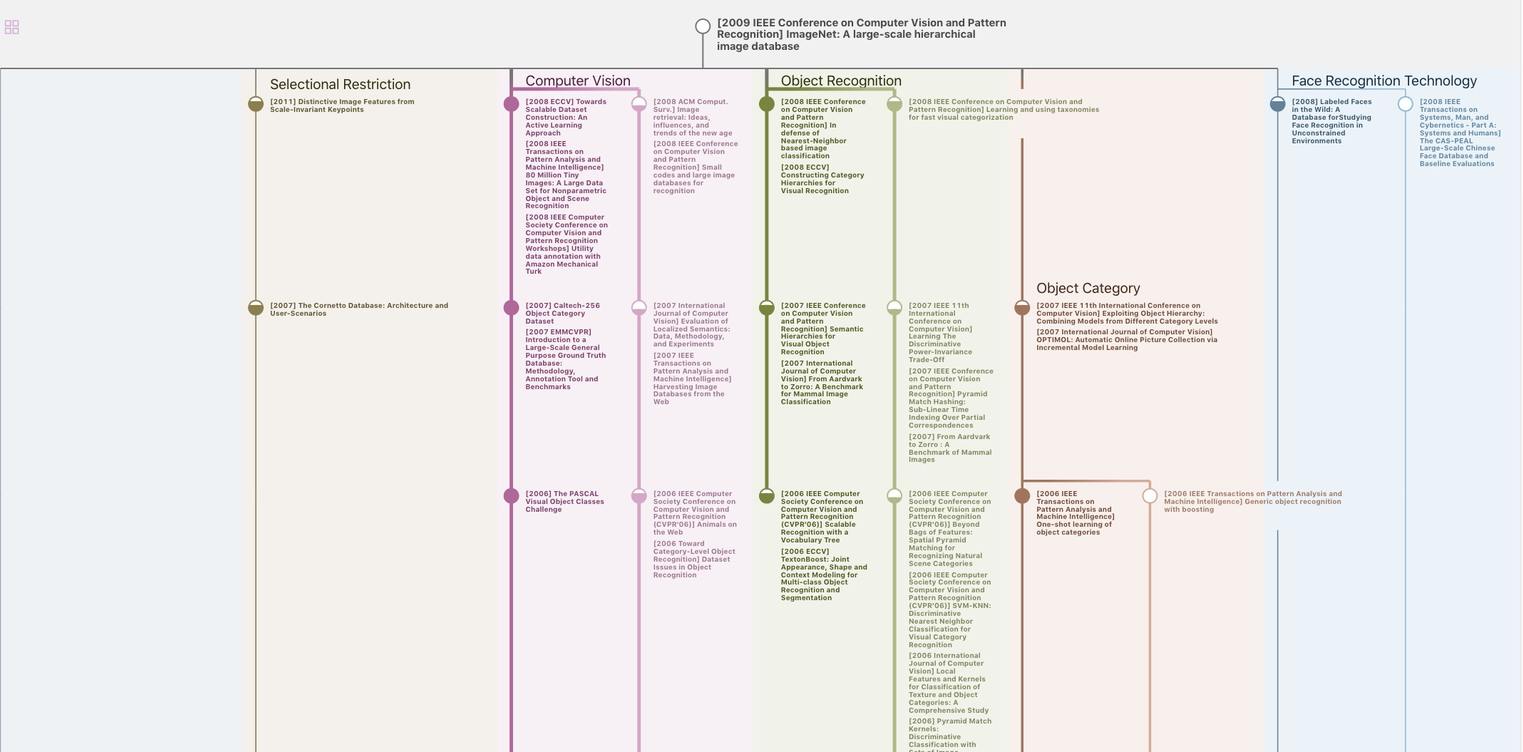
生成溯源树,研究论文发展脉络
Chat Paper
正在生成论文摘要