Automated Endmember Determination and Adaptive Spectral Mixture Analysis Using Kernel Methods
Proceedings of SPIE, the International Society for Optical Engineering/Proceedings of SPIE(2013)
摘要
Various phenomena occur in geographic regions that cause pixels of a scene to contain spectrally mixed pixels. The mixtures may be linear or nonlinear. It could simply be that the pixel size of a sensor is too large so many pixels contain patches of different materials within them (linear), or there could be microscopic mixtures and multiple scattering occurring within pixels (non-linear). Often enough, scenes may contain cases of both linear and non-linear mixing on a pixel-by-pixel basis. Furthermore, appropriate endmembers in a scene are not always easy to determine. A reference spectral library of materials may or may not be available, yet, even if a library is available, using it directly for spectral unmixing may not always be fruitful. This study investigates a generalized kernel-based method for spectral unmixing that attempts to determine if each pixel in a scene is linear or non-linear, and adapts to compute a mixture model at each pixel accordingly. The effort also investigates a kernel-based support vector method for determining spectral endmembers in a scene. Two scenes of hyperspectral imagery calibrated to reflectance are used to validate the methods. We test the approaches using a HyMAP scene collected over the Waimanalo Bay region in Oahu, Hawaii, as well as an AVIRIS scene collected over the oil spill region in the Gulf of Mexico during the Deepwater Horizon oil incident.
更多查看译文
关键词
Kernel methods,linear spectral mixing,non-linear spectral mixing,endmember determination,hyperspectral
AI 理解论文
溯源树
样例
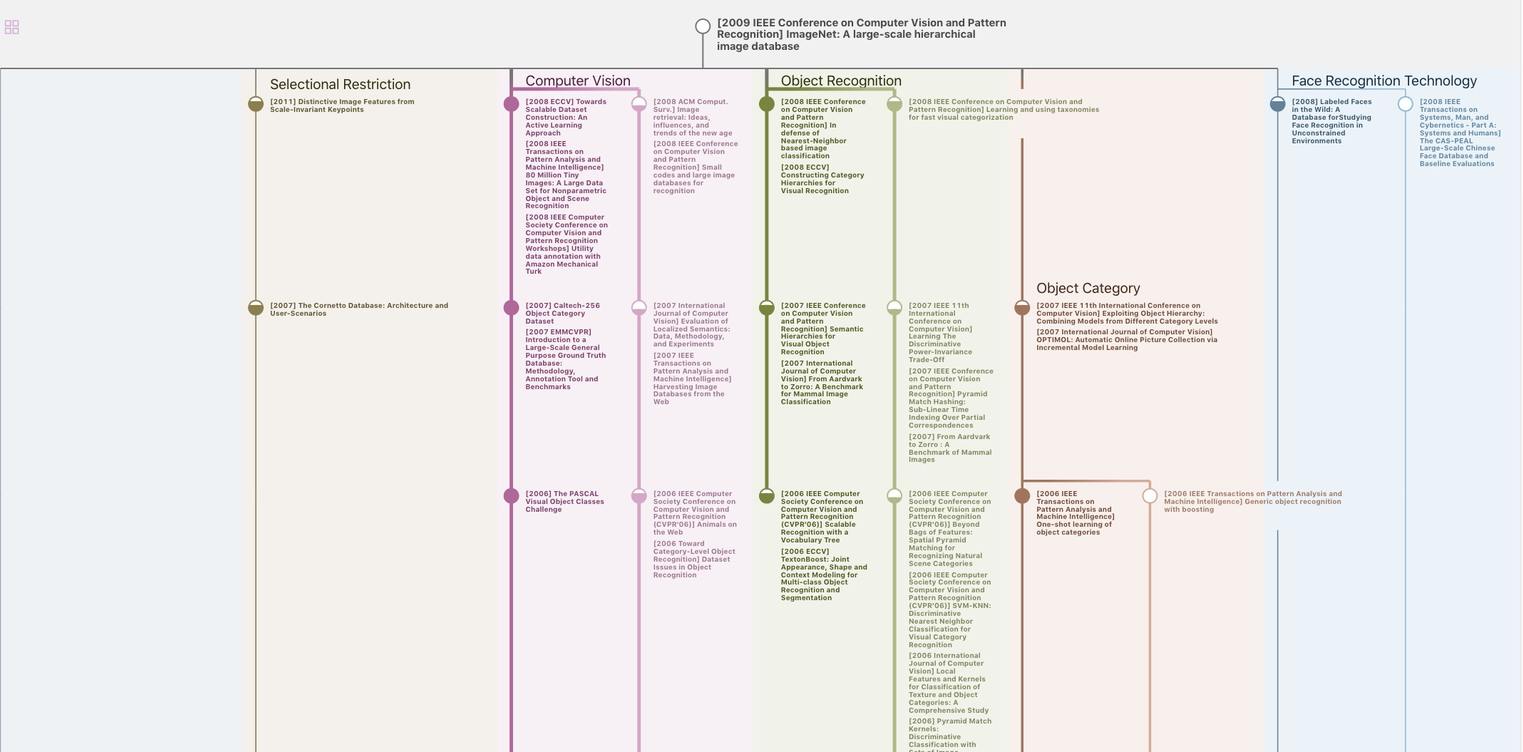
生成溯源树,研究论文发展脉络
Chat Paper
正在生成论文摘要