The MCMC split sampler: A block Gibbs sampling scheme for latent Gaussian models
mag(2015)
摘要
A novel computationally efficient Markov chain Monte Carlo (MCMC) scheme for latent Gaussian models (LGMs) is proposed in this paper. The sampling scheme is a two block Gibbs sampling scheme designed to exploit the model structure of LGMs. We refer to the proposed sampling scheme as the MCMC split sampler. The principle idea behind the MCMC split sampler is to split the latent Gaussian parameters into two vectors. The former vector consists of latent parameters which appear in the data density function, while the latter vector consists of latent parameters which do not appear in it. The former vector is placed in the first block of the proposed sampling scheme and the latter vector is placed in the second block along with any potential hyperparameters. The resulting conditional posterior density functions within the blocks allow the MCMC split sampler to handle, by design, LGMs with latent models imposed on more than just the mean structure of the data density function. The MCMC split sampler is also designed to be applicable for any choice of a parametric data density function. Moreover, it scales well in terms of computational efficiency when the dimension of the latent model increase.
更多查看译文
AI 理解论文
溯源树
样例
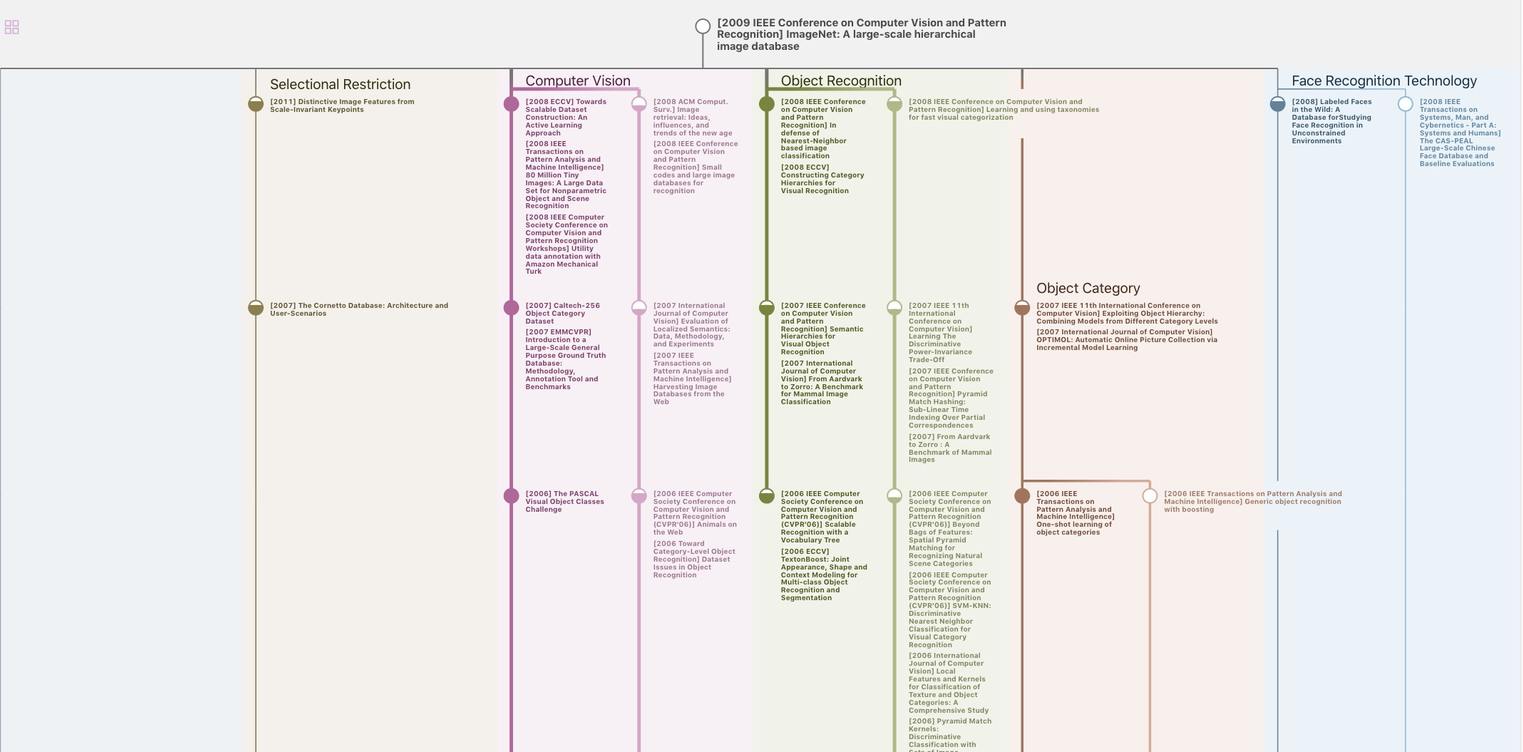
生成溯源树,研究论文发展脉络
Chat Paper
正在生成论文摘要