A new way to learn acoustic events
mag(2013)
摘要
Most speech recognition systems still use Mel Frequency Cepstral Coefficients (MFCC’s) or Perceptual Linear Prediction Coefficients because these preserve a lot of the information required for recognition while being much more compact than a high-resolution spectrogram. As computers get faster and methods of modeling high-dimensional data improve, however, high-resolution spectrograms or other very high-dimensional representations of the sound wave become more attractive. They have already surpassed MFCC’s for some tasks [1]. Psychologists have argued that high-quality recognition would be facilitated by finding acoustic events or landmarks that have well-defined onset times, amplitudes and rates in addition to being present or absent. We introduce a new way of learning such acoustic events by using a new type of autoencoder that is given both a spectrogram and a desired global transformation and learns to output the transformed spectrogram. By specifying the global transformation in the appropriate way, we can force the autoencoder to extract accoustic events that, in addition to a probability of being present, have explicit onset times, amplitudes and rates. This makes it much easier to compute relationships between acoustic events.
更多查看译文
AI 理解论文
溯源树
样例
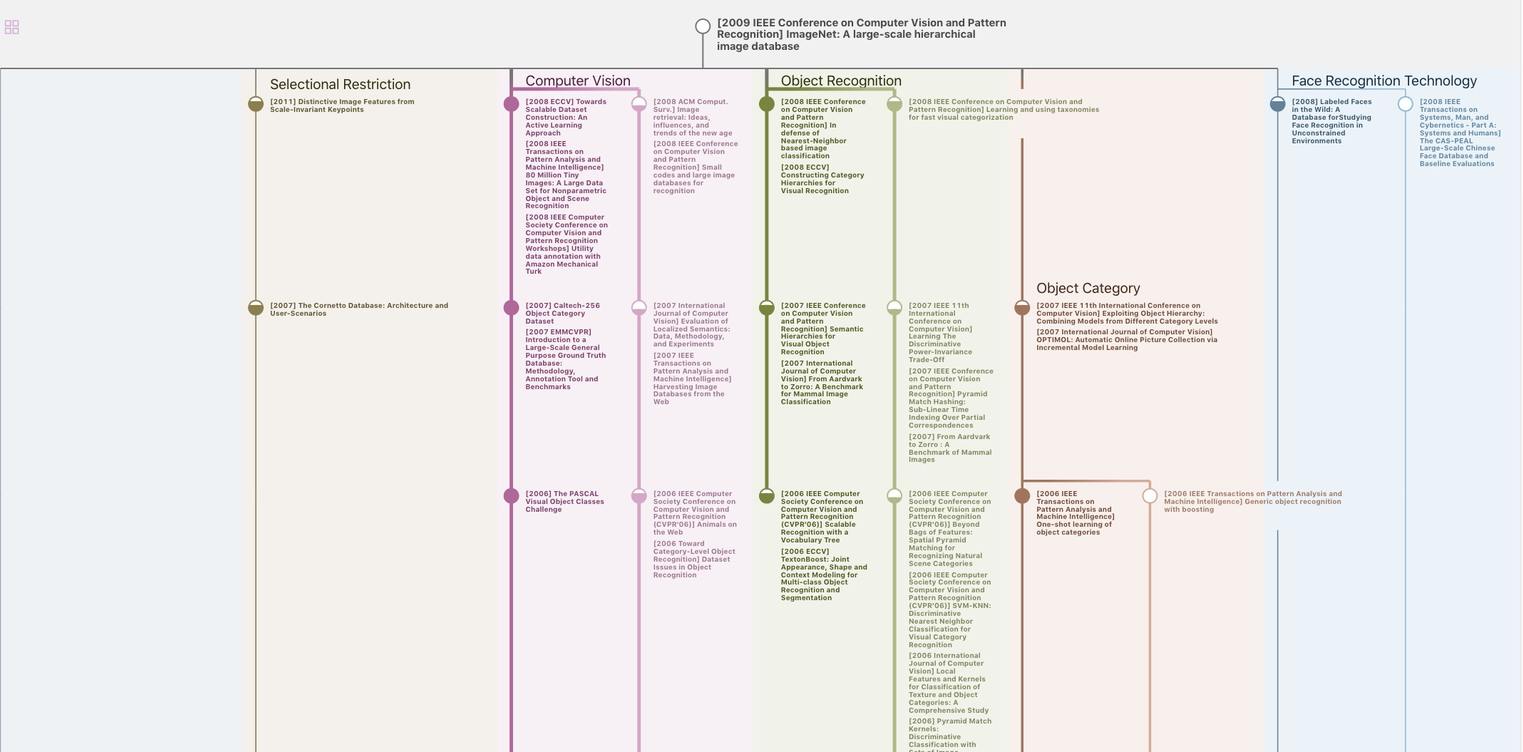
生成溯源树,研究论文发展脉络
Chat Paper
正在生成论文摘要