Data Uncertainty in Markov Chains: Application to Cost-Effectiveness Analyses of Medical Innovations
Periodicals(2018)
摘要
AbstractCost-effectiveness studies of medical innovations often suffer from data inadequacy. When Markov chains are used as a modeling framework for such studies, this data inadequacy can manifest itself as imprecision in the elements of the transition matrix. In this paper, we study how to compute maximal and minimal values for the discounted value of the chain with respect to a vector of state-wise costs or rewards as these uncertain transition parameters jointly vary within a given uncertainty set. We show that these problems are computationally tractable if the uncertainty set has a row-wise structure. Conversely, we prove that the row-wise structure is necessary for tractability. Without it, the problems become computationally intractable strongly NP-hard. We apply our model to assess the cost-effectiveness of fecal immunochemical testing FIT, a new screening method for colorectal cancer. Our results show that despite the large uncertainty in FIT's performance, it could be cost-effective relative to the prevailing screening method of colonoscopy.The online appendix is available at https://doi.org/10.1287/opre.2017.1685.
更多查看译文
关键词
cost-effectiveness analysis,Markov models,uncertain parameters,sensitivity analysis
AI 理解论文
溯源树
样例
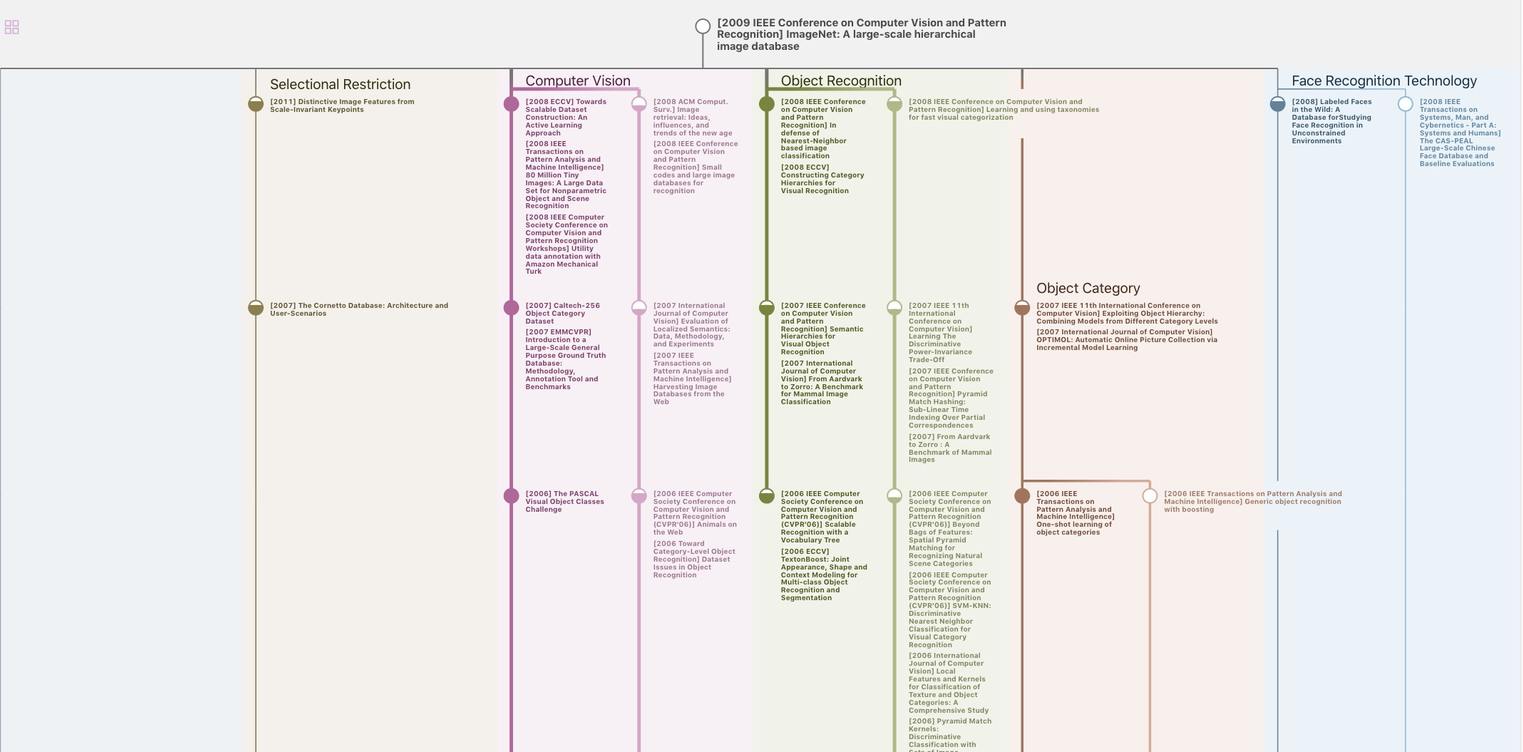
生成溯源树,研究论文发展脉络
Chat Paper
正在生成论文摘要