Multi-Kernel Partial Least Squares Regression based on Adaptive Genetic Algorithm
PROCEEDINGS OF THE 2015 INTERNATIONAL CONFERENCE ON AUTOMATION, MECHANICAL CONTROL AND COMPUTATIONAL ENGINEERING(2015)
摘要
Kernel learning has been a focus of machine learning domain recently. Kernel partial least squares (KPLS) algorithm can construct nonlinear model using extract latent variables from the input and output data space simultaneously. However, generalization performance of KPLS model relies mostly on kernel types and kernel parameters, which are difference to modeling of different applicable background. Intelligent optimization algorithm can be used to search these parameters. Thus, a new multi-kernel partial least squares regression approach based on linear multi-kernel construction method and adaptive genetic algorithm (AGA) is proposed in this paper. Normally used global and local kernels are weighed to obtain the mixed multi-kernel of KPLS algorithm. These kernel's parameters and weighting coefficients are selected using AGA optimization algorithm. The experimental results based on Benchmark data set show that the proposed approach has better prediction performance than that of single kernel based modeling method.
更多查看译文
关键词
Multi-kernel learning (MKL),Kernel partial least squares (KPLS),Adaptive genetic algorithm (AGA)
AI 理解论文
溯源树
样例
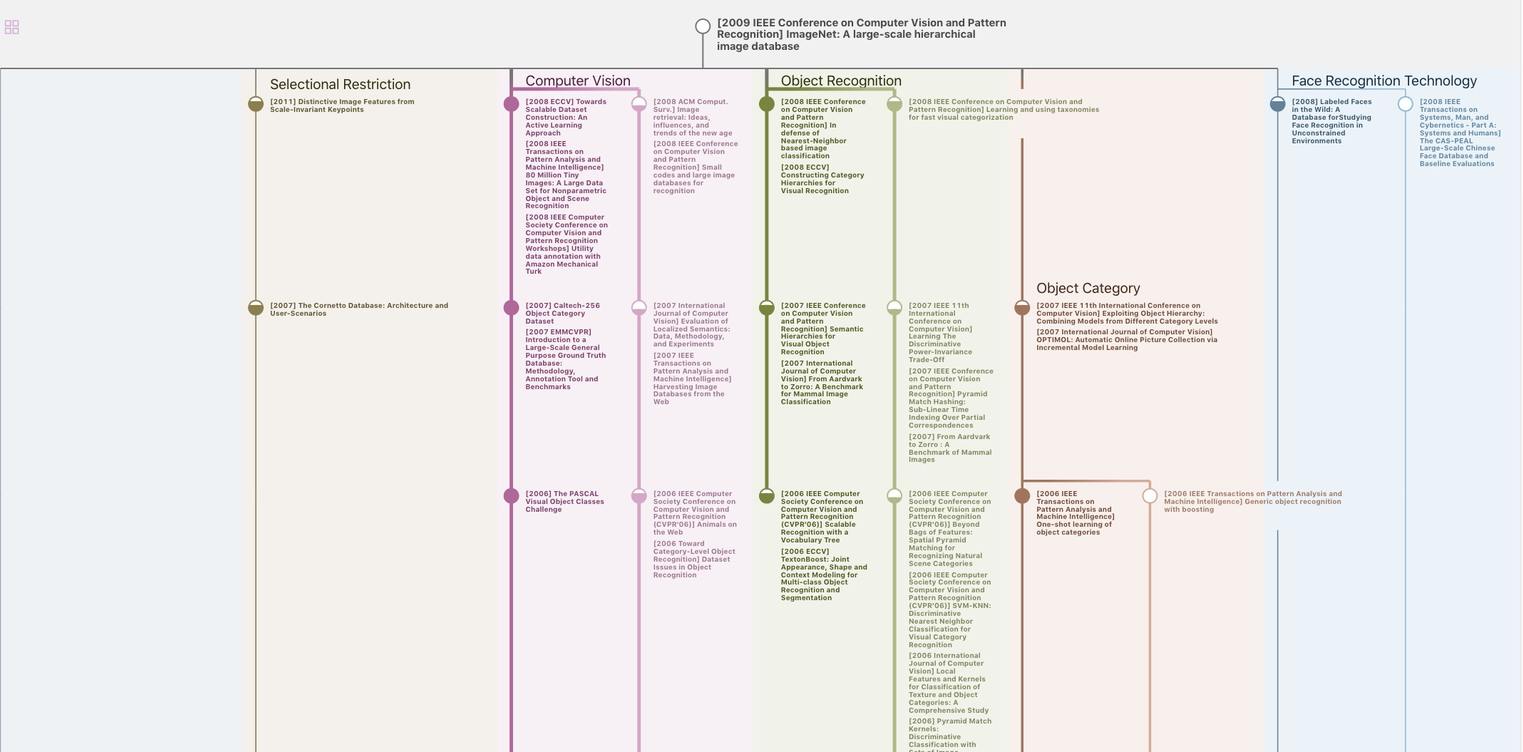
生成溯源树,研究论文发展脉络
Chat Paper
正在生成论文摘要