A Rank-SVM Approach to Anomaly Detection
arXiv (Cornell University)(2014)
摘要
We propose a novel non-parametric adaptive anomaly detection algorithm for high dimensional data based on rank-SVM. Data points are first ranked based on scores derived from nearest neighbor graphs on n-point nominal data. We then train a rank-SVM using this ranked data. A test-point is declared as an anomaly at alpha-false alarm level if the predicted score is in the alpha-percentile. The resulting anomaly detector is shown to be asymptotically optimal and adaptive in that for any false alarm rate alpha, its decision region converges to the alpha-percentile level set of the unknown underlying density. In addition we illustrate through a number of synthetic and real-data experiments both the statistical performance and computational efficiency of our anomaly detector.
更多查看译文
关键词
anomaly detection,rank-svm
AI 理解论文
溯源树
样例
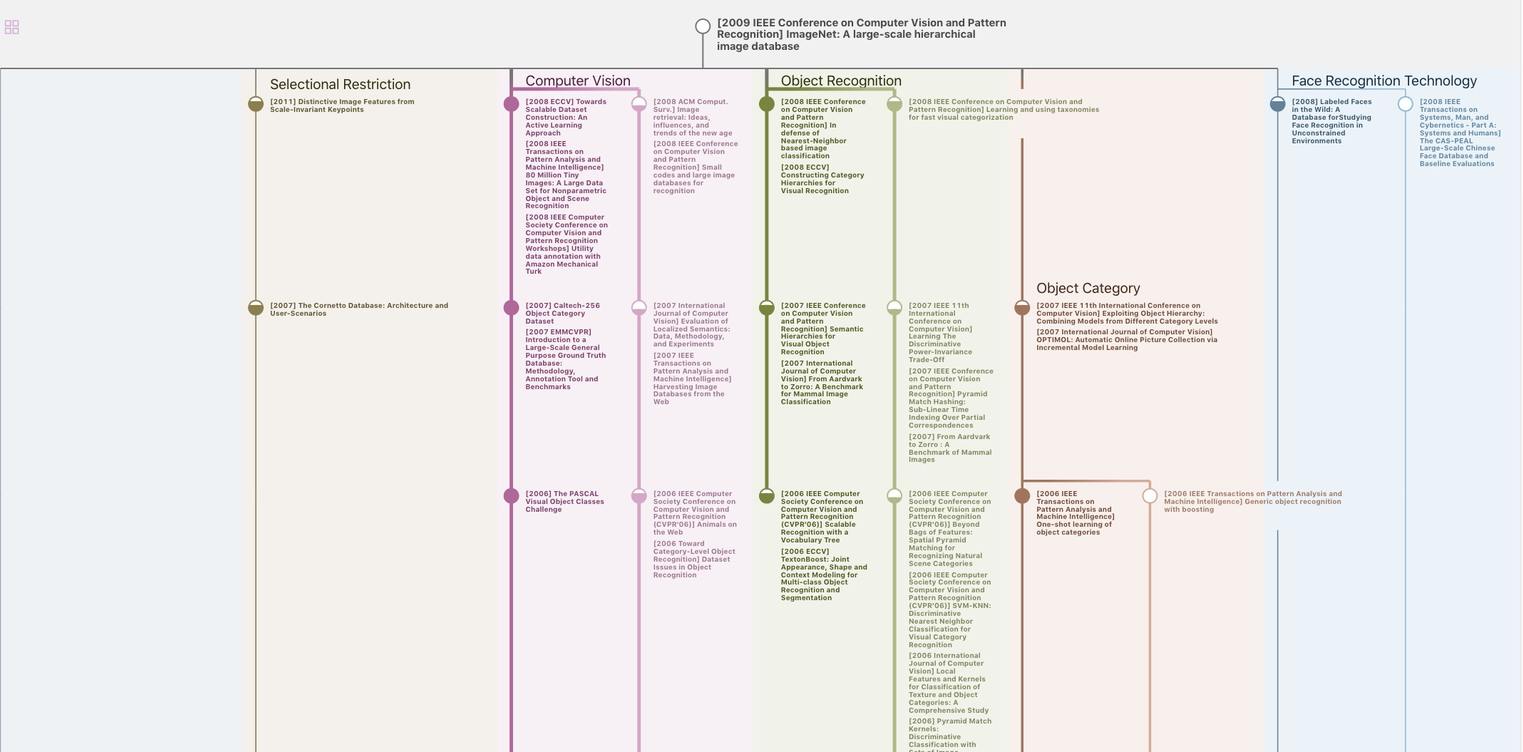
生成溯源树,研究论文发展脉络
Chat Paper
正在生成论文摘要