Multi-agent Communication meets Natural Language: Synergies between Functional and Structural Language Learning
ACL(2020)
摘要
We present a method for combining multi-agent communication and traditional data-driven approaches to natural language learning, with an end goal of teaching agents to communicate with humans in natural language. Our starting point is a language model that has been trained on generic, not task-specific language data. We then place this model in a multi-agent self-play environment that generates task-specific rewards used to adapt or modulate the model, turning it into a task-conditional language model. We introduce a new way for combining the two types of learning based on the idea of reranking language model samples, and show that this method outperforms others in communicating with humans in a visual referential communication task. Finally, we present a taxonomy of different types of language drift that can occur alongside a set of measures to detect them.
更多查看译文
关键词
structural language learning,natural language,communication,multi-agent
AI 理解论文
溯源树
样例
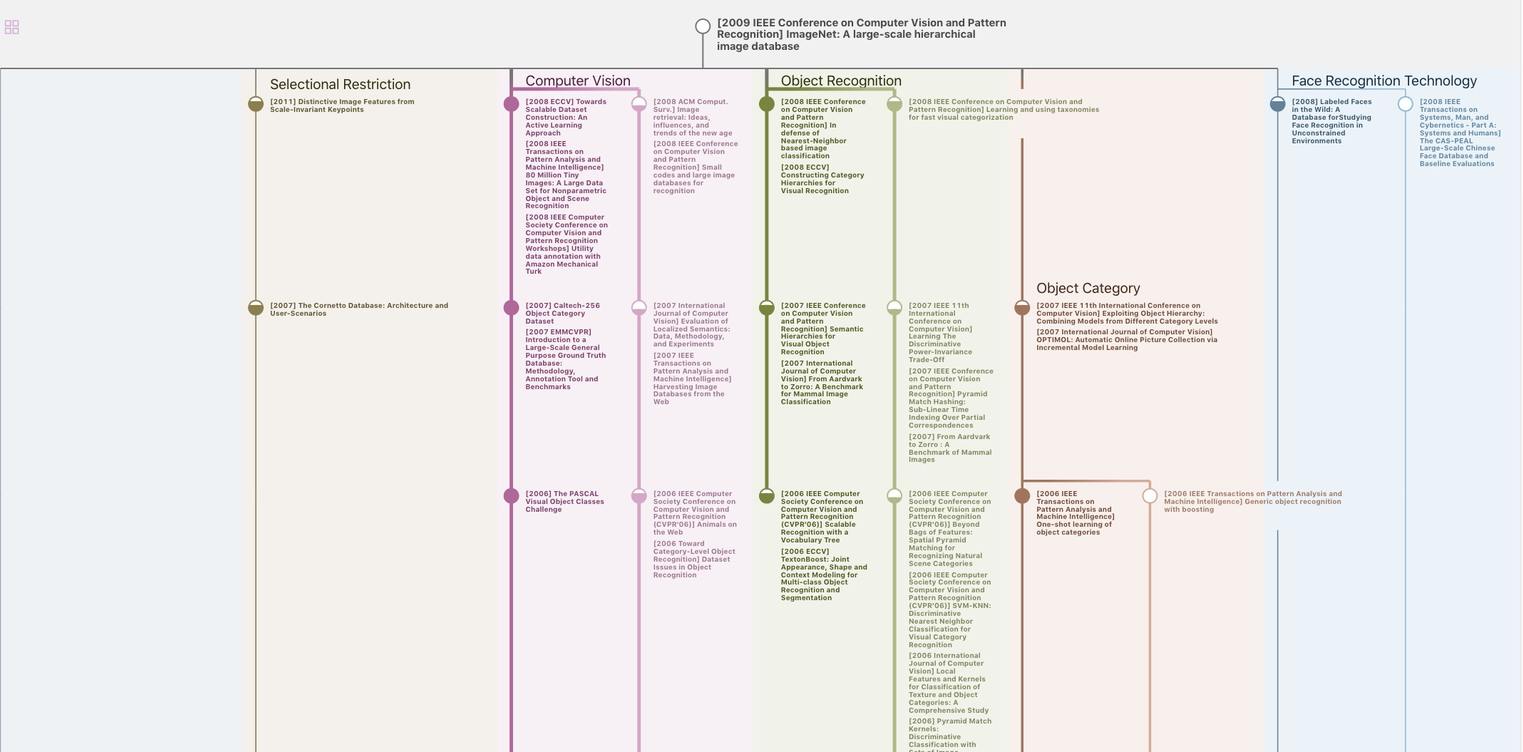
生成溯源树,研究论文发展脉络
Chat Paper
正在生成论文摘要