Active Covariance Matrix Adaptation For Multi-Objective Cma-Es
2013 SIXTH INTERNATIONAL CONFERENCE ON ADVANCED COMPUTATIONAL INTELLIGENCE (ICACI)(2013)
摘要
This paper proposes a novel approach for a derandomized covariance matrix adaptation for multi-objective optimization. Common derandomized multi-objective algorithms only utilize the information gained from successful mutations. However in case of optimization problems with a limited budget for fitness evaluations inferior mutations provide additional information to adjust the search. The proposed algorithm, called active-(mu+lambda)-MO-CMA-ES, extends previous approaches as it reduces the covariance along directions of unsuccessful mutations. In experiments on a set of commonly accepted multi-objective test problems the presented algorithm outperforms other derandomized evolution strategies.
更多查看译文
关键词
evolutionary computation
AI 理解论文
溯源树
样例
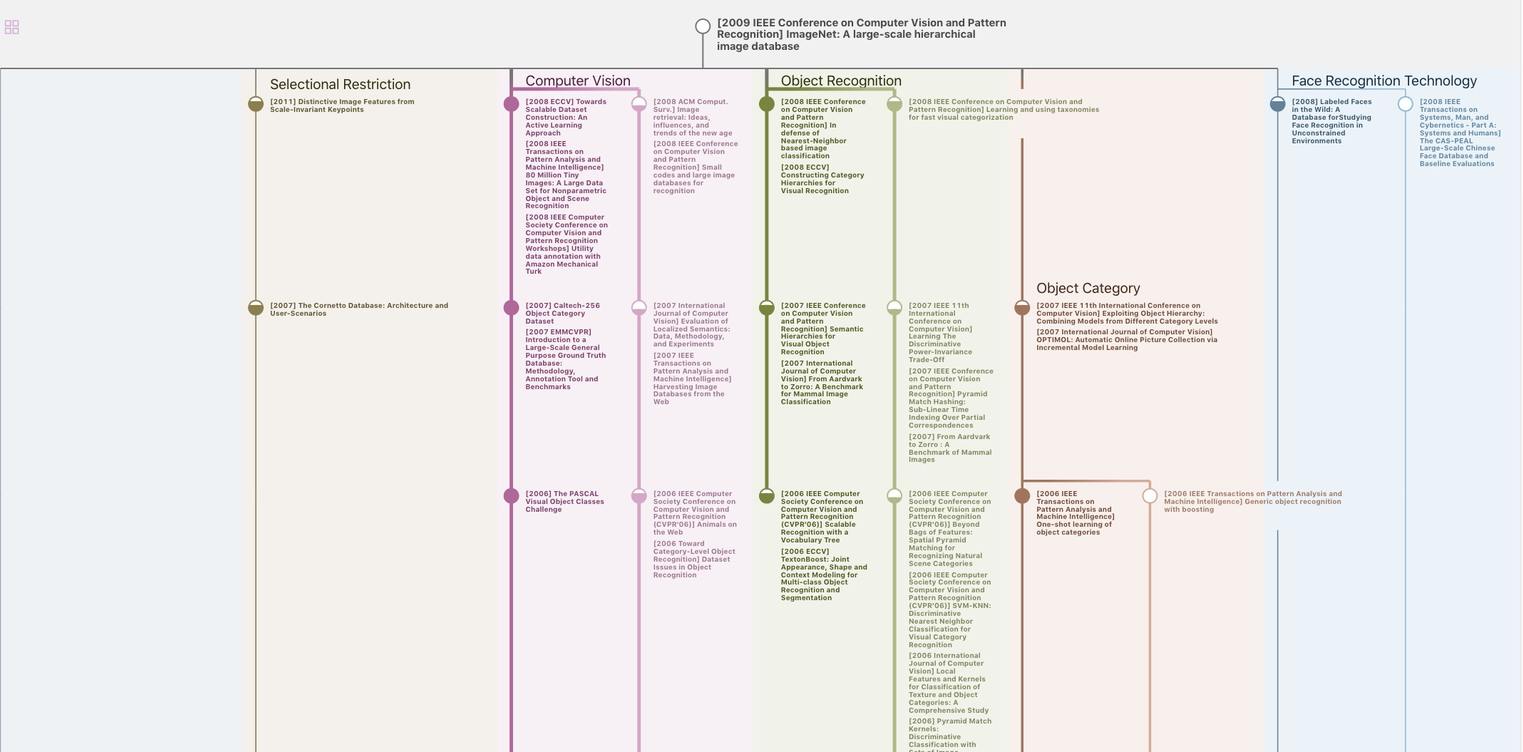
生成溯源树,研究论文发展脉络
Chat Paper
正在生成论文摘要