A Predictive Perspective on Measures of Influence in Networks
mag(2010)
摘要
Identifying the most important or prominent actors in a network has been an area of much interest in Social Network Analysis dating back to Moreno’s work in the 1930’s [1]. This interest has spurred the formulation of many graph-based sociometrics for ranking actors in complex physical, biological and social networks. These sociometrics are usually based on intuitive notions such as access and control over resources, or brokerage of information [2]; and has yielded measures such as Degree Centrality, Closeness Centrality and Betweeness Centrality [3]. In the exploratory analysis of networks, the question of whether these measures of centrality really capture what we mean by “importance” is often not directly addressed. However, when such sociometrics start being used to drive decisions in more quantitative fields, there emerges a need to empirically answer this question. Probably the most popular of these measures in the Computer Science community is PageRank, which is a variant of Eigenvector Centrality [4]. Once its use in Information Retrieval (IR) and Web search in particular became popular, it led to more rigorous evaluation of PageRank and variants on measurable IR tasks [5]. With the rise of Web 2.0, with its focus on user-generated content and social networks, various socio-metrics are being increasingly used to produce ranked lists of “top” bloggers, twitterers, etc. For example, Twitterholic.com and WeFollow.com use degree centrality (number of followers), while TunkRank.com uses a variant of PageRank to rank users of the micro-blogging service Twitter. In the domain of blogs, Technorati assigns an authority score to a blogger based on the number of blogs linking to her website in the last six months. Similarly, Blogpulse ranks blogs based on the number of times it’s cited by other bloggers over the last 30 days. Do these rankings really identify “influential” authors, and if so, which ranking is better? With the increased demand for Social Media Analytics, with its focus on deriving marketing insight from the analysis of blogs and other social media, there’s a growing need to address this question. This paper is a step in that direction. It is our position, that the question of whether a particular influence measure is good is ill-posed, unless it is put in the context of a measurable task or desired outcome. Constructing such predictive tasks of interest, not only guides the choice of relationships we build a network on, but also allows for the quantitative comparison of different socio-metrics. In this paper, we present a case study on data collected for 40 million Twitter accounts. We look at marketing-driven tasks, such as detecting the potential for viral outbreaks of messages (tweets). We build three different graphs based on the network of followers, rebroadcast (retweet) networks, and the network of replies and mentions. We conduct a similar study on detecting the influence of publications, through the analysis of citation networks. Extensive empirical results demonstrate that different measures provide the best ranking for these tasks – underscoring the importance of addressing the question of influence based on a desired objective. Taking a predictive perspective of measures of influence can also suggest alternative socio-metrics, and we show that combining aspects of different measures produces a composite ranking mechanism that is most beneficial for each desired predictive task. We compare several approaches to combining influence measures through rank aggregation methods, such as approximations of Kemeny optimal aggregation [6]. In addition, we introduce novel supervised rank aggregation techniques that leverage the ground truth on a subset of users to further improve ranking. We demonstrate the efficacy of these methods compared to several baseline approaches.
更多查看译文
AI 理解论文
溯源树
样例
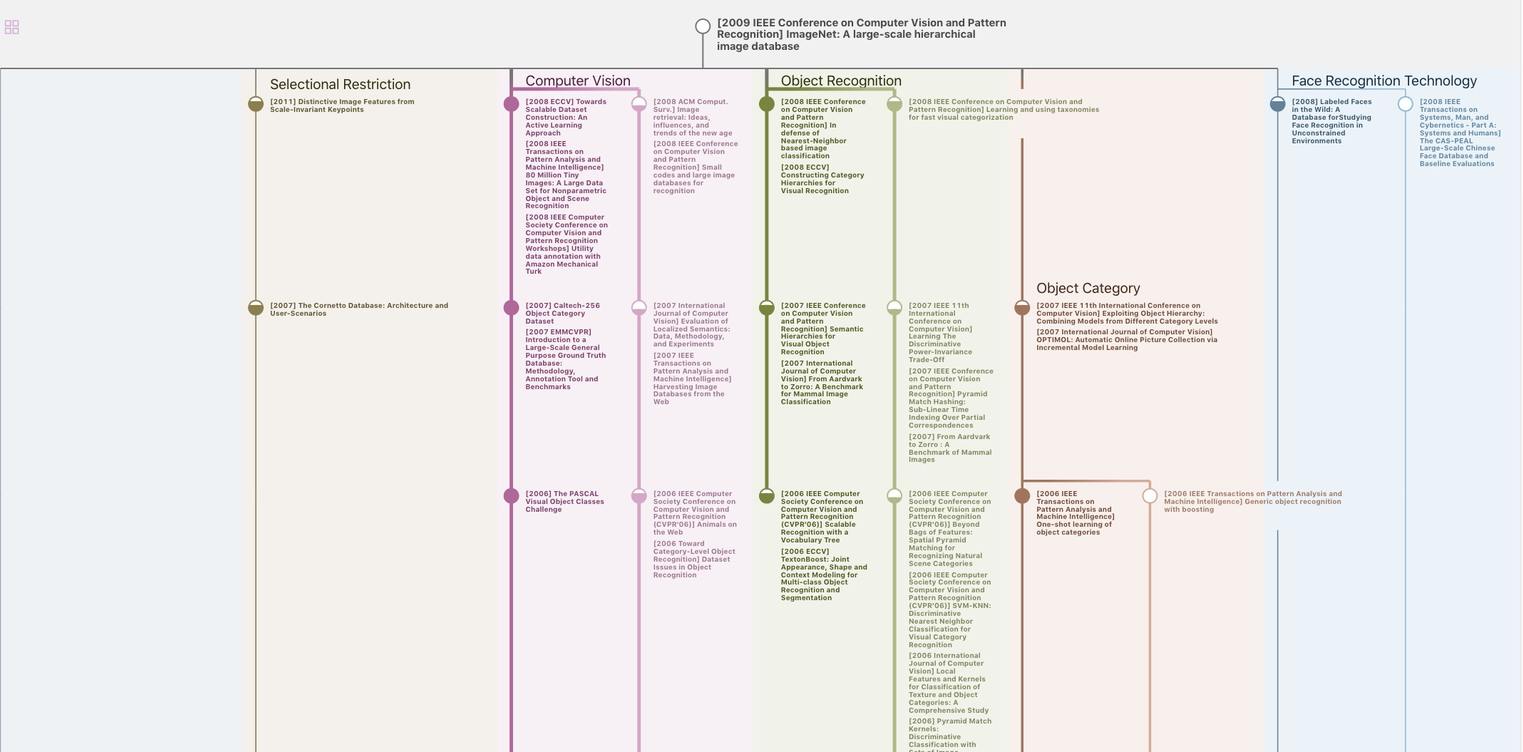
生成溯源树,研究论文发展脉络
Chat Paper
正在生成论文摘要