Feature transformation of neural activity with sparse and low-rank decomposition
Proceedings of SPIE(2015)
摘要
We propose a novel application of the sparse and low-rank (SLR) decomposition method to decode cognitive states for concept activity measured using fMRI BOLD. Current decoding methods attempt to reduce the dimensionality of fMRI BOLD signals to increase classification rate, but do not address the separable issues of multiple noise sources and complexity in the underlying data. Our feature transformation method extends SLR to separate task activity from the resting state and extract concept specific cognitive state. We show a significant increase in single trial decoding of concepts from fMRI BOLD using SLR to extract task specific cognitive state.
更多查看译文
关键词
Neural activity decoding,neural feature selection,neural feature transformation,sparse and low-rank decomposition
AI 理解论文
溯源树
样例
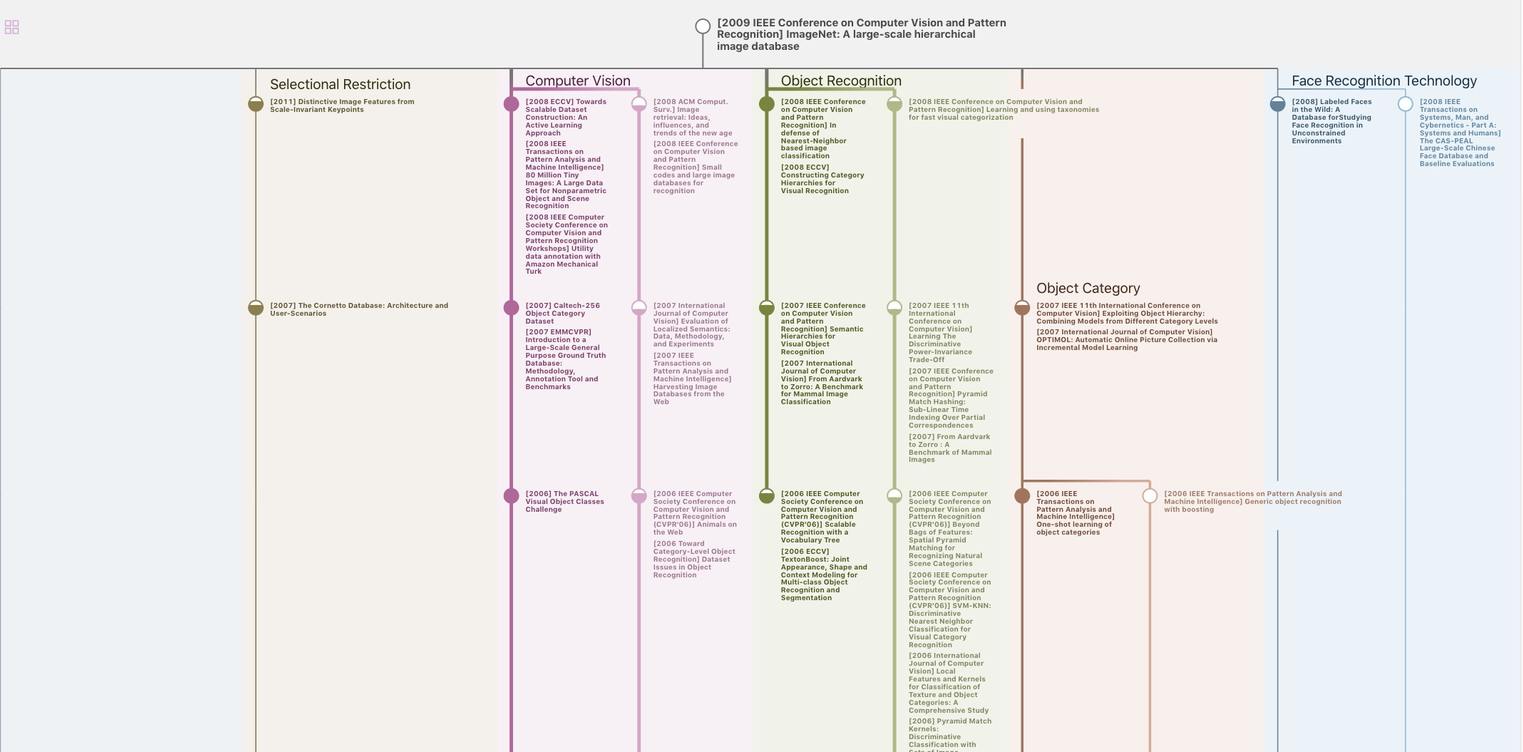
生成溯源树,研究论文发展脉络
Chat Paper
正在生成论文摘要