Variable Selection in General Frailty Models Using Penalized H-Likelihood
JOURNAL OF COMPUTATIONAL AND GRAPHICAL STATISTICS(2014)
摘要
Variable selection methods using a penalized likelihood have been widely studied in various statistical models. However, in semiparametric frailty models, these methods have been relatively less studied because the marginal likelihood function involves analytically intractable integrals, particularly when modeling multicomponent or correlated frailties. In this article, we propose a simple but unified procedure via a penalized h-likelihood (HL) for variable selection of fixed effects in a general class of semiparametric frailty models, in which random effects may be shared, nested, or correlated. We consider three penalty functions (least absolute shrinkage and selection operator [LASSO], smoothly clipped absolute deviation [SCAD], and HL) in our variable selection procedure. We show that the proposed method can be easily implemented via a slight modification to existing HL estimation approaches. Simulation studies also show that the procedure using the SCAD or HL penalty performs well. The usefulness of the new method is illustrated using three practical datasets too. Supplementary materials for the article are available online.
更多查看译文
关键词
LASSO,H-likelihood penalty function,Penalized h-likelihood,Variable selection,SCAD,Frailty models
AI 理解论文
溯源树
样例
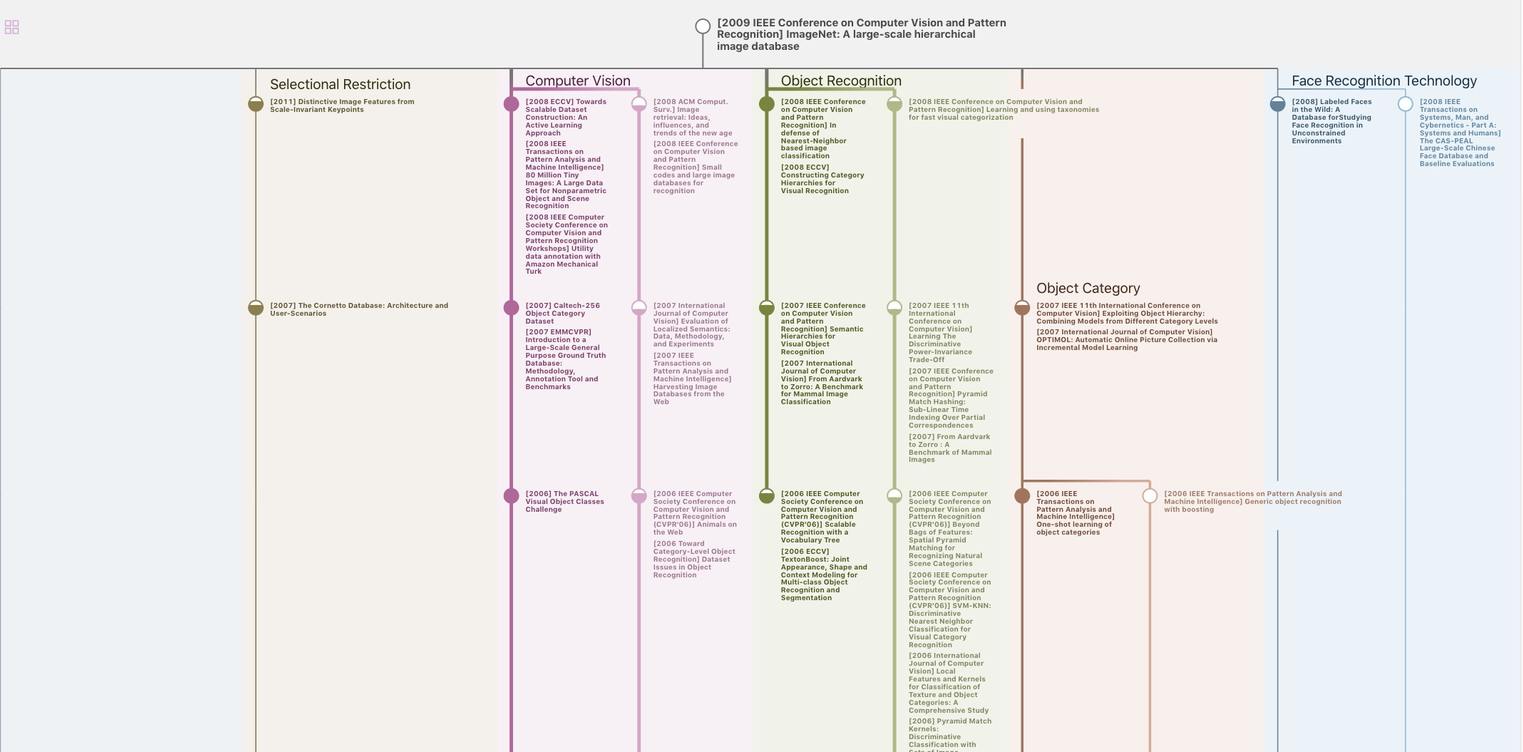
生成溯源树,研究论文发展脉络
Chat Paper
正在生成论文摘要