Development and industrial application of soft sensors with on-line Bayesian model updating strategy
Journal of Process Control(2013)
摘要
This paper deals with the issues associated with the development of data-driven models as well as model update strategy for soft sensor applications. A practical yet effective solution is proposed. Key process variables that are difficult to measure are commonly encountered in practice due to limitations of measurement techniques. Even with appropriate instruments, some measurements are only available through off-line laboratory analysis with typical sampling intervals of several hours. Soft sensors are inferential models that can provide continuous on-line prediction of hidden variables; such models are capable of combining real-time measurements with off-line lab data. Due to the prevalence of plant-model mismatch, it is important to update the model using the latest reference data. In this paper, parameters of data-driven models are estimated using particle filters under the framework of expectation-maximization (EM) algorithms. A Bayesian methodology for model calibration strategy is formulated. The proposed framework for soft sensor development is applied to an industrial process to provide on-line prediction of a quality variable. (C) 2012 Elsevier Ltd. All rights reserved.
更多查看译文
关键词
Soft sensor,Process identification,Bayesian methods,Expectation–maximization algorithm,Particle filtering
AI 理解论文
溯源树
样例
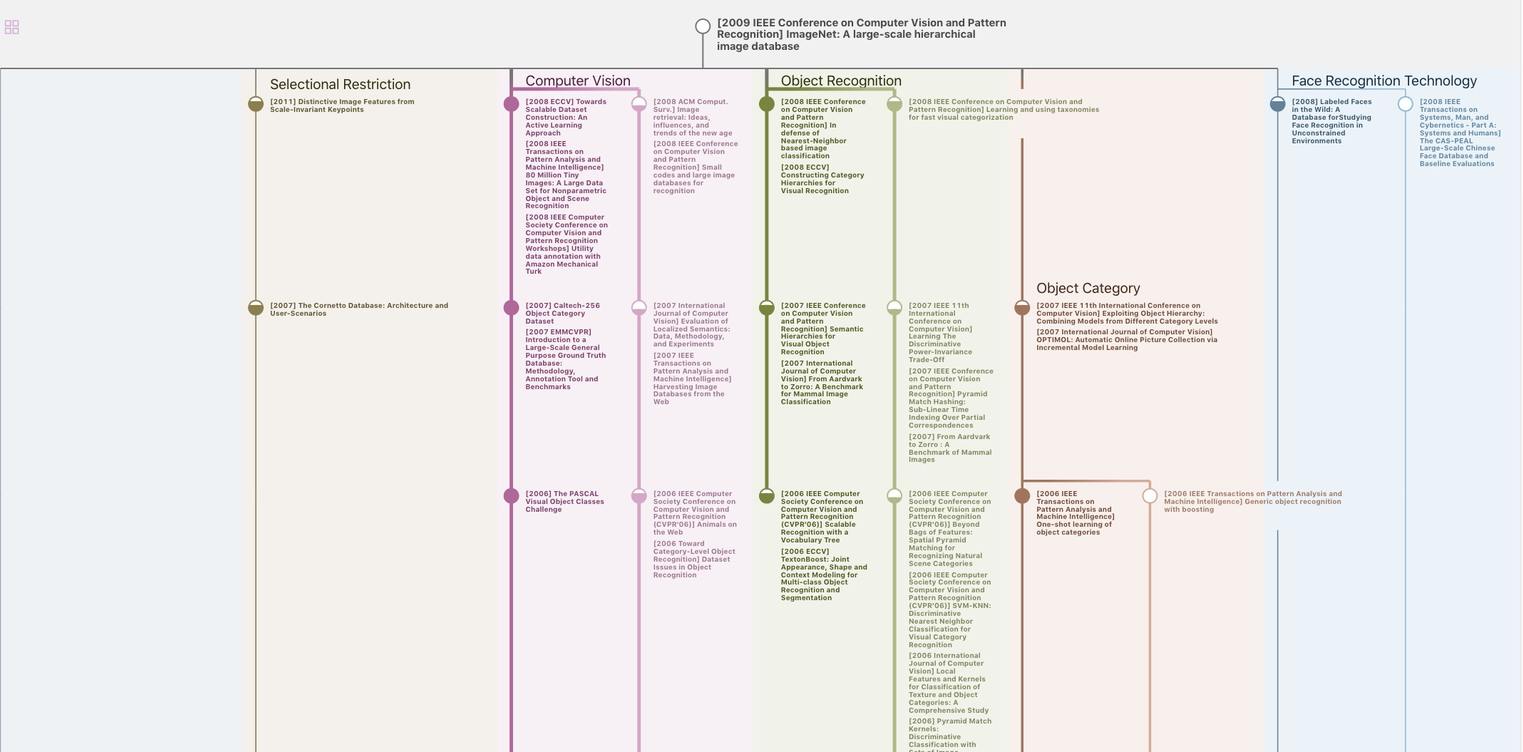
生成溯源树,研究论文发展脉络
Chat Paper
正在生成论文摘要