Real-time capable first principle based modelling of tokamak turbulent transport
NUCLEAR FUSION(2015)
摘要
A real-time capable core turbulence tokamak transport model is developed. This model is constructed from the regularized nonlinear regression of quasilinear gyrokinetic transport code output. The regression is performed with a multilayer perceptron neural network. The transport code input for the neural network training set consists of five dimensions, and is limited to adiabatic electrons. The neural network model successfully reproduces transport fluxes predicted by the original quasilinear model, while gaining five orders of magnitude in computation time. The model is implemented in a real-time capable tokamak simulator, and simulates a 300 s ITER discharge in 10 s. This proof-of-principle for regression based transport models anticipates a significant widening of input space dimensionality and physics realism for future training sets. This aims to provide unprecedented computational speed coupled with first-principle based physics for real-time control and integrated modelling applications.
更多查看译文
关键词
turbulence,tokamak transport,control,integrated modelling
AI 理解论文
溯源树
样例
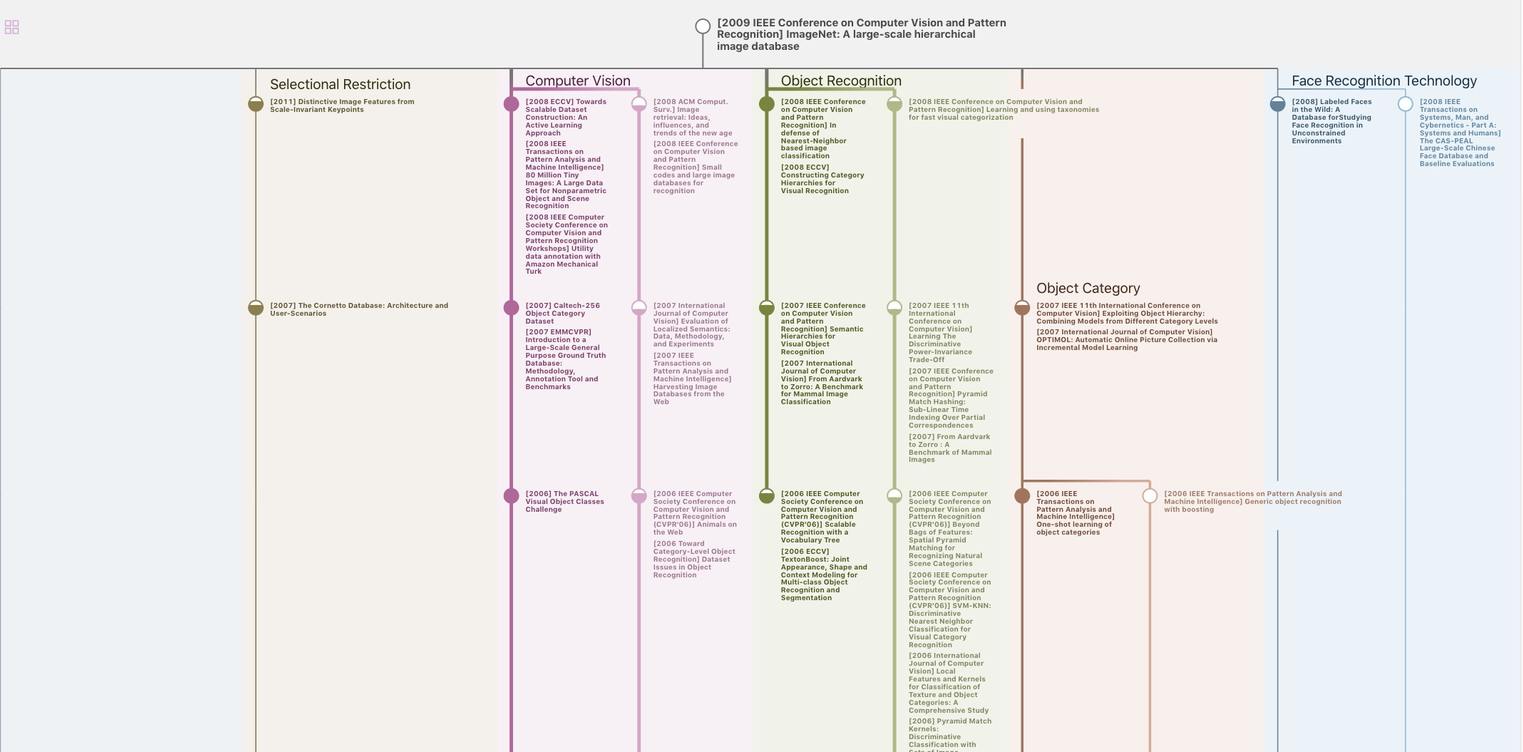
生成溯源树,研究论文发展脉络
Chat Paper
正在生成论文摘要