Compression, Generalization and Learning
JOURNAL OF MACHINE LEARNING RESEARCH(2023)
摘要
A compression function is a map that slims down an observational set into a subset of reduced size, while preserving its informational content. In multiple applications, the con-dition that one new observation makes the compressed set change is interpreted that this observation brings in extra information and, in learning theory, this corresponds to mis-classification, or misprediction. In this paper, we lay the foundations of a new theory that allows one to keep control on the probability of change of compression (which maps into the statistical "risk" in learning applications). Under suitable conditions, the cardi-nality of the compressed set is shown to be a consistent estimator of the probability of change of compression (without any upper limit on the size of the compressed set); more -over, unprecedentedly tight finite-sample bounds to evaluate the probability of change of compression are obtained under a generally applicable condition of preference. All results are usable in a fully agnostic setup, i.e., without requiring any a priori knowledge on the probability distribution of the observations. Not only these results offer a valid support to develop trust in observation-driven methodologies, they also play a fundamental role in learning techniques as a tool for hyp er-parameter tuning.
更多查看译文
关键词
Compression Schemes,Statistical Risk,Statistical Learning Theory,Scenario Approach
AI 理解论文
溯源树
样例
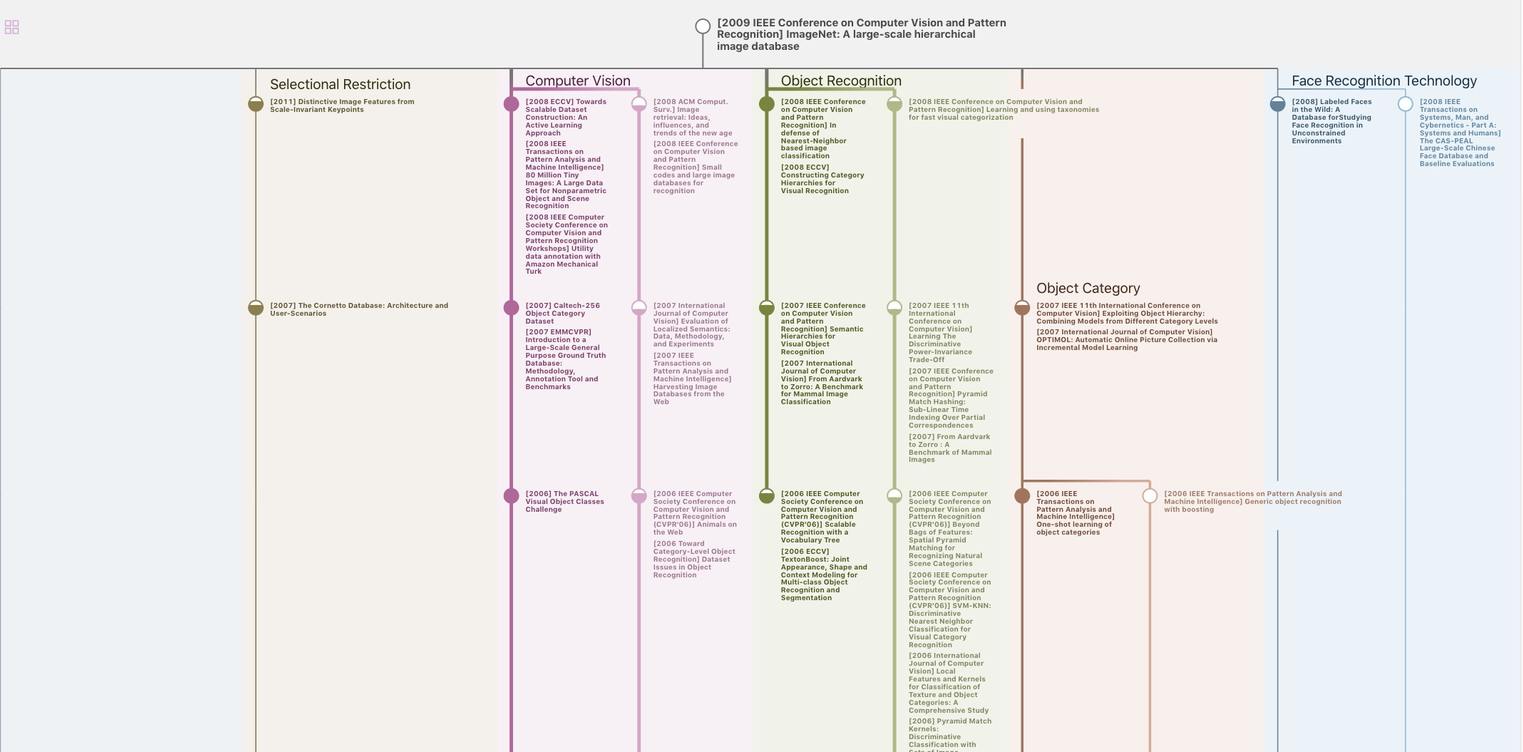
生成溯源树,研究论文发展脉络
Chat Paper
正在生成论文摘要