Efficient automatic search query formulation using phrase-level analysis: Efficient Automatic Search Query Formulation Using Phrase-Level Analysis
Journal of the Association for Information Science and Technology(2014)
摘要
AbstractOver the past decade, the volume of information available digitally over the Internet has grown enormously. Technical developments in the area of search, such as Google's Page Rank algorithm, have proved so good at serving relevant results that Internet search has become integrated into daily human activity. One can endlessly explore topics of interest simply by querying and reading through the resulting links. Yet, although search engines are well known for providing relevant results based on users' queries, users do not always receive the results they are looking for. Google's Director of Research describes clickstream evidence of frustrated users repeatedly reformulating queries and searching through page after page of results. Given the general quality of search engine results, one must consider the possibility that the frustrated user's query is not effective; that is, it does not describe the essence of the user's interest. Indeed, extensive research into human search behavior has found that humans are not very effective at formulating good search queries that describe what they are interested in. Ideally, the user should simply point to a portion of text that sparked the user's interest, and a system should automatically formulate a search query that captures the essence of the text. In this paper, we describe an implemented system that provides this capability. We first describe how our work differs from existing work in automatic query formulation, and propose a new method for improved quantification of the relevance of candidate search terms drawn from input text using phrase-level analysis. We then propose an implementable method designed to provide relevant queries based on a user's text input. We demonstrate the quality of our results and performance of our system through experimental studies. Our results demonstrate that our system produces relevant search terms with roughly two-thirds precision and recall compared to search terms selected by experts, and that typical users find significantly more relevant results 31% more relevant more quickly 64% faster using our system than self-formulated search queries. Further, we show that our implementation can scale to request loads of up to 10 requests per second within current online responsiveness expectations <2-second response times at the highest loads tested.
更多查看译文
关键词
text mining
AI 理解论文
溯源树
样例
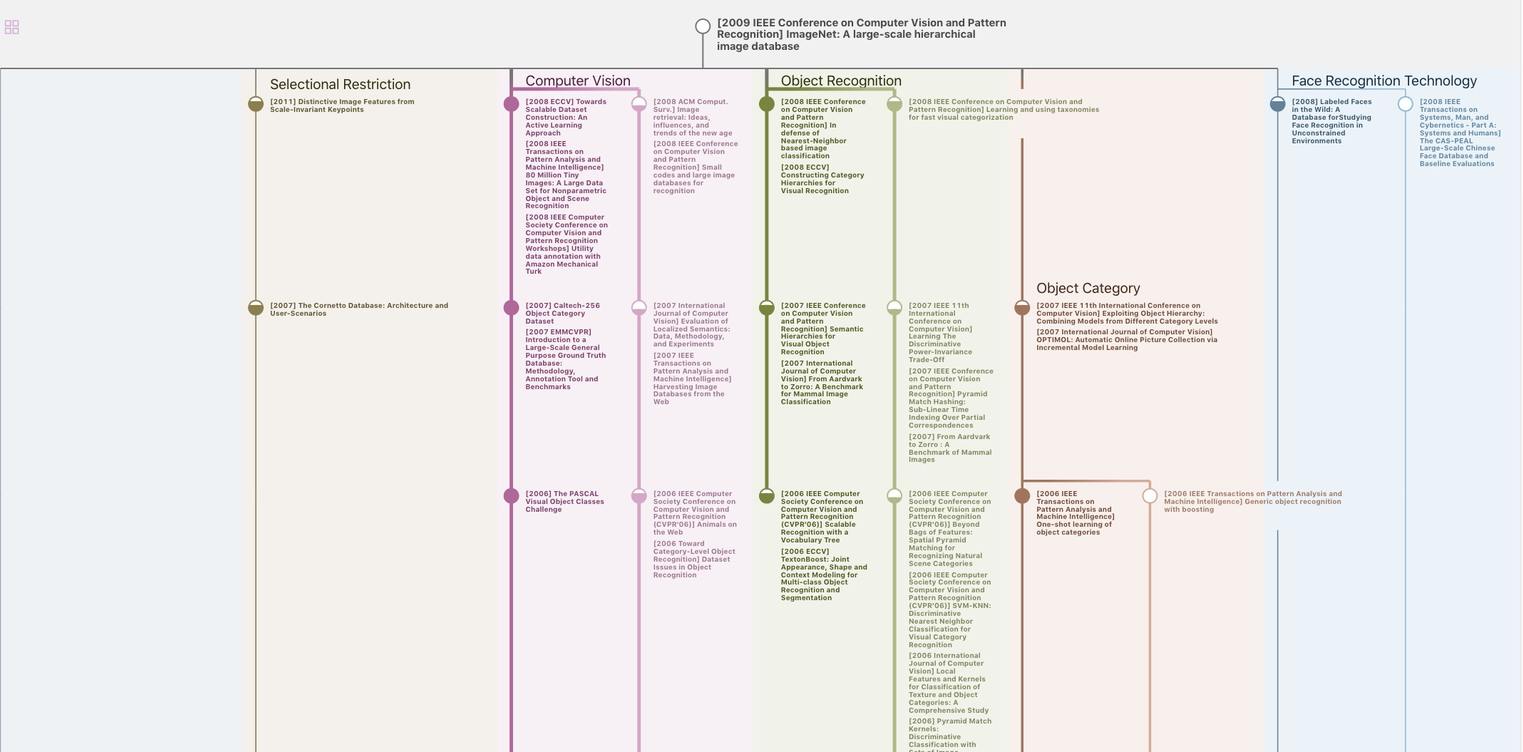
生成溯源树,研究论文发展脉络
Chat Paper
正在生成论文摘要