A localized adaptive soft sensor for dynamic system modeling
Chemical Engineering Science(2014)
摘要
A new, localized adaptive soft sensor (LASS) is presented in this paper. The algorithm addresses two issues that arise in modeling by localized adaptive RPLS (LARPLS) with a forgetting factor: (1) the soft sensors used in LARPLS and (2) the preset threshold for updating the local region of the process (re-localization). Instead of using recursive partial least squares (RPLS) with a forgetting factor in the LASS model, an RPLS algorithm is used in a moving window to provide the local learning framework for online prediction in the soft sensor. This approach enables better predictive performance from a simpler model. Adaptive thresholding for re-localization is used in the new LASS model in place of the preset threshold in LARPLS to track process dynamics more effectively. A new, averaged bias updating strategy is applied to the LASS models, to avoid the need to preselect a weighting factor. Averaging of this newly developed LASS model and a model based on LARPLS with adaptive thresholding is also examined. A comparison is made with several other global and local models, and the newly developed LASS with a moving-window is shown to be more adaptive in the face of process changes, as demonstrated in the modeling of three different chemical processes.
更多查看译文
关键词
LASS,Moving-window,Adaptive thresholding,Averaged bias updating,Averaged LASS
AI 理解论文
溯源树
样例
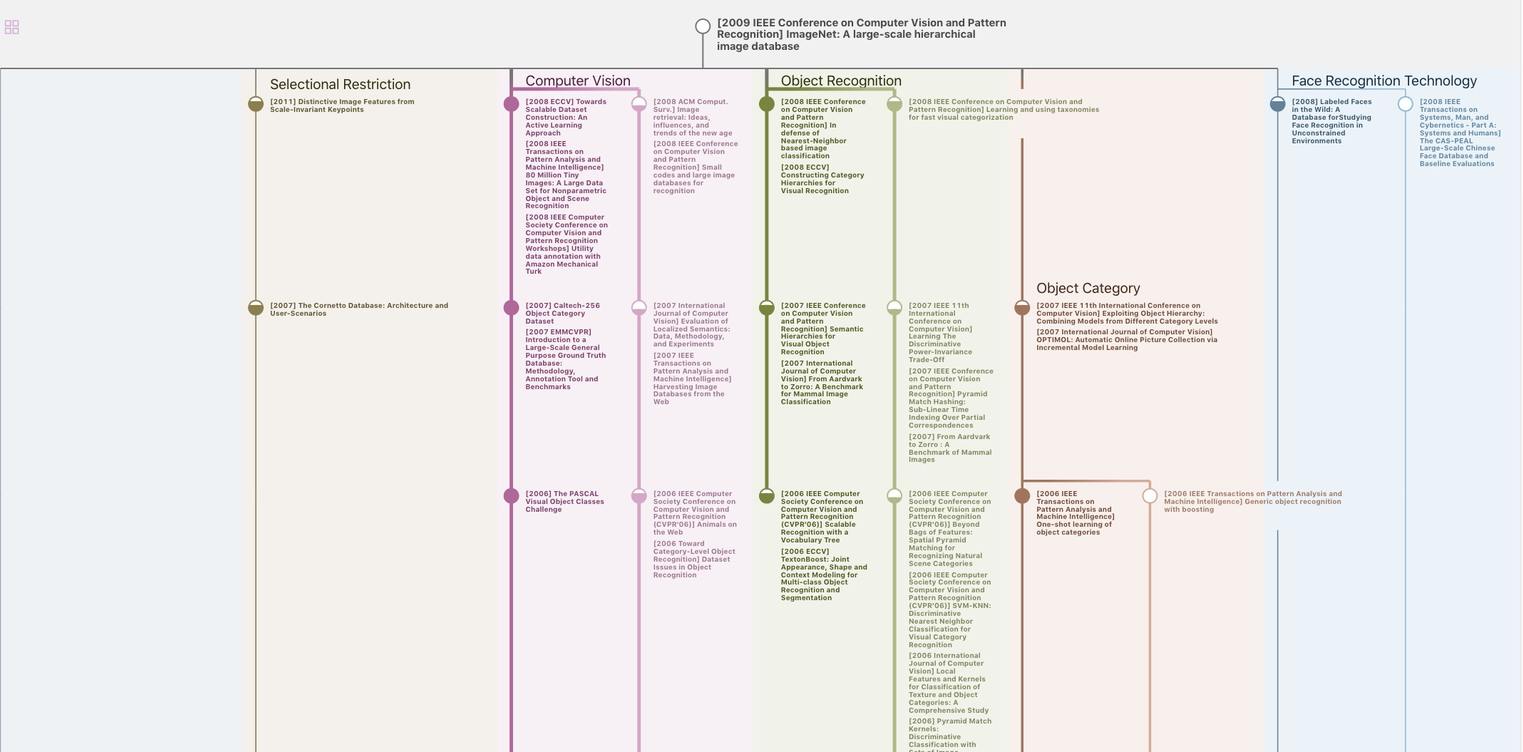
生成溯源树,研究论文发展脉络
Chat Paper
正在生成论文摘要