Adaptive Bayesian compound designs for dose finding studies
Journal of Statistical Planning and Inference(2012)
摘要
We consider the problem of how to efficiently and safely design dose finding studies. Both current and novel utility functions are explored using Bayesian adaptive design methodology for the estimation of a maximum tolerated dose (MTD). In particular, we explore widely adopted approaches such as the continual reassessment method and minimizing the variance of the estimate of an MTD. New utility functions are constructed in the Bayesian framework and are evaluated against current approaches. To reduce computing time, importance sampling is implemented to re-weight posterior samples thus avoiding the need to draw samples using Markov chain Monte Carlo techniques. Further, as such studies are generally first-in-man, the safety of patients is paramount. We therefore explore methods for the incorporation of safety considerations into utility functions to ensure that only safe and well-predicted doses are administered. The amalgamation of Bayesian methodology, adaptive design and compound utility functions is termed adaptive Bayesian compound design (ABCD). The performance of this amalgamation of methodology is investigated via the simulation of dose finding studies. The paper concludes with a discussion of results and extensions that could be included into our approach.
更多查看译文
关键词
Adaptive design,Compound utility,Importance sampling,Markov chain Monte Carlo,Optimal design,Safety,Utility functions
AI 理解论文
溯源树
样例
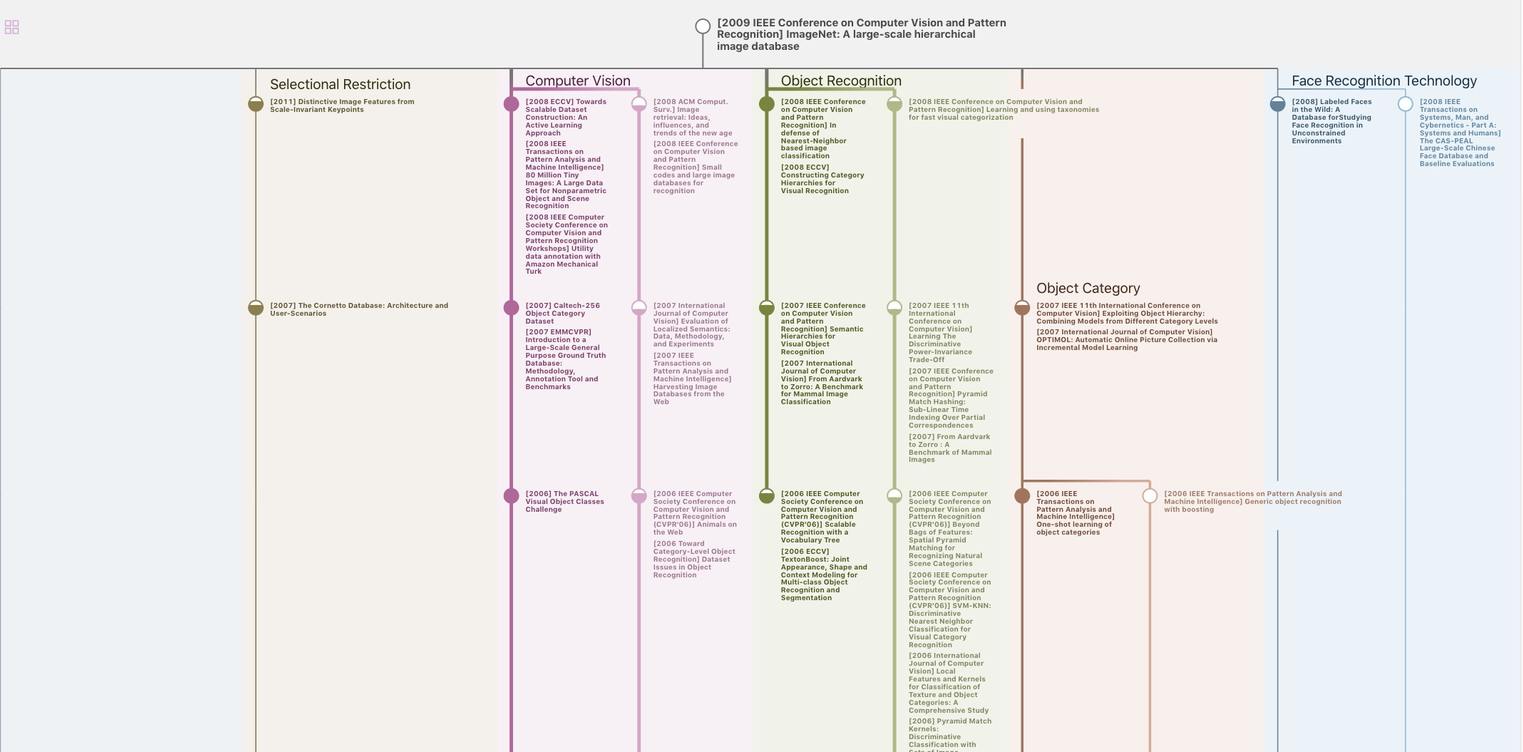
生成溯源树,研究论文发展脉络
Chat Paper
正在生成论文摘要