Estimation of the mixed logit likelihood function by randomized quasi-Monte Carlo
Transportation Research Part B: Methodological(2012)
摘要
We examine the effectiveness of randomized quasi-Monte Carlo (RQMC) techniques to estimate the integrals that express the discrete choice probabilities in a mixed logit model, for which no closed form formula is available. These models are used extensively in travel behavior research. We consider popular RQMC constructions such as randomized Sobol', Faure, and Halton points, but our main emphasis is on randomly-shifted lattice rules, for which we study how to select the parameters as a function of the considered class of integrands. We compare the effectiveness of all these methods and of standard Monte Carlo (MC) to reduce both the variance and the bias when estimating the log-likelihood function at a given parameter value. In our numerical experiments, randomized lattice rules (with carefully selected parameters) and digital nets are the best performers and they reduce the bias as much as the variance. With panel data, in our examples, the performance of all RQMC methods degrades rapidly when we simultaneously increase the dimension and the number of observations per individual. (C) 2011 Elsevier Ltd. All rights reserved.
更多查看译文
关键词
Discrete choice,Mixed logit,Randomized quasi-Monte Carlo,MSE reduction,Lattice rules
AI 理解论文
溯源树
样例
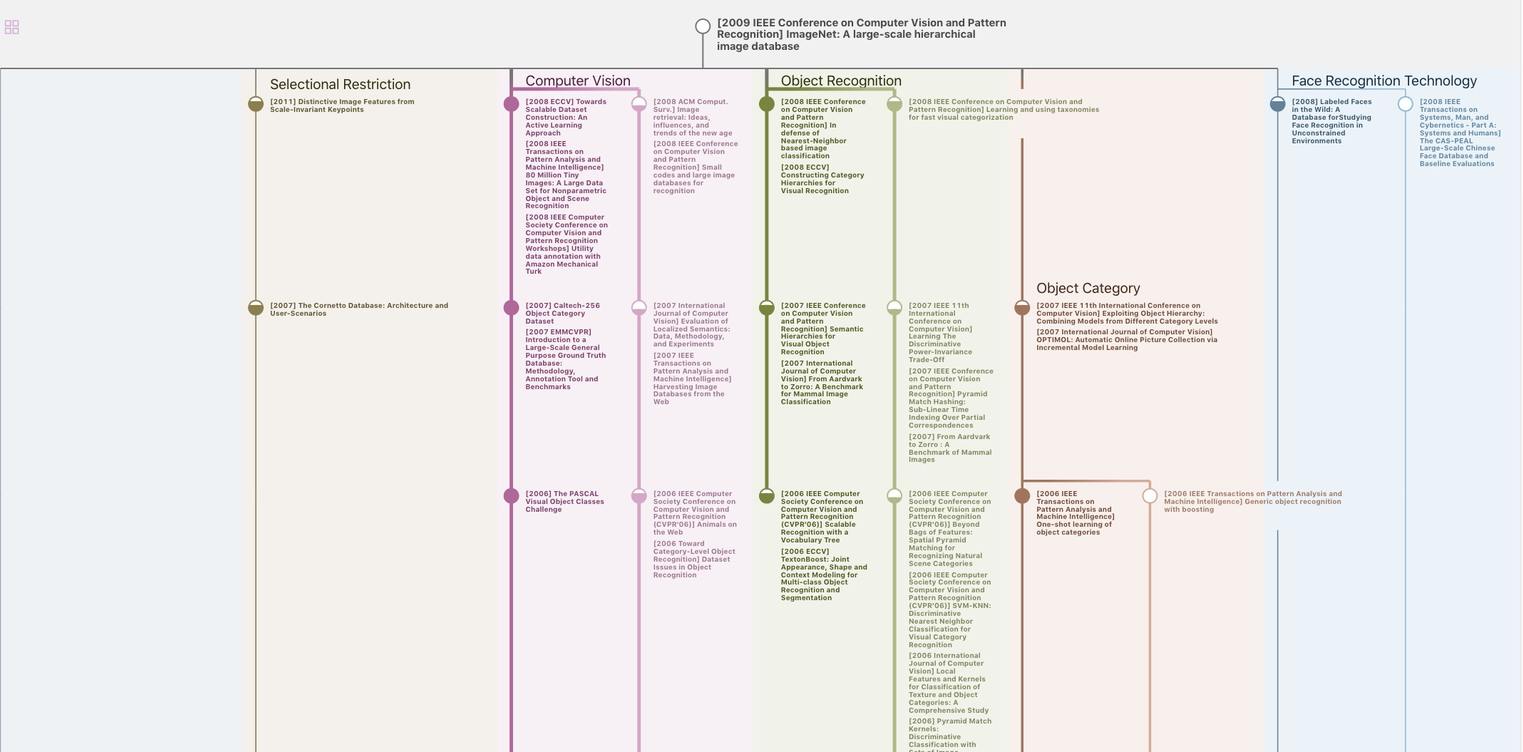
生成溯源树,研究论文发展脉络
Chat Paper
正在生成论文摘要