Bayesian Phylogenetic Inference Using a Combinatorial Sequential Monte Carlo Method
JOURNAL OF THE AMERICAN STATISTICAL ASSOCIATION(2016)
摘要
The application of Bayesian methods to large-scale phylogenetics problems is increasingly limited by computational issues, motivating the development of methods that can complement existing Markov chain Monte Carlo (MCMC) schemes. Sequential Monte Carlo (SMC) methods are approximate inference algorithms that have become very popular for time series models. Such methods have been recently developed to address phylogenetic inference problems but currently available techniques are only applicable to a restricted class of phylogenetic tree models compared to MCMC. In this article, we propose an original combinatorial SMC (CSMC) method to approximate posterior phylogenetic tree distributions, which is applicable to a general class of models and can be easily combined with MCMC to infer evolutionary parameters. Our method only relies on the existence of a flexible partially ordered set structure and is more generally applicable to sampling problems on combinatorial spaces. We demonstrate that the proposed CSMC algorithm provides consistent estimates under weak assumptions, is computationally fast, and is additionally easily parallelizable. Supplementary materials for this article are available online.
更多查看译文
关键词
Bayesian inference,Particle Markov chain Monte Carlo,Phylogenetics,Poset,Sequential Monte Carlo
AI 理解论文
溯源树
样例
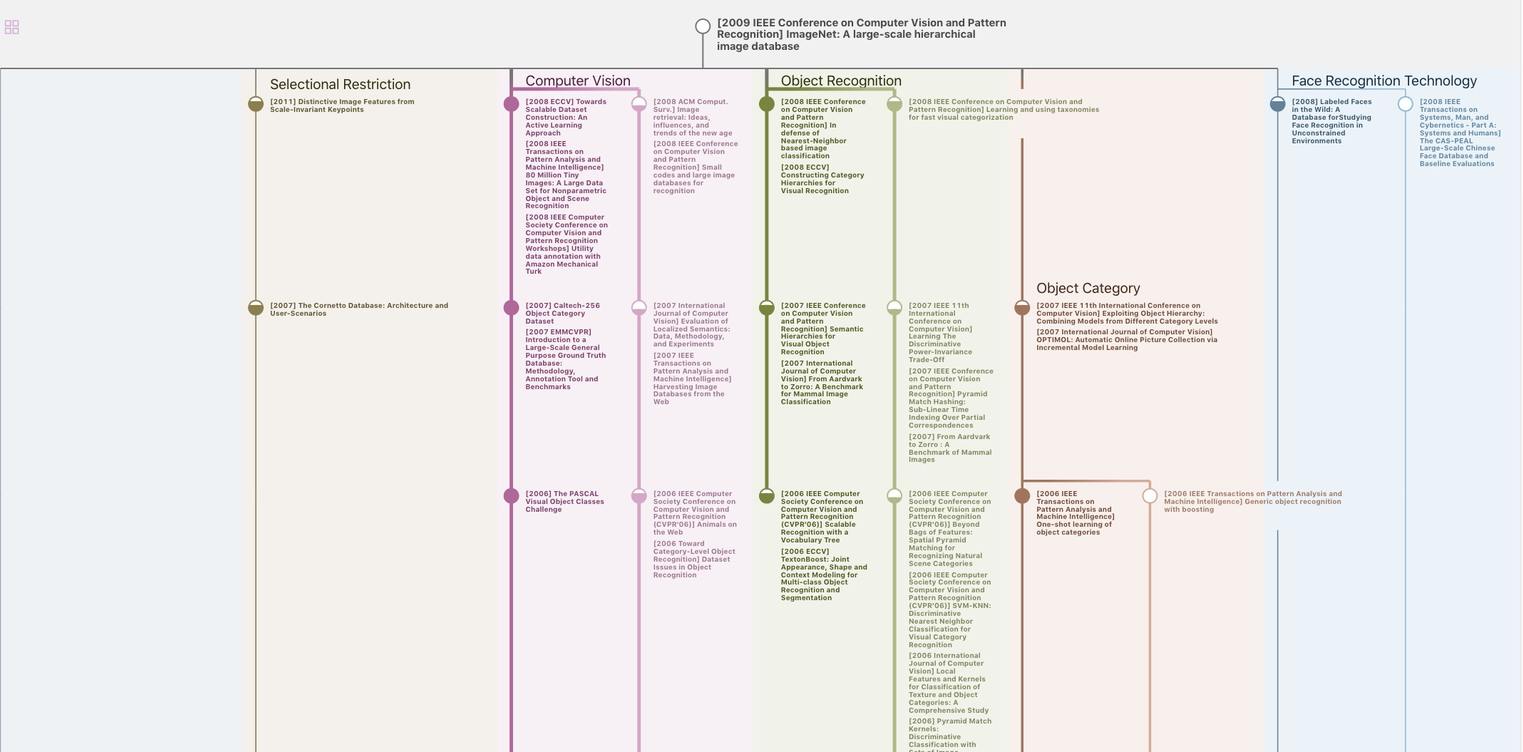
生成溯源树,研究论文发展脉络
Chat Paper
正在生成论文摘要