Approximations of the Optimal Importance Density Using Gaussian Particle Flow Importance Sampling
JOURNAL OF THE AMERICAN STATISTICAL ASSOCIATION(2016)
摘要
Recently developed particle flow algorithms provide an alternative to importance sampling for drawing particles from a posterior distribution, and a number of particle filters based on this principle have been proposed. Samples are drawn from the prior and then moved according to some dynamics over an interval of pseudo-time such that their final values are distributed according to the desired posterior. In practice, implementing a particle flow, sampler requires multiple layers of approximation, with the result that the final samples do not in general have the correct posterior distribution. In this article we consider using an approximate Gaussian flow for sampling with a class of nonlinear Gaussian models. We use the particle flow within an importance sampler, correcting for the discrepancy between the target and actual densities with importance weights. We present a suitable numerical integration procedure for use with this flow and an accompanying step-size control algorithm. In a filtering context, we use the particle flow to sample from the optimal importance density, rather than the filtering density itself, avoiding the need to make analytical or numerical approximations of the predictive density. Simulations using particle flow importance sampling within a particle filter demoristrate significant improvement over standard approximations of the optimal importance density, and the algorithm falls within the standard sequential Monte Carlo framework.
更多查看译文
关键词
Algorithms,Bayesian methods,Sampling,Signal processing,Time series
AI 理解论文
溯源树
样例
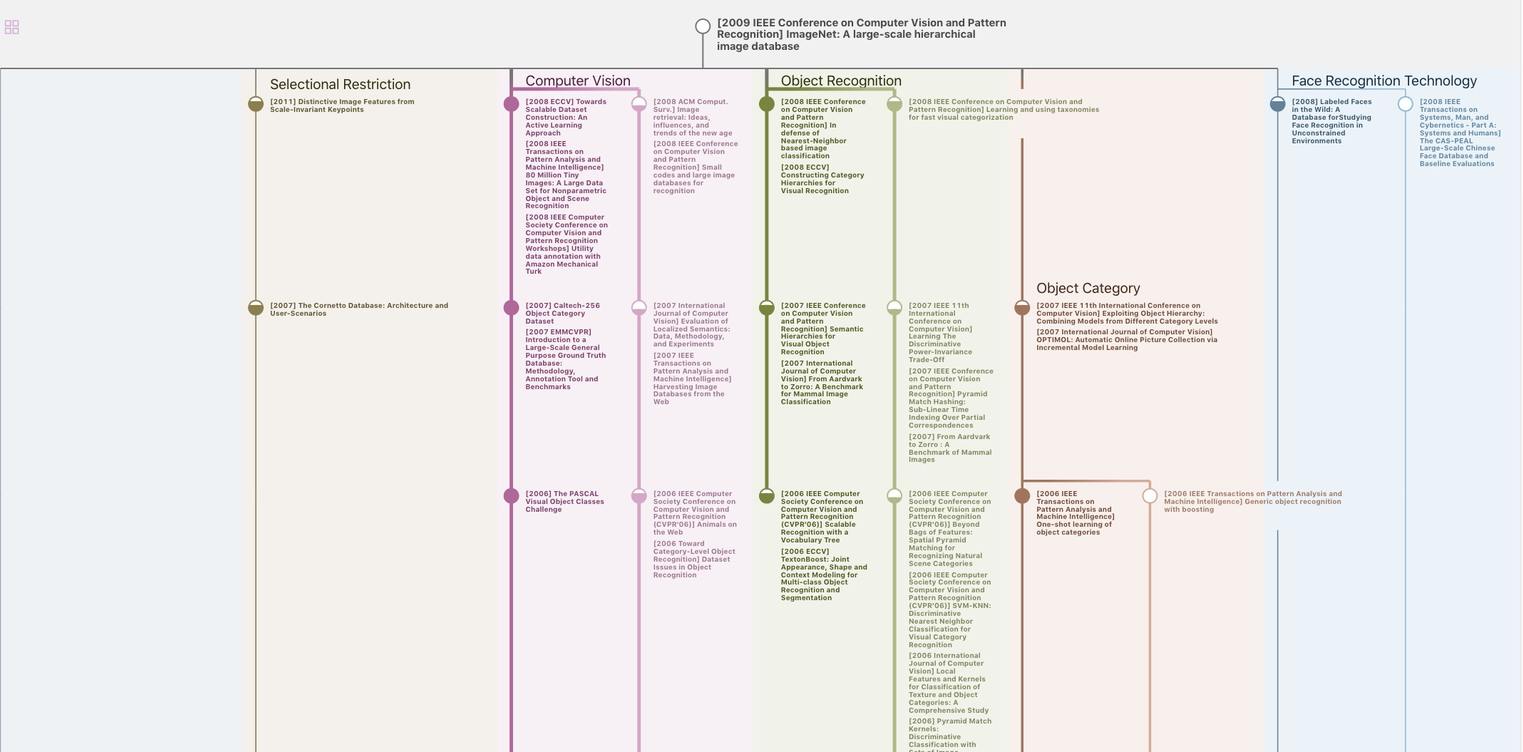
生成溯源树,研究论文发展脉络
Chat Paper
正在生成论文摘要