Bayesian approach to magnetotelluric tensor decomposition
ANNALS OF GEOPHYSICS(2010)
摘要
Magnetotelluric directional analysis and impedance tensor decomposition are basic tools to validate a local/regional composite electrical model of the underlying structure. Bayesian stochastic methods approach the problem of the parameter estimation and their uncertainty characterization in a fully probabilistic fashion, through the use of posterior model probabilities. We use the standard Groom-Bailey 3-D local/2-D regional composite model in our bayesian approach. We assume that the experimental impedance estimates are contamined with the Gaussian noise and define the likelihood of a particular composite model with respect to the observed data. We use non-informative, flat priors over physically reasonable intervals for the standard Groom-Bailey decomposition parameters. We apply two numerical methods, the Markov chain Monte Carlo procedure based on the Gibbs sampler and a single-component adaptive Metropolis algorithm. From the posterior samples, we characterize the estimates and uncertainties of the individual decomposition parameters by using the respective marginal posterior probabilities. We conclude that the stochastic scheme performs reliably for a variety of models, including the multisite and multifrequency case with up to several hundreds of parameters. Though the Monte Carlo samplers are computationally very intensive, the adaptive Metropolis algorithm increase the speed of the simulations for large-scale problems.
更多查看译文
AI 理解论文
溯源树
样例
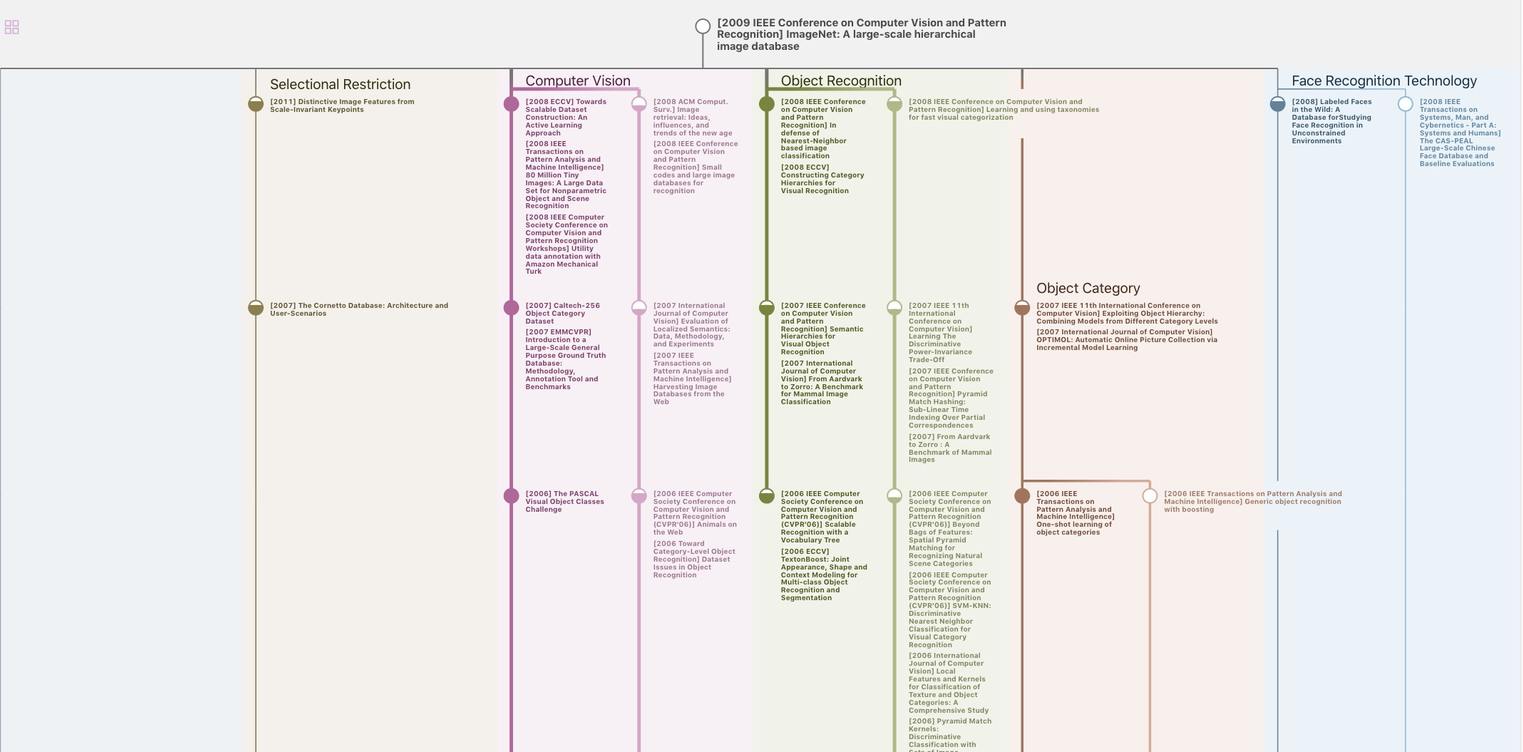
生成溯源树,研究论文发展脉络
Chat Paper
正在生成论文摘要