Can Suspended Fine-Sediment Transport in Shallow Lakes Be Predicted Using MVRVM with Limited Observations?
JOURNAL OF ENVIRONMENTAL ENGINEERING(2016)
摘要
The study of sediment transport in water natural bodies is a challenging task. There have been several attempts to describe sediment mathematically using hydraulic characteristics of water bodies. Most researchers who developed empirical formulas to describe sediment transport performed laboratory experiments with assumptions that did not take into account variations of hydraulic parameters and the fine sediment sizes that are part of this phenomenon. Recently, new approaches for studying sediment transport have been developed involving the use of machine-learning algorithms that have proven accuracy and efficiency in predicting sediment transport. A novel machine-learning method, the Multivariate Relevance Vector Machine (MVRVM), has yet to be tested to model sediment transport and water quality in estuaries and lakes. The selection of the MVRVM is suggested by the limited field observations that present challenges for alternative statistical learning machines, and by the promise of using the MVRVM approach to inform future data-collection efforts. This paper tests the success of calibrating the MVRVM model to predict suspended fine-sediment transport and other environmental measures in Mud Lake, southeastern Idaho, United States. In addition, the authors introduce and explain the technique that can be used to arrange the data which will allow the model to work. Training and validation results for turbidity, total suspended solids (TSS), pH, dissolved oxygen (DO), and water temperature are presented. These results emphasize that modeling the water-quality constituents and sediment transport with few observations is possible using the MVRVM. (C) 2015 American Society of Civil Engineers.
更多查看译文
关键词
Lakes,Shallow water,Water supply systems,Hydraulics,Water quality,Sediment transport,Hydrologic models,Sediment,Idaho,United States
AI 理解论文
溯源树
样例
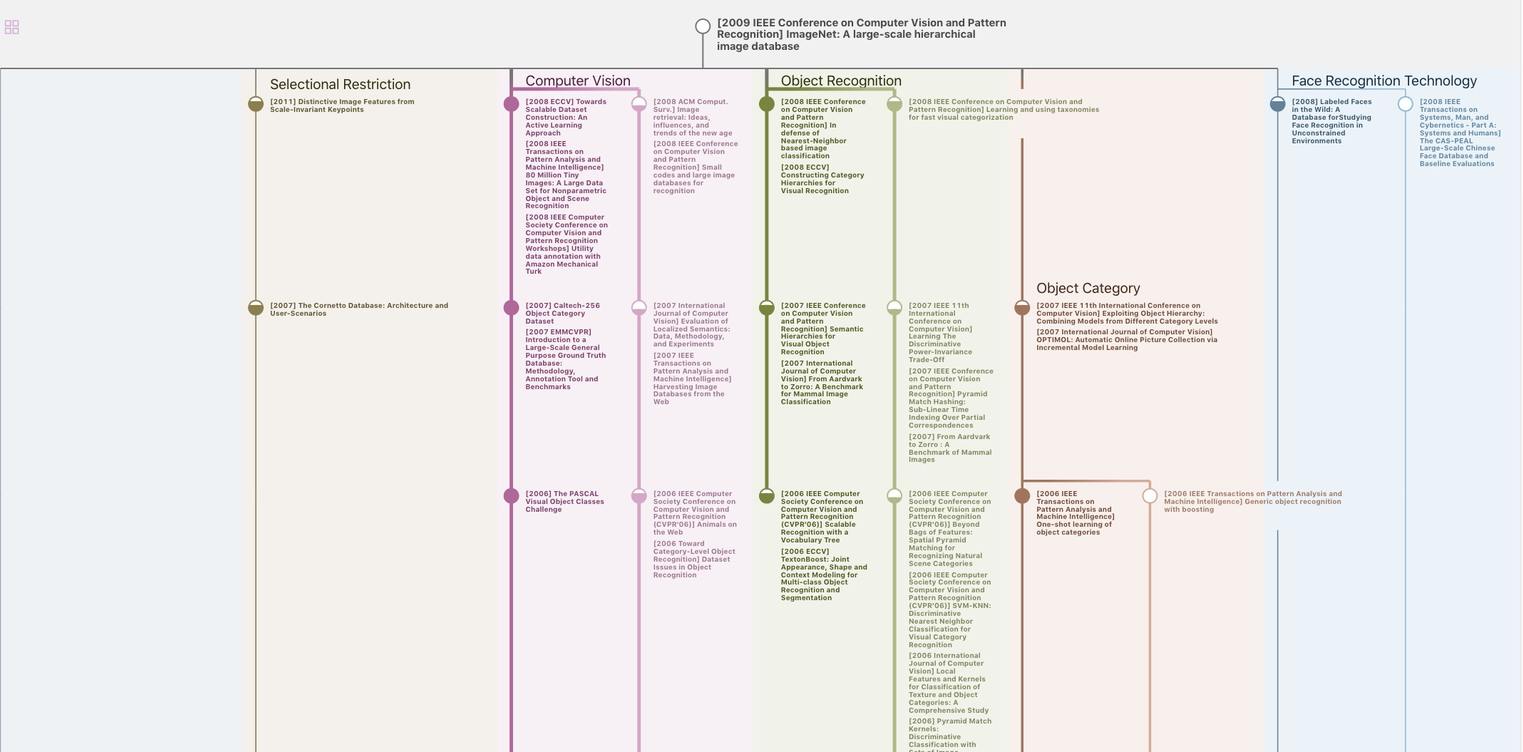
生成溯源树,研究论文发展脉络
Chat Paper
正在生成论文摘要