STING: Self-attention Based Time-series Imputation Networks Using GAN.
2021 IEEE International Conference on Data Mining (ICDM)(2021)
摘要
Time series data are ubiquitous in real-world applications. However, one of the most common problems is that the time series could have missing values by the inherent nature of the data collection process. So imputing missing values from multivariate (correlated) time series is imperative to improve a prediction performance while making an accurate data-driven decision. Conventional works for imputation simply delete missing values or fill them based on mean/zero. Although recent works based on deep neural networks have shown remarkable results, they still have a limitation to capture the complex generation process of multivariate time series. In this paper, we propose a novel imputation method for multivariate time series, called STING (Self-attention based Time-series Imputation Networks using GAN). We take advantage of generative adversarial networks and bidirectional recurrent neural networks to learn the latent representations of time series. In addition, we introduce a novel attention mechanism to capture the weighted correlations of a whole sequence and avoid the potential bias brought by unrelated ones. The experimental results on three real-world datasets demonstrate that STING outperforms the existing state-of-the-art methods in terms of imputation accuracy as well as downstream tasks with the imputed values therein.
更多查看译文
关键词
time-series imputation,self-attention,generative adversarial networks,bidirectional RNN
AI 理解论文
溯源树
样例
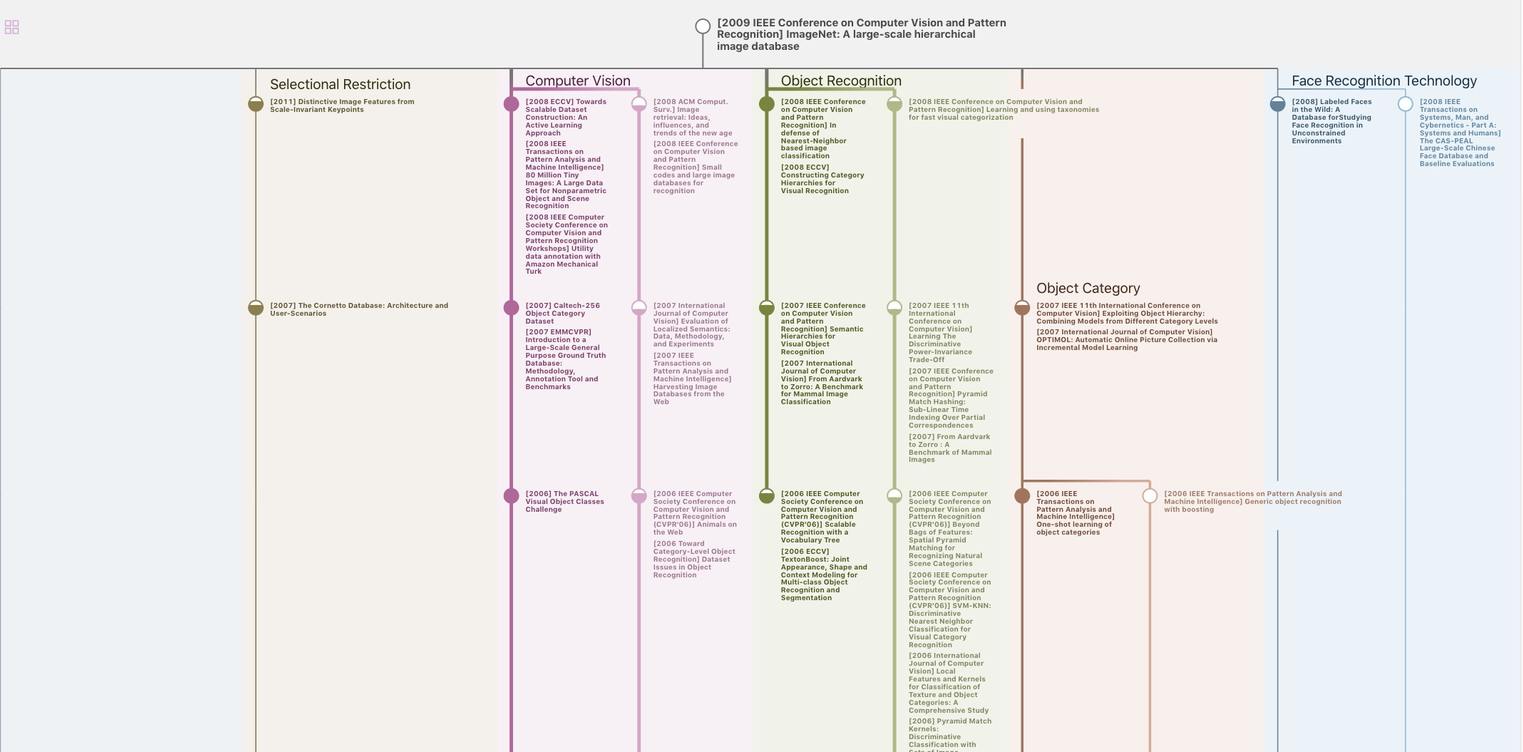
生成溯源树,研究论文发展脉络
Chat Paper
正在生成论文摘要