Parallel geometric classification of stem cells by their three-dimensional morphology
Computational Science & Discovery(2013)
摘要
There is a need for tools to classify cells based on their three-dimensional (3D) shape. Cells exist in vivo in 3D, cells are frequently cultured within 3D scaffolds in vitro and 3D scaffolds are used for cell delivery in tissue engineering therapies. Recent work indicates that the physical structure of a tissue engineering scaffold can direct stem cell function by driving stem cells into morphologies that induce their differentiation. Thus, we have developed a rapid method for classifying cells based on their 3D shape. First, random lines are intersected with 3D Z-stacks of confocal images of stem cells. The intersection lengths are stored in histograms, which are then used to train a support vector machine (SVM) learning algorithm to distinguish between stem cells cultured on differentiation-inducing 3D scaffolds and those cultured on non-differentiating flat substrates. The trained SVM is able to properly classify the ?new? query cells over 80% of the time. The algorithm is easily parallelizable and we demonstrate its implementation on a commodity graphics processing unit (GPU). Use of a GPU to run the algorithm increases throughput by over 100-fold as compared to use of a CPU. The algorithm is also progressive, providing an approximate answer quickly and refining the answer over time. This allows further increase in the throughput of the algorithm by allowing the SVM classification scheme to terminate early if it becomes confident enough of the class of the cell being analyzed. These results demonstrate a rapid method for classifying stem cells based on their 3D shape that can be used by tissue engineers for identifying 3D tissue scaffold structures that drive stem cells into shapes that correlate with differentiation.
更多查看译文
AI 理解论文
溯源树
样例
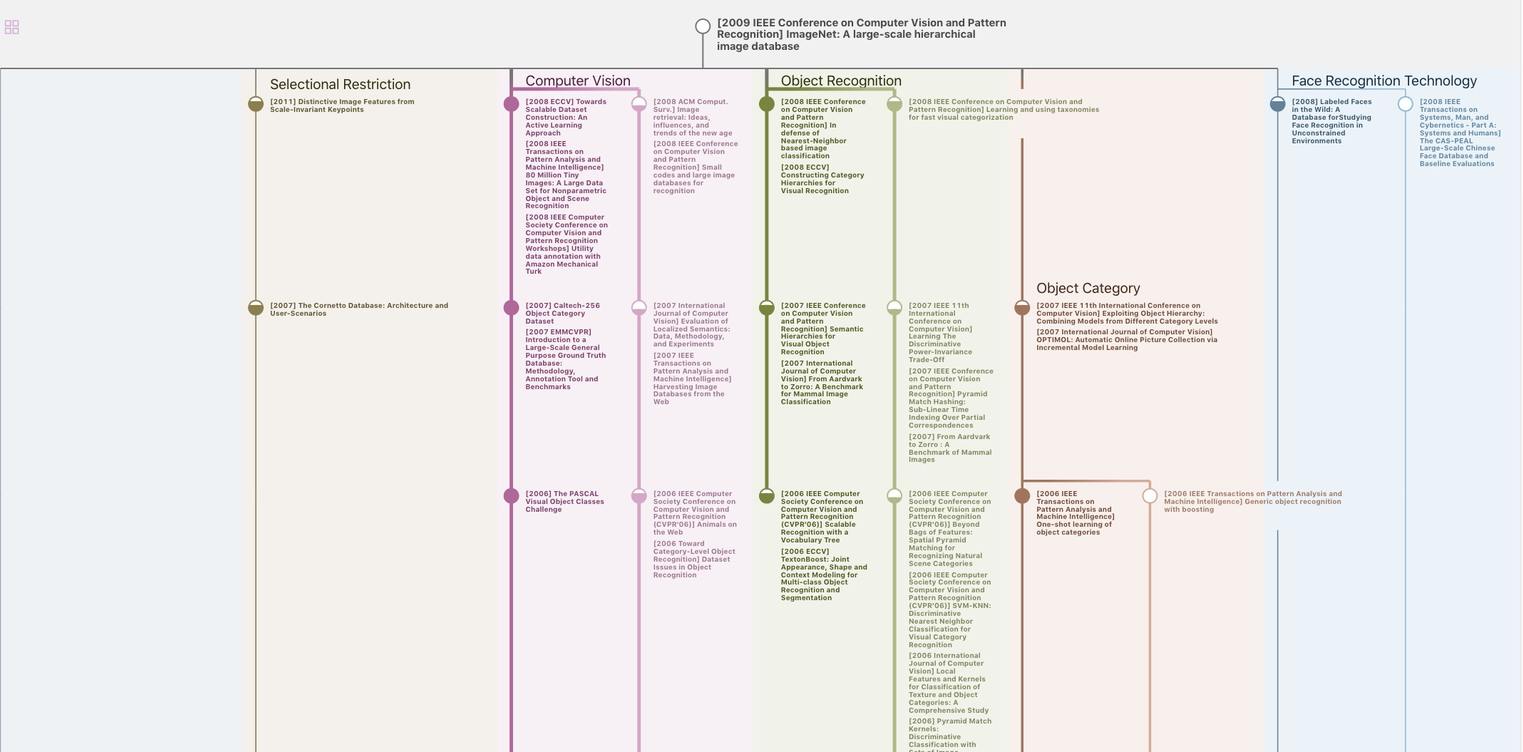
生成溯源树,研究论文发展脉络
Chat Paper
正在生成论文摘要