NONUNIFORM SPARSE RECOVERY WITH SUBGAUSSIAN MATRICES
ELECTRONIC TRANSACTIONS ON NUMERICAL ANALYSIS(2014)
摘要
Compressive sensing predicts that sufficiently sparse vectors can be recovered from highly incomplete information using efficient recovery methods such as l(1)-minimization. Random matrices have become a popular choice for the measurement matrix. Indeed, near-optimal uniform recovery results have been shown for such matrices. In this note we focus on nonuniform recovery using subgaussian random matrices and l(1)-minimization. We provide conditions on the number of samples in terms of the sparsity and the signal length which guarantee that a fixed sparse signal can be recovered with a random draw of the matrix using l(1)-minimization. Our proofs are short and provide explicit and convenient constants.
更多查看译文
关键词
compressed sensing,sparse recovery,random matrices,l(1)-minimization
AI 理解论文
溯源树
样例
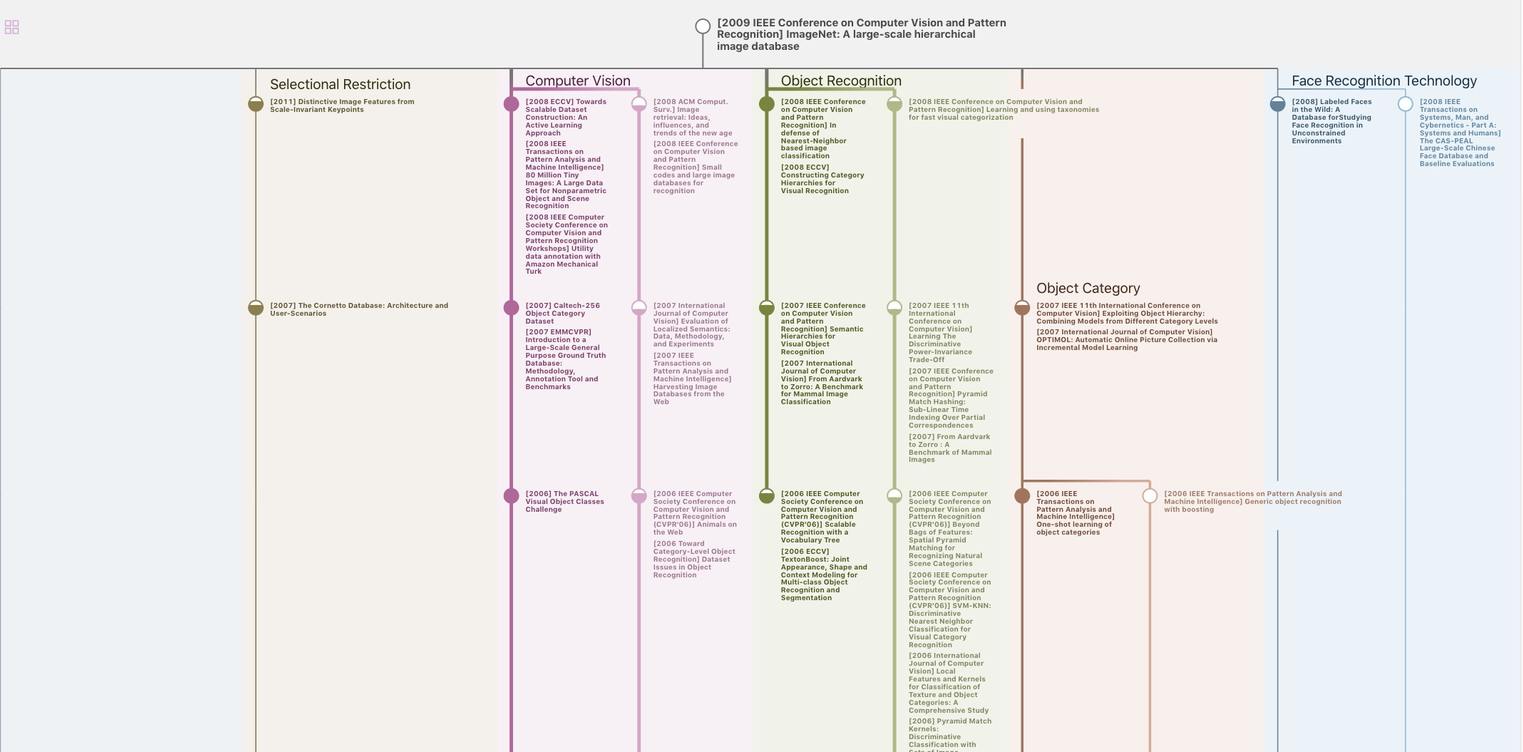
生成溯源树,研究论文发展脉络
Chat Paper
正在生成论文摘要