Graphical Representation of Missing Data Problems
STRUCTURAL EQUATION MODELING-A MULTIDISCIPLINARY JOURNAL(2015)
摘要
Rubin's classic missingness mechanisms are central to handling missing data and minimizing biases that can arise due to missingness. However, the formulaic expressions that posit certain independencies among missing and observed data are difficult to grasp. As a result, applied researchers often rely on informal translations of these assumptions. We present a graphical representation of missing data mechanism, formalized in Mohan, Pearl, and Tian (2013). We show that graphical models provide a tool for comprehending, encoding, and communicating assumptions about the missingness process. Furthermore, we demonstrate on several examples how graph-theoretical criteria can determine if biases due to missing data might emerge in some estimates of interests and which auxiliary variables are needed to control for such biases, given assumptions about the missingness process.
更多查看译文
关键词
auxiliary variables,full information,graphical models,maximum likelihood,missing data,multiple imputation
AI 理解论文
溯源树
样例
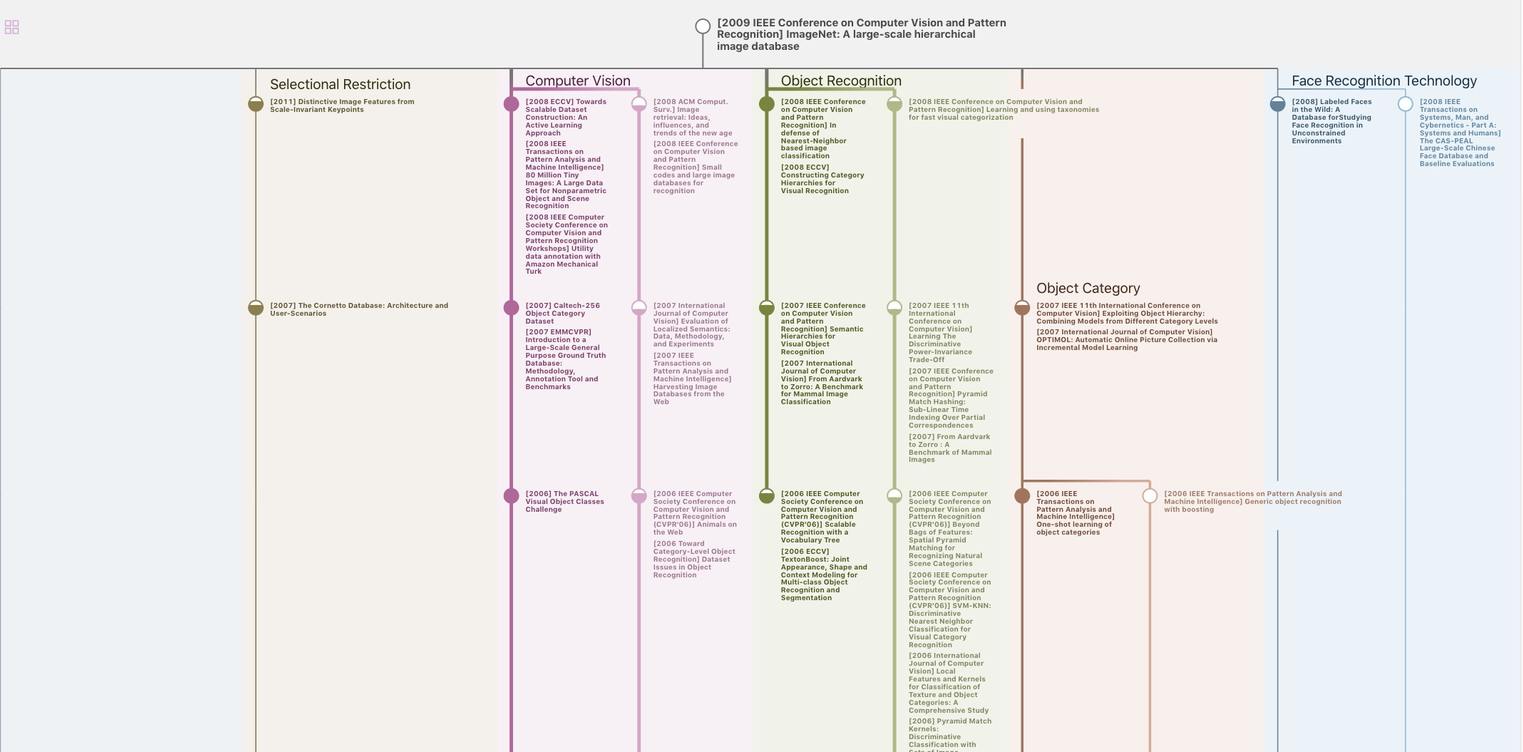
生成溯源树,研究论文发展脉络
Chat Paper
正在生成论文摘要