Kernel-based mixture models for classification
Computational Statistics(2014)
摘要
A generative model for classification based on kernels and mixtures of univariate Gamma distributions is introduced. It models the point distances to cluster centroids in the transformed Hilbert space associated with the inner product induced by the kernel. The distances are readily computed using the kernel trick. Nested within this kernel-based Gamma mixture model (KMM) are two special cases corresponding to the kernel-based mixture of exponentials and the kernel-based mixture of spherical Gaussians. The Akaike information criterion is used to select an appropriate parsimonious type-of-mixture model for the data at hand. A powerful classification rule based on the knowledge of all point distances to every class centroid is developed based on this model. The flexibility in the choice of the kernel and the probabilistic nature of a mixture distribution makes KMM appealing for modeling and inference. A comparison with other popular classification methods shows that this model is very efficient when handling high dimensional data.
更多查看译文
关键词
Machine learning,Supervised classification,Discriminant analysis,Model selection
AI 理解论文
溯源树
样例
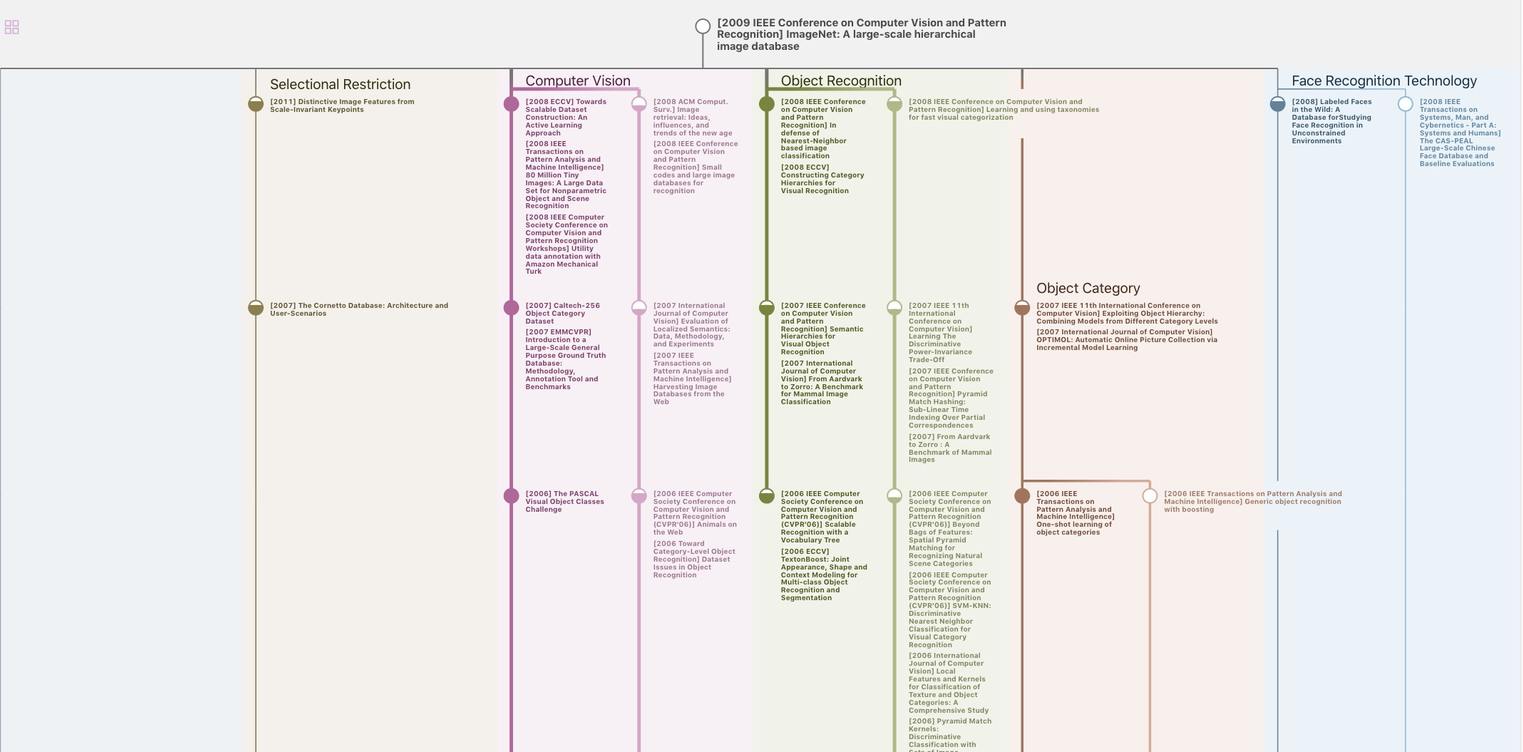
生成溯源树,研究论文发展脉络
Chat Paper
正在生成论文摘要