A Sample Selection Model with Skew‐normal Distribution
SCANDINAVIAN JOURNAL OF STATISTICS(2016)
摘要
Non-random sampling is a source of bias in empirical research. It is common for the outcomes of interest (e.g.wage distribution) to be skewed in the source population. Sometimes, the outcomes are further subjected to sample selection, which is a type of missing data, resulting in partial observability. Thus, methods based on complete cases for skew data are inadequate for the analysis of such data and a general sample selection model is required. Heckman proposed a full maximum likelihood estimation method under the normality assumption for sample selection problems, and parametric and non-parametric extensions have been proposed. We generalize Heckman selection model to allow for underlying skew-normal distributions. Finite-sample performance of the maximum likelihood estimator of the model is studied via simulation. Applications illustrate the strength of the model in capturing spurious skewness in bounded scores, and in modelling data where logarithm transformation could not mitigate the effect of inherent skewness in the outcome variable.
更多查看译文
关键词
generalized skew-normal distribution,missing data,non-random sample,sample selection
AI 理解论文
溯源树
样例
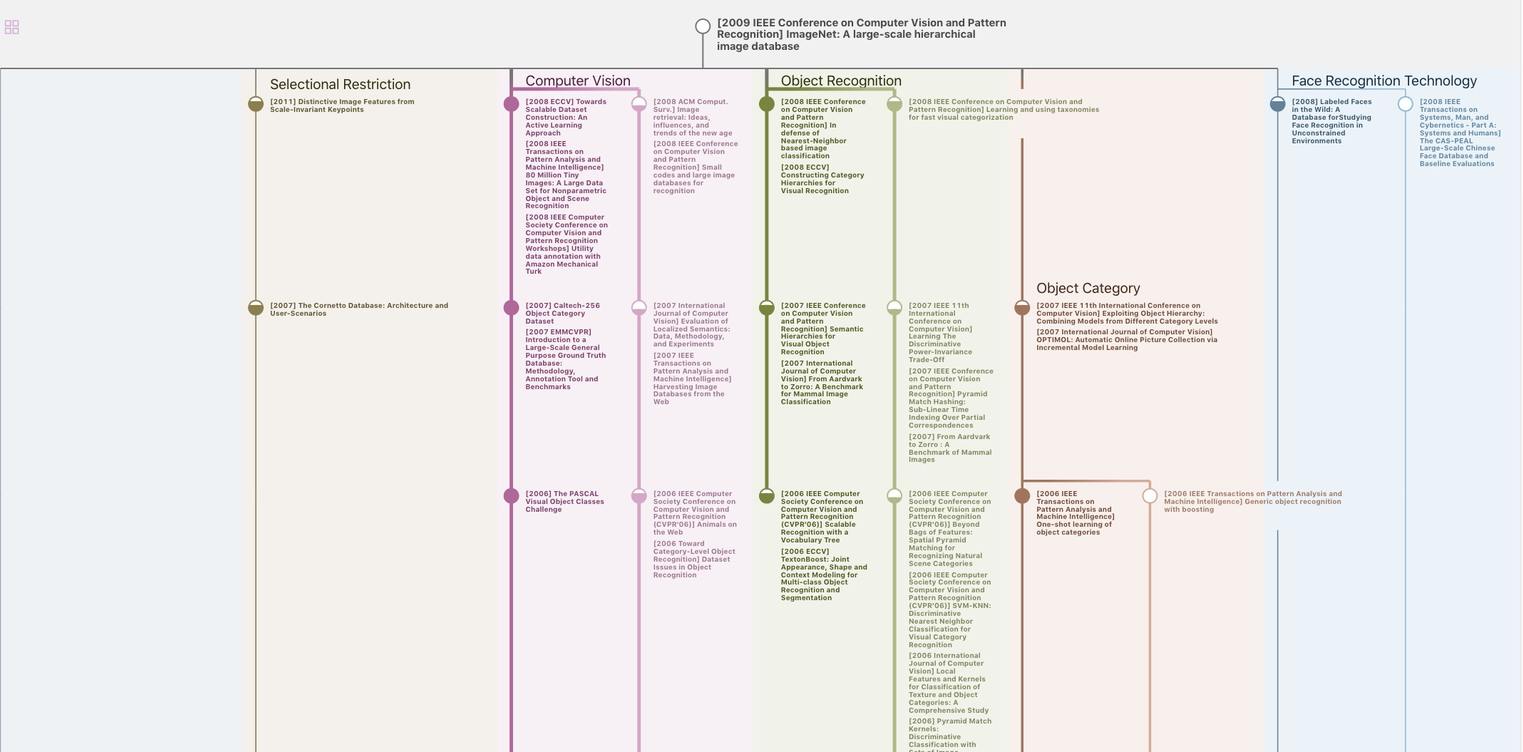
生成溯源树,研究论文发展脉络
Chat Paper
正在生成论文摘要