MP39-20 ALEATORIC LARGE DATASET ANALYSIS FRAMEWORK: A PRACTICAL APPROACH TO MINING PUBLIC EXPRESSION DATA FOR CANCER RESEARCHERS
The Journal of urology/The journal of urology(2014)
摘要
You have accessJournal of UrologyBladder Cancer: Basic Research IV1 Apr 2014MP39-20 ALEATORIC LARGE DATASET ANALYSIS FRAMEWORK: A PRACTICAL APPROACH TO MINING PUBLIC EXPRESSION DATA FOR CANCER RESEARCHERS Jonathan A. Ewald, Howard H. Bailey, William A. Ricke, and Tracy M. Downs Jonathan A. EwaldJonathan A. Ewald More articles by this author , Howard H. BaileyHoward H. Bailey More articles by this author , William A. RickeWilliam A. Ricke More articles by this author , and Tracy M. DownsTracy M. Downs More articles by this author View All Author Informationhttps://doi.org/10.1016/j.juro.2014.02.1335AboutPDF ToolsAdd to favoritesDownload CitationsTrack CitationsPermissionsReprints ShareFacebookTwitterLinked InEmail INTRODUCTION AND OBJECTIVES Public archives of expression microarray data provide a powerful resource for cancer research that can save valuable resources by allowing existing data to be re-purposed. These results from previous and current studies include data from in vitro experiments and analyses of clinical patient-derived tissues, including tumors. The availability of these data to the at-large cancer research community can promote the development of innovative ideas that otherwise may not occur to a professional data analyst. However, many investigators avoid this resource due to a perception that these data are inaccessible and too big for a cancer biologist or clinician to manage and interrogate effectively. Recent advances in bioinformatics software development have produced open-access tools more friendly to use by the general population. Our objective was to develop a practical, versatile workflow to guide cancer researchers’ use of public expression microarray data for hypothesis development and validation. METHODS In our experiences exploring and interrogating this resource, we have developed a simple, intuitive framework largely through trial-and-error to guide our selection, comparison, and analysis. RESULTS This approach, called ALDAF (Aleatoric Large Dataset Analysis Framework), provides a rules-based needs-driven strategy that is adjustable and adaptable to diverse research goals. This includes: (1) Exploring and Identifying Datasets; (2) Calculating Differences; (3) Selecting Data: Top-Down; (4) Selecting Data: Bottom-Up; (5) Bioinformatics Analysis and Interpretation; and (6) Validation of Selected Data. Data is available through the NCBI Gene Expression Omnibus (GEO), which can be searched according to keywords to identify applicable data. To compile, compare, identify and select data, we use Microsoft Excel to automate many of these tasks using formulas that simplify and accelerate this process. Resulting lists of genes can then be analyzed and interpreted using open-access web-based bioinformatics programs including WebGestalt, DAVID, Chilibot, and PubGene/Coremine. Gene lists can also be verified by iterative analysis of additional independent datasets to further select genes of interest, or validated by novel original experiments. CONCLUSIONS We conclude that ALDAF provides a simple, intuitive and practical means for cancer researchers to utilize public expression microarray data. © 2014FiguresReferencesRelatedDetails Volume 191 Issue 4S April 2014 Page: e433 Advertisement Copyright & Permissions© 2014Metrics Author Information Jonathan A. Ewald More articles by this author Howard H. Bailey More articles by this author William A. Ricke More articles by this author Tracy M. Downs More articles by this author Expand All Advertisement Advertisement PDF downloadLoading ...
更多查看译文
AI 理解论文
溯源树
样例
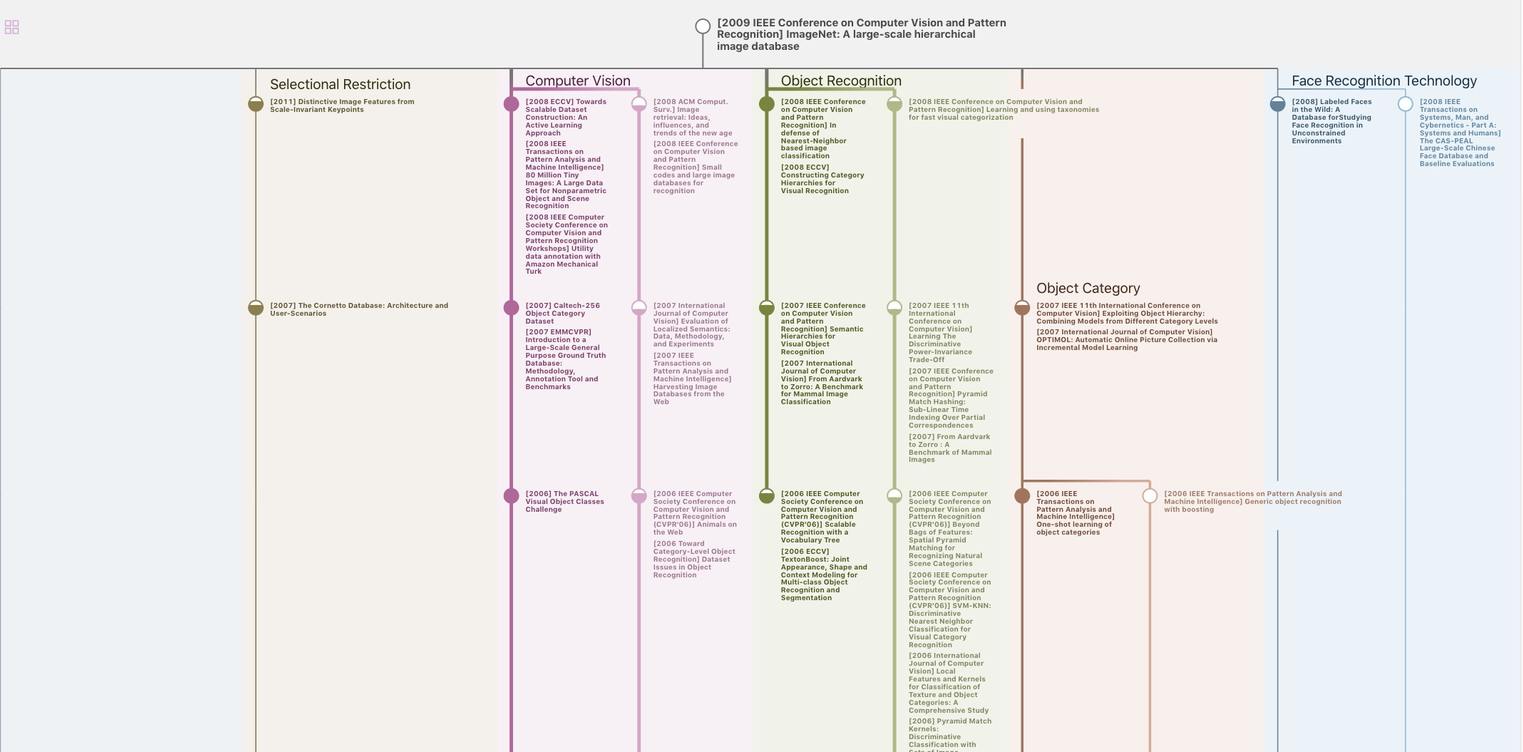
生成溯源树,研究论文发展脉络
Chat Paper
正在生成论文摘要