Stochastic identification of malware with dynamic traces
ANNALS OF APPLIED STATISTICS(2014)
摘要
A novel approach to malware classification is introduced based on analysis of instruction traces that are collected dynamically from the program in question. The method has been implemented online in a sandbox environment (i.e., a security mechanism for separating running programs) at Los Alamos National Laboratory, and is intended for eventual host-based use, provided the issue of sampling the instructions executed by a given process without disruption to the user can be satisfactorily addressed. The procedure represents an instruction trace with a Markov chain structure in which the transition matrix, P, has rows modeled as Dirichlet vectors. The malware class (malicious or benign) is modeled using a flexible spline logistic regression model with variable selection on the elements of P, which are observed with error. The utility of the method is illustrated on a sample of traces from malware and nonmalware programs, and the results are compared to other leading detection schemes (both signature and classification based). This article also has supplementary materials available online.
更多查看译文
关键词
Malware detection,classification,elastic net,Relaxed Lasso,Adaptive Lasso,logistic regression,splines,empirical Bayes
AI 理解论文
溯源树
样例
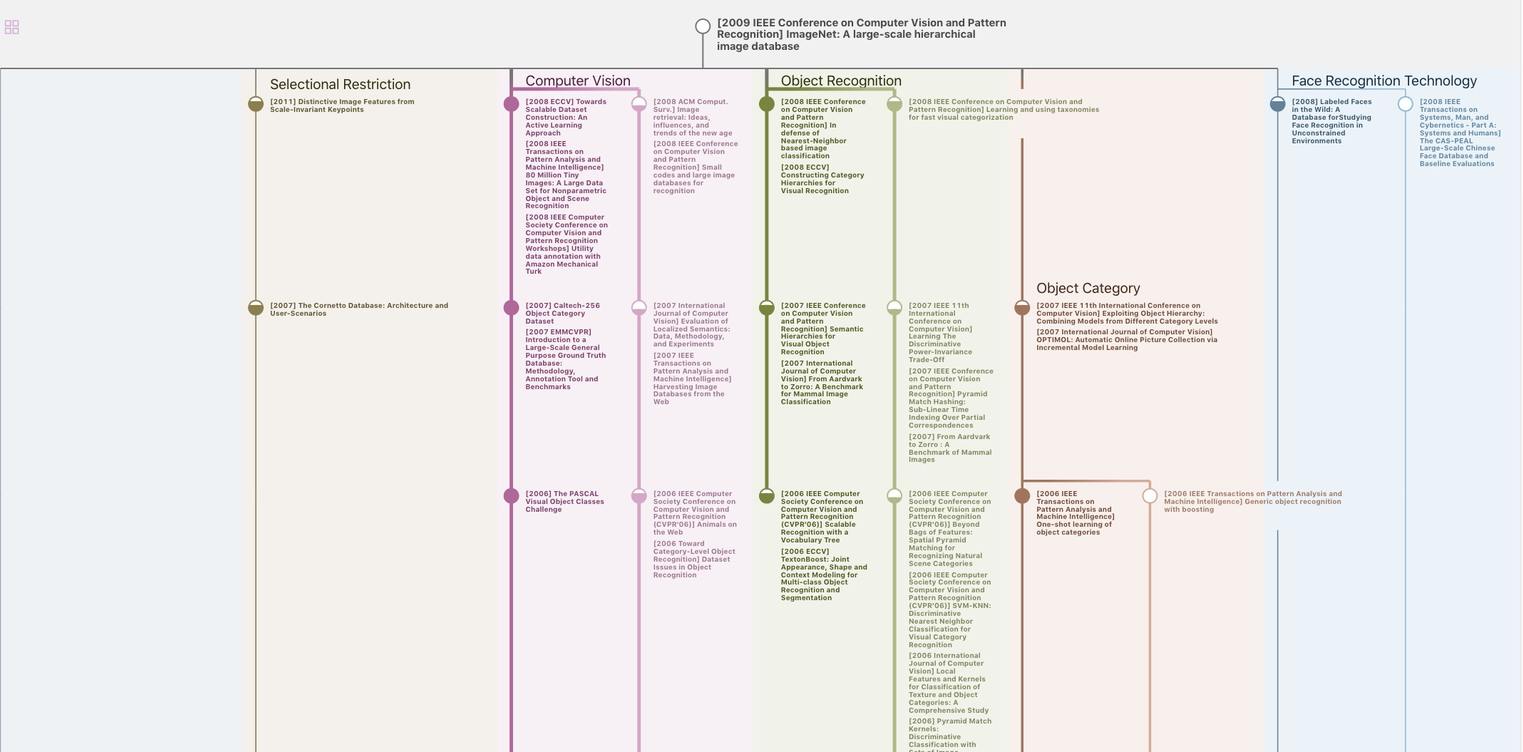
生成溯源树,研究论文发展脉络
Chat Paper
正在生成论文摘要