Adaptive LASSO for linear regression models with ARMA-GARCH errors
COMMUNICATIONS IN STATISTICS-SIMULATION AND COMPUTATION(2017)
摘要
The linear regression models with the autoregressive moving average (ARMA) errors (REGARMA models) are often considered, in order to reflect a serial correlation among observations. In this article, we focus on an adaptive least absolute shrinkage and selection operator (LASSO) (ALASSO) method for the variable selection of the REGARMA models and extend it to the linear regression models with the ARMA-generalized autoregressive conditional heteroskedasticity (ARMA-GARCH) errors (REGARMA-GARCH models). This attempt is an extension of the existing ALASSO method for the linear regression models with the AR errors (REGAR models) proposed by Wang etal. in 2007. New ALASSO algorithms are proposed to determine important predictors for the REGARMA and REGARMA-GARCH models. Finally, we provide the simulation results and real data analysis to illustrate our findings.
更多查看译文
关键词
Adaptive LASSO,ARMA error models,ARMA-GARCH error models,Penalized regression,Variable selection,62J05,62J07,62F10
AI 理解论文
溯源树
样例
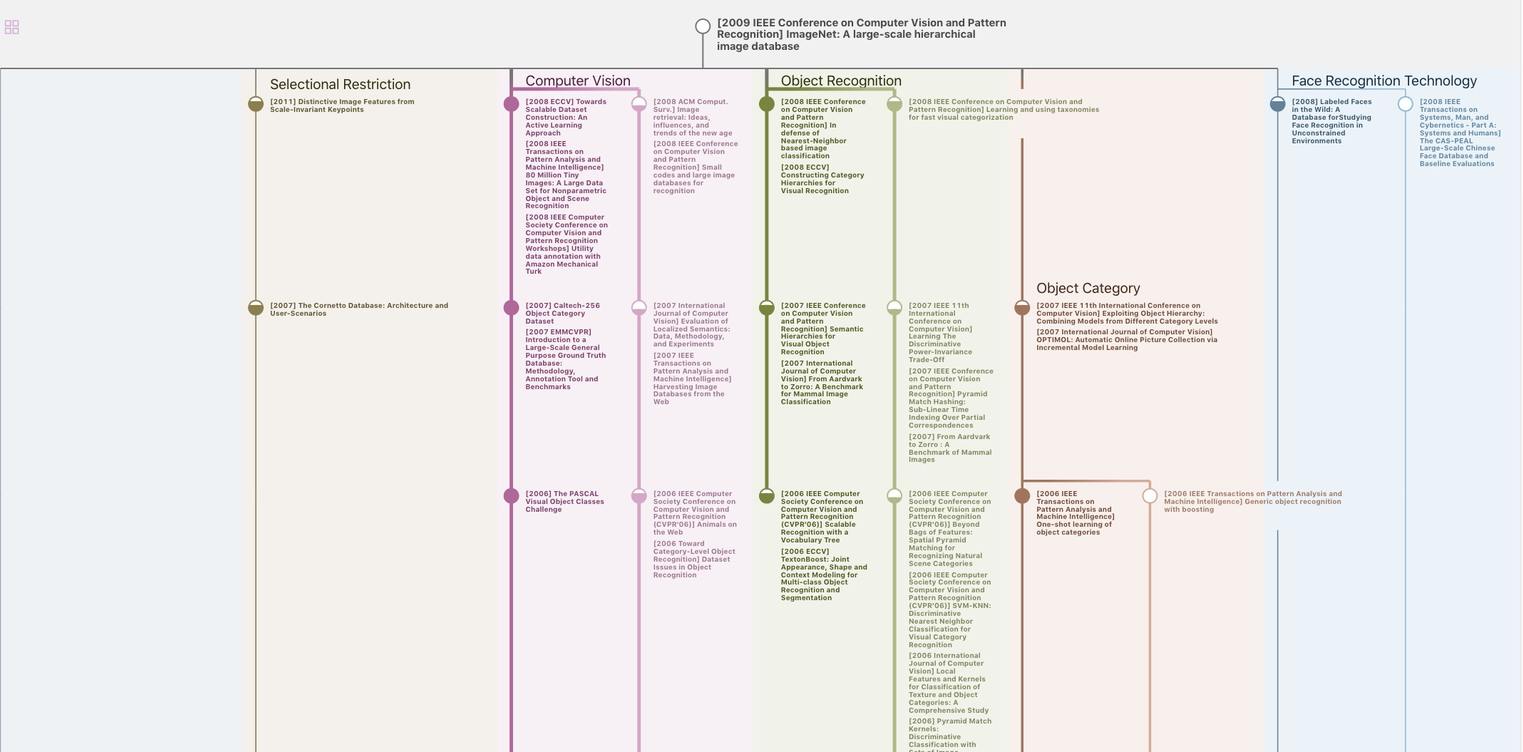
生成溯源树,研究论文发展脉络
Chat Paper
正在生成论文摘要