Heteroscedastic modelling via the autoregressive conditional variance subspace
CANADIAN JOURNAL OF STATISTICS-REVUE CANADIENNE DE STATISTIQUE(2014)
摘要
The paper deals with nonparametric estimation of the conditional variance of a time series based on a nonlinear autoregressive model in the squared innovation time series, which does not require specification of a model. We introduce a notion called the autoregressive central variance subspace (ACVS) to obtain the information included in the conditional variance of time series data. We use the squared time series to identify the ACVS by a nonparametric kernel method. In addition, we simultaneously estimate the unknown dimension and lag of the ACVS by a modified information criterion. Finally, we investigate the performance of all the estimators including the ACVS through simulations and a real analysis, which suggests implementing a new dimension reduction approach to modelling time series data that exhibits volatility. (C) 2014 Statistical Society of Canada
更多查看译文
关键词
Autoregressive central variance subspace,autoregressive conditional heteroscedasticity,financial time series,Kernel method,modified information criterion
AI 理解论文
溯源树
样例
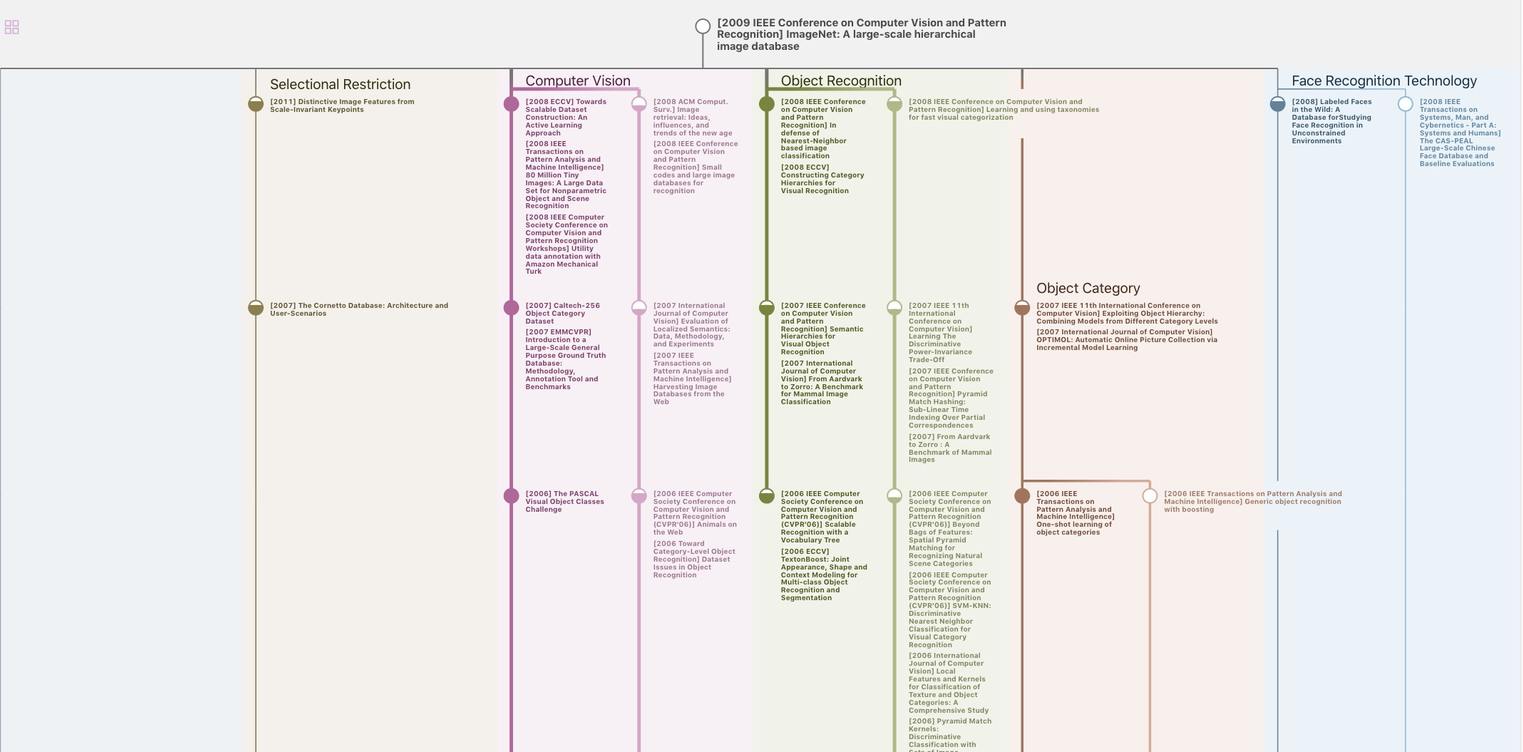
生成溯源树,研究论文发展脉络
Chat Paper
正在生成论文摘要