Language Independent Tokenization vs. Stemming in Automated Detection of Health Websites’ HONcode Conformity: An Evaluation
Procedia Computer Science(2015)
摘要
Authors evaluated supervised automatic classification algorithms for determination of health related web-page compliance with individual HONcode criteria of conduct (www.hon.ch/Conduct.html). The current study used varying length character n-gram vectors to represent healthcare web page documents – not the traditional approach of using word vectors. The training/testing collection comprised web page fragments that HONcode experts had cited as the basis for individual HONcode compliance during the manual certification process (described below). The authors compared automated classification performance of n-gram tokenization to the automated classification performance of document words and Porter-stemmed document words using a Naive Bayes classifier and DF (document frequency) dimensionality reduction metrics. The study attempted to determine whether the automated, language-independent approach might safely replace single word-based classification. Using 5-grams as document features, authors also compared the baseline DF reduction function to Chi-square and Z-score dimensionality reductions. While the Z-score approach statistically significantly improved precision for some HONcode compliance components, the Chi-square performance was unreliable, performing very well for some criteria and poorly for others. Overall study results indicate that n-gram tokenization provide a potentially viable alternative to document word stemming.
更多查看译文
关键词
Machine learning,N-gram,HONcode.
AI 理解论文
溯源树
样例
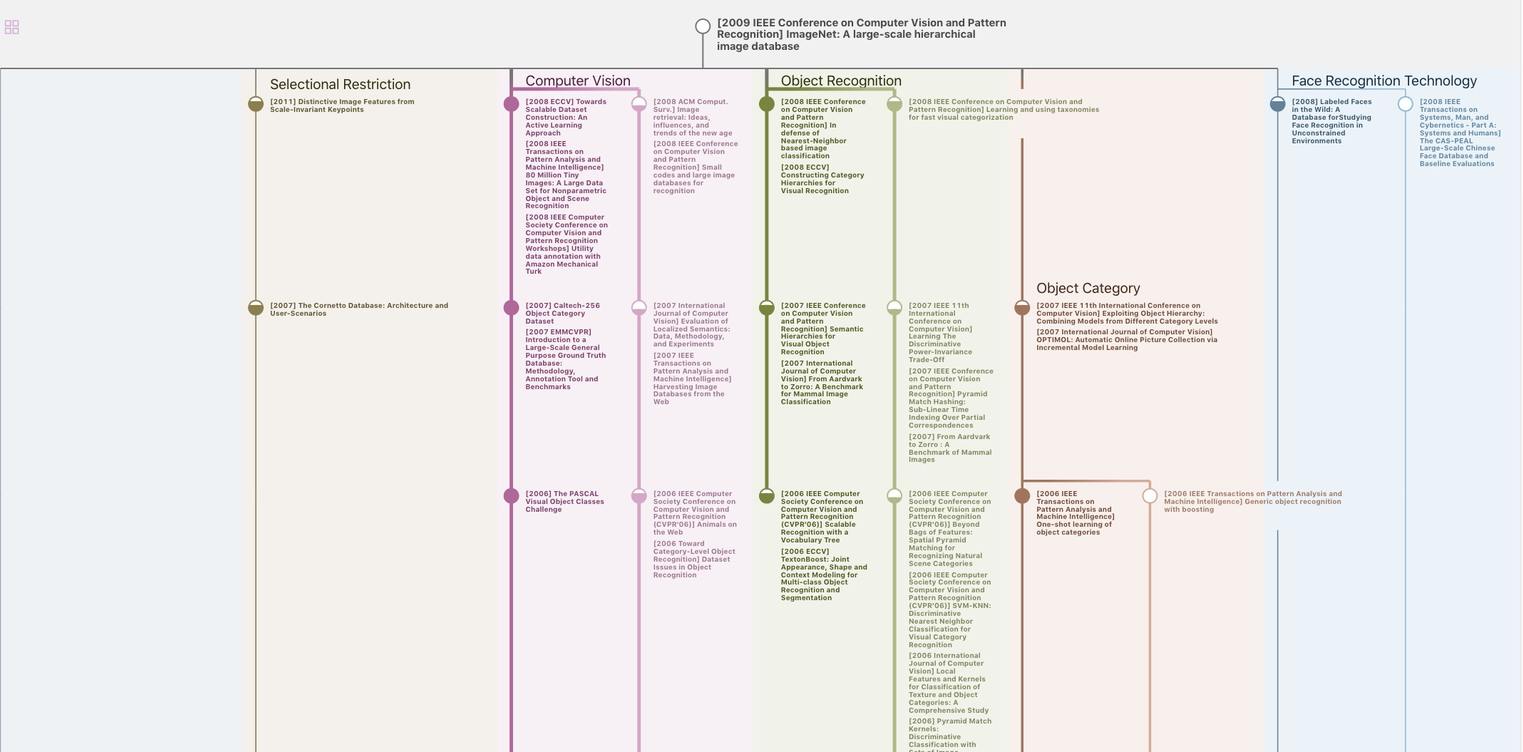
生成溯源树,研究论文发展脉络
Chat Paper
正在生成论文摘要