Application of All-Relevant Feature Selection for the Failure Analysis of Parameter-Induced Simulation Crashes in Climate Models
Feature Selection for Data and Pattern Recognition Studies in Computational Intelligence(2014)
摘要
Climate models are extremely complex pieces of software. They reflect the best knowledge on the physical components of the climate; nevertheless, they contain several parameters, which are too weakly constrained by observations, and can potentially lead to a simulation crashing. Recently a study by Lucas et al. (2013) has shown that machine learning methods can be used for predicting which combinations of parameters can lead to the simulation crashing and hence which processes described by these parameters need refined analyses. In the current study we reanalyse the data set used in this research using different methodology. We confirm the main conclusion of the original study concerning the suitability of machine learning for the prediction of crashes. We show that only three of the eight parameters indicated in the original study as relevant for prediction of the crash are indeed strongly relevant, three others are relevant but redundant and two are not relevant at all. We also show that the variance due to the split of data between training and validation sets has a large influence both on the accuracy of predictions and on the relative importance of variables; hence only a cross-validated approach can deliver a robust prediction of performance and relevance of variables.
更多查看译文
关键词
All-relevant feature selection,Strong and weak relevance,Feature importance,Boruta,Random forest
AI 理解论文
溯源树
样例
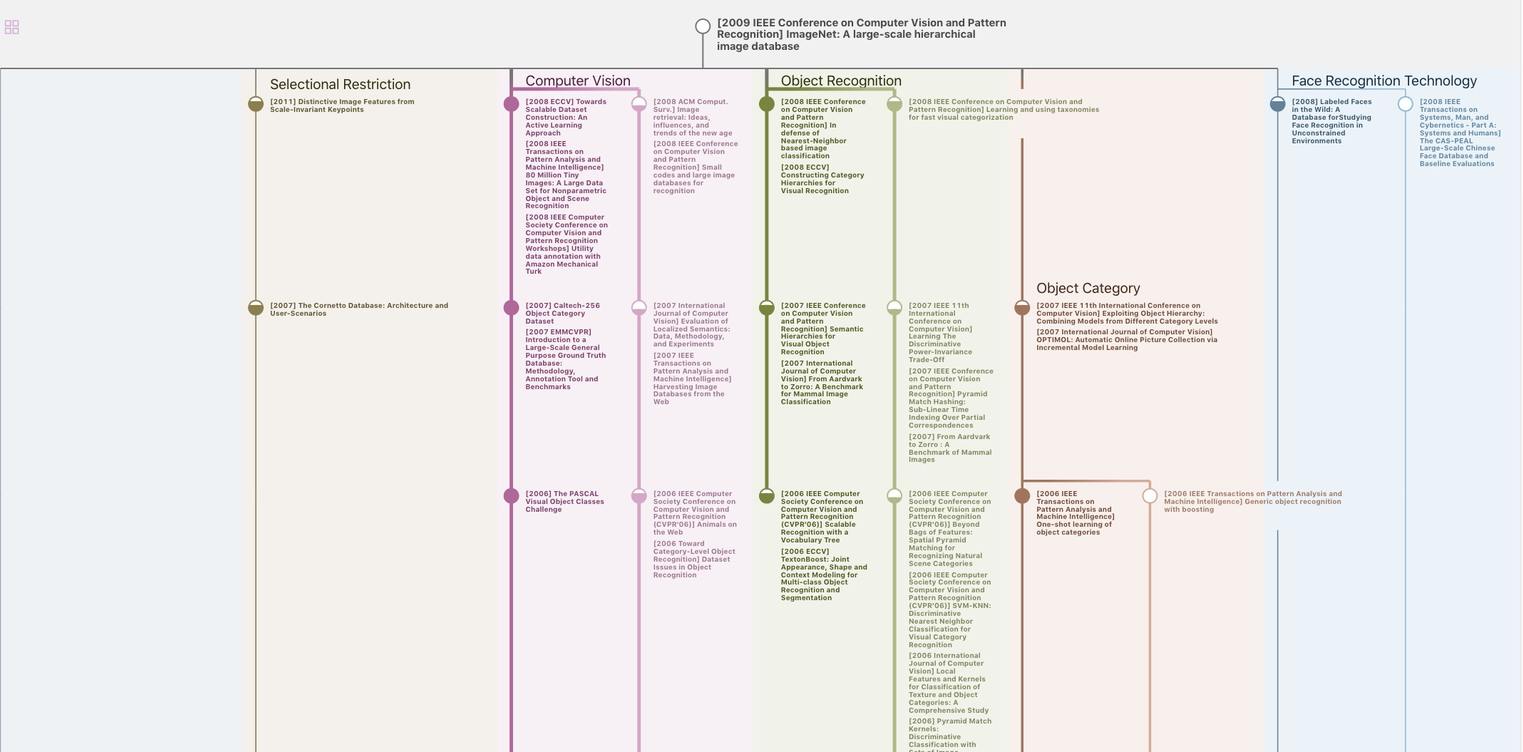
生成溯源树,研究论文发展脉络
Chat Paper
正在生成论文摘要