Generalized expectation–maximization approach to LPV process identification with randomly missing output data
Chemometrics and Intelligent Laboratory Systems(2015)
摘要
This paper considers parameter estimation for linear parameter varying (LPV) systems with randomly missing output data. The multi-model LPV model is adopted and the identification problem is formulated under the scheme of the generalized expectation–maximization (GEM) algorithm. In order to deal with the missing output data, the local models are firstly taken to have the finite impulse response (FIR) model structure. To alleviate potential overparameterization problem, a prior on FIR model coefficients is imposed and the GEM algorithm is modified to derive the maximum a posterior (MAP) estimates of the multi-mode LPV FIR model parameters. Since the FIR model is not suitable for general control applications, a multi-mode LPV output error (OE) model is then identified by applying the GEM algorithm to the same identification data set with parameters initialized based on the estimated FIR models. One simulation example and two experiments are presented to demonstrate the efficiency of the proposed method.
更多查看译文
关键词
LPV system identification,Missing output data,Generalized expectation–maximization algorithm,Maximum a posterior estimate,Experiment verification
AI 理解论文
溯源树
样例
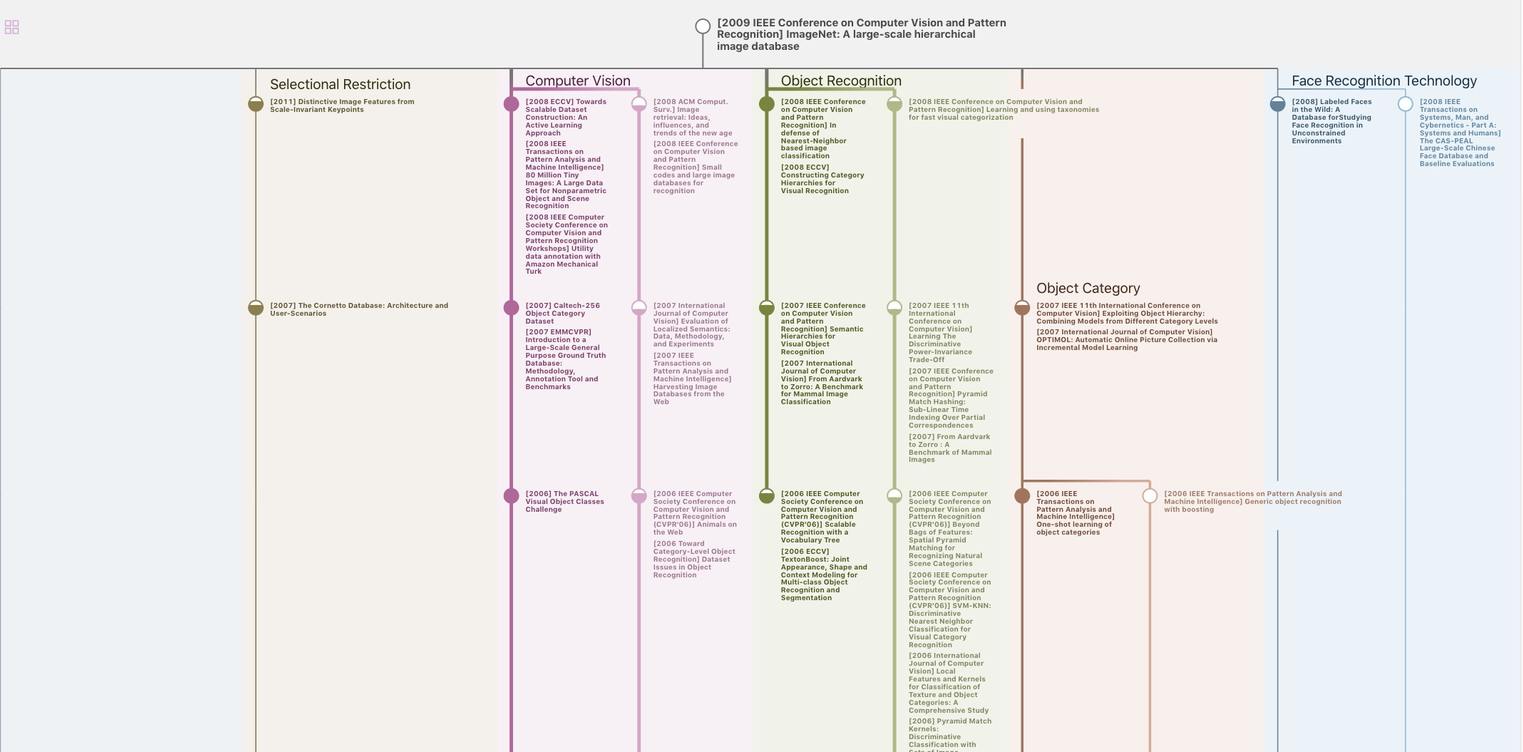
生成溯源树,研究论文发展脉络
Chat Paper
正在生成论文摘要